
An official website of the United States government
The .gov means it’s official. Federal government websites often end in .gov or .mil. Before sharing sensitive information, make sure you’re on a federal government site.
The site is secure. The https:// ensures that you are connecting to the official website and that any information you provide is encrypted and transmitted securely.
- Publications
- Account settings
Preview improvements coming to the PMC website in October 2024. Learn More or Try it out now .
- Advanced Search
- Journal List
- J Prev Med Public Health
- v.54(3); 2021 May
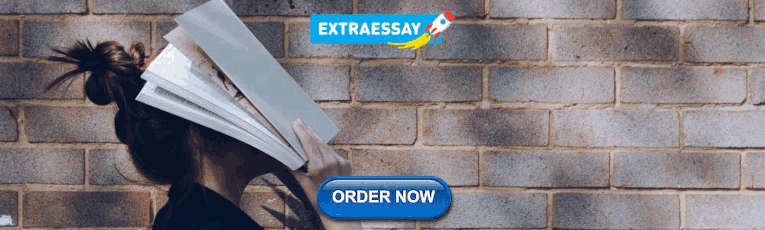
Introduction to Mediation Analysis and Examples of Its Application to Real-world Data
Sun jae jung.
1 Department of Preventive Medicine, Yonsei University College of Medicine, Seoul, Korea
2 Department of Public Health, Yonsei University Graduate School, Seoul, Korea
Traditional epidemiological assessments, which mainly focused on evaluating the statistical association between two major components-the exposure and outcome-have recently evolved to ascertain the in-between process, which can explain the underlying causal pathway. Mediation analysis has emerged as a compelling method to disentangle the complex nature of these pathways. The statistical method of mediation analysis has evolved from simple regression analysis to causal mediation analysis, and each amendment refined the underlying mathematical theory and required assumptions. This short guide will introduce the basic statistical framework and assumptions of both traditional and modern mediation analyses, providing examples conducted with real-world data.
INTRODUCTION
In the early days, traditional analytic epidemiological methods mainly focused on the statistical association between two major variables: the exposure (E) and the outcome (Y). However, methods have evolved to explore the “black box” between the E and the Y by investigating the mechanism underlying the association and various pathways. In the same context, the mechanism has also been visualized as being near the center of “Chinese boxes,” or a set of nested boxes. The “black box” is presumed to contain factors, both above and below the level of the individual—the factors above the individual may contain items such as interpersonal dynamics and socioeconomic status, including items related to ethnicity and politics, whereas the factors below the individual level comprise genes, proteins, cells, and organ systems [ 1 ].
Mediation analysis was developed to assess this “black box,” and psychologists and social scientists have utilized this framework particularly frequently. Mediation analysis can explore and evaluate biological or social mechanisms, thereby elucidating unknown biological pathways and/or aiding in policy-making [ 2 ]. However, because of advances in methodologies, including biostatistics, epidemiological research designs, and causal inference, traditional mediation analysis has evolved and been applied in various fields. In particular, the concept of mediation analysis has been especially appealing in social sciences and psychology. There are several overviews of these topics [ 3 - 6 ], and this study is a guide to the full literature.
TRADITIONAL REGRESSION-BASED MEDIATION ANALYSIS
Mediation was initially hypothesized as a variable in the middle of a causal chain. Previously, most of the epidemiological reports focused on evaluating the simple association between E and Y as in Figure 1A . However, as in Figure 1B , it is shown that an E affects a mediator (M), which in turn affects an Y. The M fully mediates the effect from the E to the Y. However, situations were identified where the M does not fully mediate the effect of E on the Y, which led to the concept of partial mediation, as depicted in Figure 1C . As shown in Figure 1C , the effect of an E can be exerted directly on an Y (direct effect, path c’) or take a detour via a M (indirect effect, paths a and b). Initially, the criteria to be regarded as a M were that E should have a statistically significant association with M, and that M should also have a statistically significant association with Y. The initial criteria also included the condition that the mediation analysis could be performed only if there was a statistically significant association between E and Y; this significant relationship between E and Y should be no longer significant after controlling for the previous paths from E to M and M to Y. However, the latter two conditions were further criticized due to the existence of inconsistent and partial mediation, and were therefore omitted from the essential conditions needed for mediation analysis.

A conceptual diagram of mediation analysis (A) traditional epidemiological assessment, (B) full mediation, and (C) partial mediation.
In contrast to a moderator or confounder, a M is interpreted as involving a causal pathway between E and Y. A detailed definition of a M is provided in the work of Robins and Greenland [ 7 ]. The seminal work on this concept of a M or intervening variable was based on Judd and Kenny [ 8 , 9 ] and Baron and Kenny [ 10 ]’s article utilizing the regression method.
In Judd and Kenny[ 8 , 9 ]’s difference of coefficients approach, mediation analysis can be conceptualized as utilizing two regressions, as follows. First, we run a simple regression analysis with E on Y without M to estimate path c’.
Second, we carry out a multivariable regression with E and M to predict Y.
In this case, as the coefficient B reflects the total effect (TE), the direct effect from the E to Y c’ shown in Figure 1C , corresponds to B 1 in equation 2 . The difference method calculates the indirect effect by subtracting the direct effect (c’) from the TE, as follows:
This is a simple and widely used approach to screen for the possible presence of a M. However, the logistic regression method has been criticized for lacking a causal interpretation. The difference method has been used to check for mediation, but non-significant findings using this method do not exclude the chance of possible mediation [ 11 ].
The other approach is the product method, which was introduced by Sobel and used by Baron and Kenny [ 10 ]. In this method, again, a multivariable regression is conducted with E and M to predict Y.
However, the next step is to regress M on X and can be written as
In equation 3 , B reflects path a in Figure 1C , and B 2 in equation 2 reflects b in Figure 1C . The coefficient of the indirect effect, B indirect , is calculated by multiplying the 2 coefficients, B 2 and B.
Generally, when there is no interaction between an E and a M, these two methods coincide, except for logistic regression. In particular, for rare Ys (approximately under 10%) with no confounding factors, these 2 estimates will, from a practical standpoint, reflect the natural indirect effect (NIE), which will be discussed in the causal mediation section. The difference method is beneficial because there is no restriction of the M distribution; it can be continuous or categorical (including binary). In contrast, the product method requires a linear model to be applied for the M [ 11 ]. In situations with common Ys, especially when they are binary, a log-linear regression model instead of logistic regression is recommended [ 12 ].
To calculate the confidence interval (CI) of the indirect effect, 2 approaches have been suggested. The first approach utilizes the Sobel test, which is based on the product of 2 normally distributed values of coefficients. In this case, an assumption should be made about the shape of the sampling distribution of the indirect effect. The second approach uses resampling methods, such as bootstrap testing, which does not require a prior assumption of the sampling distribution. Usually, the bootstrap method involves resampling at least 750 times, for which reason the default resampling setting is 1000 times in many macros (e.g., R and the PROCESS macro in SAS [ 13 , 14 ]).
EXAMPLE OF REGRESSION-BASED MEDIATION ANALYSIS
Kim et al. [ 15 ] conducted a study to estimate the mediating effect of lifestyle factors on the association between social networks and metabolic syndrome, utilizing the baseline data of the community-based Cardiovascular and Metabolic Diseases Etiology Research Center cohort. In total, 10 103 participants were recruited from 2013 to 2018, and their egocentric social network properties were measured using a social network card that was previously applied and standardized [ 16 ]. From the raw data of the social network cards, the authors extracted and calculated the size of the social network and the closeness of the social network, which were used as quantitative E variables. Measurements of blood pressure, the lipid profile, fasting glucose, and waist circumference were made in the initial cohort, and metabolic syndrome was defined based on the National Cholesterol Education Program Adult Treatment Panel III criteria as the presence of 3 or more criteria.
As potential Ms, the authors tested 4 domains: physical inactiveness (3 categories: vigorous activities, moderate activities, and walking), alcohol consumption (binary variable: current drinker vs. non-drinker), cigarette smoking (binary variable: current smoker vs. non-smoker), and depressive symptoms (continuous variable: range 0-63 by Beck Depressive Inventory-II score).
After conducting the multivariable logistic regression for the E (social network properties, continuous variables) and Y (metabolic syndrome, yes/no), mediation analysis was performed with the ‘mediation’ package developed by Imai et al. [ 17 ] in the R software [ 18 ]. The analysis was conducted in 3 steps: (1) producing a M model, (2) producing an Y model, and (3) conducting a mediation analysis and sensitivity analysis. In the M model, social network properties and other covariates were regressed to explain lifestyle factors. The metabolic syndrome variable was then regressed on social network properties, lifestyle factors, and other covariates. These two models were grouped with the “mediate” function, which was run to estimate the direct effect, indirect effect, and their 95% CI by a quasi-Bayesian Monte Carlo method, including 5000 simulations per estimate set.
As there were 4 potential Ms, the authors applied each M and tested the indirect effect. They found that only physical activity significantly mediated the relationship between social network size and metabolic syndrome in both genders (men: effect size [ES]=5.2×10 -3 , p=0.024; women: ES=3.1×10 -3 , p <0.001) ( Figure 2A )

Brief conceptual diagrams of examples in this review. (A) Brief conceptual diagram by Kim et al. 2020 [ 15 ]. (B) Brief conceptual diagram by Lee et al. 2021 [ 23 ]. NDE, natural direct effect; OR, odds ratio; CI, confidence interval; NIE, natural indirect effect; TE, total effect. * p<0.05.
INTRODUCING CAUSAL MEDIATION ANALYSIS
After the rise of the counterfactual framework for modern causal inference, the traditional approach in mediation analyses was expanded and re-developed to solve the previous limitations regarding non-linearities and interactions, focusing on the decomposition of direct and indirect effects [ 19 , 20 ]. Among the major issues raised, assumptions related to confounding factors and the interaction between the E and the M were reflected and re-developed in causal mediation analysis [ 7 , 21 ]. In the counterfactual concept, an individual is hypothetically compared under an E and in the absence of the E in identical situations, including time and surrounding conditions. If the potential Ys are different based on this comparison, the E is regarded as causal for the Y [ 22 ].
In causal mediation analysis, 3 terms regarding the previous indirect and direct effects are suggested. The natural direct effect (NDE) and NIE can be interpreted in traditional mediation analysis. There would be a difference between the counterfactual Ys if an individual was exposed to 2 different counterfactual situations, where the M value would be random at the reference value of the E. In contrast, the controlled direct effect (CDE) is different regarding the mediation value used in the calculation since the M is set to a certain fixed level. If there is no interaction between E and M, then the CDE usually coincides with the NDE [ 4 ].
For example, an analysis using the NDE would ask “how much would the Y (e.g., suicide rate) change if the E was set at e=1 versus e=0 (e.g., exercise program), but for each participant, the M (e.g., the Patient Health Questionnaire [PHQ]-9) was kept at the level it would have been in the absence of the E (i.e., the mean depressive symptom score of the group that did not participate in the exercise program)?” An analysis using the CDE would ask, “how much would the Y (e.g., suicide rate) would change on average if the M was controlled at a certain level (e.g., PHQ-9=5) uniformly in the population?” Likewise, an analysis using the NIE would answer the question, “how much would the Y (e.g., suicide rate) would change on average if the E was controlled at the level it would be with the E present (e.g., with everyone participating in the exercise program), but with the M (e.g., PHQ-9 change) changed from the level it would be with the E at the reference level (e.g., the usual rate of people in the exercise program) to the level it would be if the E is present?” In sum, the TE would correspond to the question, “how much would the Y (e.g., suicide rate) change overall with a change in the E from the reference value to the present?” This implies that the sum of the NDE and NIE equals the TE. Generally, the CDE has received more interest for policy evaluations, whereas the NIE and NDE have been used to elucidate the actions of various biological mechanisms.
Similar to traditional mediation analysis, causal mediation analysis presumes the following temporal ordering: the E must precede the M measurement, and the Y measurement is performed after the M measurement. In addition, to interpret the mediation causally, 4 other assumptions related to confounding should be satisfied. First, all the known confounders should be controlled, and there should be no unmeasured confounding of the E-Y relationship (C 1 ) ( Figure 3 ). If the E is randomized (e.g., in randomized clinical trials), this assumption will be met. Second, all the known confounders should be controlled, and there should be no unmeasured confounding of the M-Y relationship (C 2 ). In this case, it would not be enough to randomize only the E. Third, there should be no unmeasured confounding of the E-M relationship, or all the known confounders should be controlled, which would be covered by E randomization. Lastly, there should be no confounding related to the M-Y relationship affected by the E, which means there is no arrow from E to C 2 in Figure 3 . As mentioned previously, randomizing the E (or treatment) is not enough to completely solve the confounding issue; randomizing E (which gives a probable even distribution of C 1 ) would not be sufficient to control the confounding, which can also occur between the M and Y, represented as C 2 . In this case, conducting several sensitivity analyses would help, including situations with unmeasured confounding. Most importantly, it is strongly recommended to construct a directed acyclic graph depicting the central hypothesis before conducting a causal mediation analysis.

Confounding assumptions in causal mediation analysis.
In 2013, SAS (SAS Institute Inc., Cary, NC, USA) macros were used to perform a causal mediation analysis by Valeri and VanderWeele [ 2 ]. This initial macro dealt with binary forms of E, binary forms of Ms, and continuous Y variables. Additionally, in this macro, count variables could be applied as the Ys. A full description of this macro has been published elsewhere [ 4 ].
EXAMPLE OF CAUSAL MEDIATION ANALYSIS
Lee et al. [ 23 ] performed a longitudinal analysis using data from 3347 participants aged 40-64 years in the Korean Genome and Epidemiology Study, who were followed up for 16 years. As the E, socioeconomic status, including educational attainment and monthly household income, were queried at the index year and categorized into 2 groups. As the Y, sleep quality was queried with the Pittsburgh Sleep Quality Index at 5 time points (years 2, 6, 8, 10, and 12). As a M, depressive symptoms were measured using the Beck Depression Inventory at year 4. Sleep quality patterns were the Y variable. Using latent class growth modeling with SAS Proc traj syntax, a group-based modeling approach was performed, and 5 subgroups were identified according to the pattern of sleep quality (“normal-stable,” “moderate-stable,” “poor-stable,” “developing to poor,” and “severely poor-stable”).
Using SAS Proc causalmed syntax, the potential mediation of depressive symptoms on the association between socioeconomic factors and longitudinal sleep quality patterns was tested. Based on the maximum likelihood method, this SAS procedure estimates the effect of causal mediation and CIs from 1000 bootstrap replications [ 24 ]. Since this procedure permits a binary Y only, the original 5 sleep quality patterns were grouped into 2 categories, including a reference category (e.g., normal-stable vs. moderate-stable, or normal-stable vs. severely poor-stable). Percentages were calculated to explain the mediation and interaction effects, and the percentage of the TE after controlling the level of the M was also calculated [ 24 ].
Overall, the associations between socioeconomic status variables and sleep patterns were not significant after full adjustment. However, depressive symptoms tended to fully mediate the associations between education/income variables and sleep quality patterns (e.g., for E=lower education vs. higher education, Y=developing to poor vs. normal-stable, TE: odds ratio [OR], 1.55; 95% CI, 0.64 to 6.03; NDE: OR, 1.38; 95% CI, 0.58 to 5.09); NIE: OR, 1.12; 95% CI, 1.04 to 1.24) ( Figure 2B ).
This paper reviewed the basic concepts of traditional mediation and causal mediation analysis with counterfactual approaches and provided examples in real-world settings.
One issue to be aware of is that a statistically significant association regarding M in the mediation analysis (e.g., a statistically significant indirect effect) does not always confirm that M is an actual M. Using different causal models does not make it possible for researchers to prove a unique M unless it is theoretically plausible. Furthermore, mediation analysis itself cannot provide that an intervening variable is a true M by probabilistic inference, since we cannot verify the likelihood distribution of all other potential Ms and alternative causal models [ 25 ]. Therefore, it is essential to understand that researchers should interpret mediation analysis within the logic of theoretical inferences.
Another issue lies in the measurement error for the M. According to a study conducted by le Cessie et al. [ 26 ], under the classical condition of a normally distributed M with non-differential misclassification, the estimated mediated association tended toward the null. If the direct and indirect effects were the same, the estimates tended away from the null. However, when the M was multinomial, this pattern did not always exist. Correction methods, such as using a weighting coefficient and attenuating the regression coefficient B2 in equation 2 , were also suggested by le Cessie et al. [ 26 ].
Theoretical concepts and statistical application methods regarding mediation analysis are rapidly developing. As a result, further discussions on filling the gap between theoretical assumptions and practical analytical issues are required. It has been suggested that conceptualization and formalism may be obstacles for epidemiologists to apply these methods to actual analysis [ 27 ] and future directions should involve the development of more unified and simple methods that could be utilized by a broader base of users. However, because of its usefulness in elucidating complex mechanisms in population data, the rapid adoption of mediation analysis in future epidemiological studies is expected.
Ethics Statement
As this review does not involve newly collected human data, institutional review board approval is not needed.
Acknowledgments
CONFLICT OF INTEREST
The author has no conflicts of interest associated with the material presented in this paper.
This research was supported by the Basic Science Research Program through the National Research Foundation of Korea (NRF) funded by the Ministry of Science and ICT (2020R1C1C1003502) and a faculty research grant of Yonsei University College of Medicine for 2019 (6-2019-0114).
AUTHOR CONTRIBUTIONS
All work was done by SJJ.
Click through the PLOS taxonomy to find articles in your field.
For more information about PLOS Subject Areas, click here .
Loading metrics
Open Access
Peer-reviewed
Research Article
Anxiety, Affect, Self-Esteem, and Stress: Mediation and Moderation Effects on Depression
Affiliations Department of Psychology, University of Gothenburg, Gothenburg, Sweden, Network for Empowerment and Well-Being, University of Gothenburg, Gothenburg, Sweden
Affiliation Network for Empowerment and Well-Being, University of Gothenburg, Gothenburg, Sweden
Affiliations Department of Psychology, University of Gothenburg, Gothenburg, Sweden, Network for Empowerment and Well-Being, University of Gothenburg, Gothenburg, Sweden, Department of Psychology, Education and Sport Science, Linneaus University, Kalmar, Sweden
* E-mail: [email protected]
Affiliations Network for Empowerment and Well-Being, University of Gothenburg, Gothenburg, Sweden, Center for Ethics, Law, and Mental Health (CELAM), University of Gothenburg, Gothenburg, Sweden, Institute of Neuroscience and Physiology, The Sahlgrenska Academy, University of Gothenburg, Gothenburg, Sweden
- Ali Al Nima,
- Patricia Rosenberg,
- Trevor Archer,
- Danilo Garcia
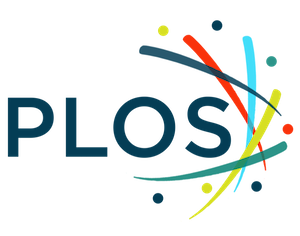
- Published: September 9, 2013
- https://doi.org/10.1371/journal.pone.0073265
- Reader Comments
23 Sep 2013: Nima AA, Rosenberg P, Archer T, Garcia D (2013) Correction: Anxiety, Affect, Self-Esteem, and Stress: Mediation and Moderation Effects on Depression. PLOS ONE 8(9): 10.1371/annotation/49e2c5c8-e8a8-4011-80fc-02c6724b2acc. https://doi.org/10.1371/annotation/49e2c5c8-e8a8-4011-80fc-02c6724b2acc View correction
Mediation analysis investigates whether a variable (i.e., mediator) changes in regard to an independent variable, in turn, affecting a dependent variable. Moderation analysis, on the other hand, investigates whether the statistical interaction between independent variables predict a dependent variable. Although this difference between these two types of analysis is explicit in current literature, there is still confusion with regard to the mediating and moderating effects of different variables on depression. The purpose of this study was to assess the mediating and moderating effects of anxiety, stress, positive affect, and negative affect on depression.
Two hundred and two university students (males = 93, females = 113) completed questionnaires assessing anxiety, stress, self-esteem, positive and negative affect, and depression. Mediation and moderation analyses were conducted using techniques based on standard multiple regression and hierarchical regression analyses.
Main Findings
The results indicated that (i) anxiety partially mediated the effects of both stress and self-esteem upon depression, (ii) that stress partially mediated the effects of anxiety and positive affect upon depression, (iii) that stress completely mediated the effects of self-esteem on depression, and (iv) that there was a significant interaction between stress and negative affect, and between positive affect and negative affect upon depression.
The study highlights different research questions that can be investigated depending on whether researchers decide to use the same variables as mediators and/or moderators.
Citation: Nima AA, Rosenberg P, Archer T, Garcia D (2013) Anxiety, Affect, Self-Esteem, and Stress: Mediation and Moderation Effects on Depression. PLoS ONE 8(9): e73265. https://doi.org/10.1371/journal.pone.0073265
Editor: Ben J. Harrison, The University of Melbourne, Australia
Received: February 21, 2013; Accepted: July 22, 2013; Published: September 9, 2013
Copyright: © 2013 Nima et al. This is an open-access article distributed under the terms of the Creative Commons Attribution License, which permits unrestricted use, distribution, and reproduction in any medium, provided the original author and source are credited.
Funding: The authors have no support or funding to report.
Competing interests: The authors have declared that no competing interests exist.
Introduction
Mediation refers to the covariance relationships among three variables: an independent variable (1), an assumed mediating variable (2), and a dependent variable (3). Mediation analysis investigates whether the mediating variable accounts for a significant amount of the shared variance between the independent and the dependent variables–the mediator changes in regard to the independent variable, in turn, affecting the dependent one [1] , [2] . On the other hand, moderation refers to the examination of the statistical interaction between independent variables in predicting a dependent variable [1] , [3] . In contrast to the mediator, the moderator is not expected to be correlated with both the independent and the dependent variable–Baron and Kenny [1] actually recommend that it is best if the moderator is not correlated with the independent variable and if the moderator is relatively stable, like a demographic variable (e.g., gender, socio-economic status) or a personality trait (e.g., affectivity).
Although both types of analysis lead to different conclusions [3] and the distinction between statistical procedures is part of the current literature [2] , there is still confusion about the use of moderation and mediation analyses using data pertaining to the prediction of depression. There are, for example, contradictions among studies that investigate mediating and moderating effects of anxiety, stress, self-esteem, and affect on depression. Depression, anxiety and stress are suggested to influence individuals' social relations and activities, work, and studies, as well as compromising decision-making and coping strategies [4] , [5] , [6] . Successfully coping with anxiety, depressiveness, and stressful situations may contribute to high levels of self-esteem and self-confidence, in addition increasing well-being, and psychological and physical health [6] . Thus, it is important to disentangle how these variables are related to each other. However, while some researchers perform mediation analysis with some of the variables mentioned here, other researchers conduct moderation analysis with the same variables. Seldom are both moderation and mediation performed on the same dataset. Before disentangling mediation and moderation effects on depression in the current literature, we briefly present the methodology behind the analysis performed in this study.
Mediation and moderation
Baron and Kenny [1] postulated several criteria for the analysis of a mediating effect: a significant correlation between the independent and the dependent variable, the independent variable must be significantly associated with the mediator, the mediator predicts the dependent variable even when the independent variable is controlled for, and the correlation between the independent and the dependent variable must be eliminated or reduced when the mediator is controlled for. All the criteria is then tested using the Sobel test which shows whether indirect effects are significant or not [1] , [7] . A complete mediating effect occurs when the correlation between the independent and the dependent variable are eliminated when the mediator is controlled for [8] . Analyses of mediation can, for example, help researchers to move beyond answering if high levels of stress lead to high levels of depression. With mediation analysis researchers might instead answer how stress is related to depression.
In contrast to mediation, moderation investigates the unique conditions under which two variables are related [3] . The third variable here, the moderator, is not an intermediate variable in the causal sequence from the independent to the dependent variable. For the analysis of moderation effects, the relation between the independent and dependent variable must be different at different levels of the moderator [3] . Moderators are included in the statistical analysis as an interaction term [1] . When analyzing moderating effects the variables should first be centered (i.e., calculating the mean to become 0 and the standard deviation to become 1) in order to avoid problems with multi-colinearity [8] . Moderating effects can be calculated using multiple hierarchical linear regressions whereby main effects are presented in the first step and interactions in the second step [1] . Analysis of moderation, for example, helps researchers to answer when or under which conditions stress is related to depression.
Mediation and moderation effects on depression
Cognitive vulnerability models suggest that maladaptive self-schema mirroring helplessness and low self-esteem explain the development and maintenance of depression (for a review see [9] ). These cognitive vulnerability factors become activated by negative life events or negative moods [10] and are suggested to interact with environmental stressors to increase risk for depression and other emotional disorders [11] , [10] . In this line of thinking, the experience of stress, low self-esteem, and negative emotions can cause depression, but also be used to explain how (i.e., mediation) and under which conditions (i.e., moderation) specific variables influence depression.
Using mediational analyses to investigate how cognitive therapy intervations reduced depression, researchers have showed that the intervention reduced anxiety, which in turn was responsible for 91% of the reduction in depression [12] . In the same study, reductions in depression, by the intervention, accounted only for 6% of the reduction in anxiety. Thus, anxiety seems to affect depression more than depression affects anxiety and, together with stress, is both a cause of and a powerful mediator influencing depression (See also [13] ). Indeed, there are positive relationships between depression, anxiety and stress in different cultures [14] . Moreover, while some studies show that stress (independent variable) increases anxiety (mediator), which in turn increased depression (dependent variable) [14] , other studies show that stress (moderator) interacts with maladaptive self-schemata (dependent variable) to increase depression (independent variable) [15] , [16] .
The present study
In order to illustrate how mediation and moderation can be used to address different research questions we first focus our attention to anxiety and stress as mediators of different variables that earlier have been shown to be related to depression. Secondly, we use all variables to find which of these variables moderate the effects on depression.
The specific aims of the present study were:
- To investigate if anxiety mediated the effect of stress, self-esteem, and affect on depression.
- To investigate if stress mediated the effects of anxiety, self-esteem, and affect on depression.
- To examine moderation effects between anxiety, stress, self-esteem, and affect on depression.
Ethics statement
This research protocol was approved by the Ethics Committee of the University of Gothenburg and written informed consent was obtained from all the study participants.
Participants
The present study was based upon a sample of 206 participants (males = 93, females = 113). All the participants were first year students in different disciplines at two universities in South Sweden. The mean age for the male students was 25.93 years ( SD = 6.66), and 25.30 years ( SD = 5.83) for the female students.
In total, 206 questionnaires were distributed to the students. Together 202 questionnaires were responded to leaving a total dropout of 1.94%. This dropout concerned three sections that the participants chose not to respond to at all, and one section that was completed incorrectly. None of these four questionnaires was included in the analyses.
Instruments
Hospital anxiety and depression scale [17] ..
The Swedish translation of this instrument [18] was used to measure anxiety and depression. The instrument consists of 14 statements (7 of which measure depression and 7 measure anxiety) to which participants are asked to respond grade of agreement on a Likert scale (0 to 3). The utility, reliability and validity of the instrument has been shown in multiple studies (e.g., [19] ).
Perceived Stress Scale [20] .
The Swedish version [21] of this instrument was used to measures individuals' experience of stress. The instrument consist of 14 statements to which participants rate on a Likert scale (0 = never , 4 = very often ). High values indicate that the individual expresses a high degree of stress.
Rosenberg's Self-Esteem Scale [22] .
The Rosenberg's Self-Esteem Scale (Swedish version by Lindwall [23] ) consists of 10 statements focusing on general feelings toward the self. Participants are asked to report grade of agreement in a four-point Likert scale (1 = agree not at all, 4 = agree completely ). This is the most widely used instrument for estimation of self-esteem with high levels of reliability and validity (e.g., [24] , [25] ).
Positive Affect and Negative Affect Schedule [26] .
This is a widely applied instrument for measuring individuals' self-reported mood and feelings. The Swedish version has been used among participants of different ages and occupations (e.g., [27] , [28] , [29] ). The instrument consists of 20 adjectives, 10 positive affect (e.g., proud, strong) and 10 negative affect (e.g., afraid, irritable). The adjectives are rated on a five-point Likert scale (1 = not at all , 5 = very much ). The instrument is a reliable, valid, and effective self-report instrument for estimating these two important and independent aspects of mood [26] .
Questionnaires were distributed to the participants on several different locations within the university, including the library and lecture halls. Participants were asked to complete the questionnaire after being informed about the purpose and duration (10–15 minutes) of the study. Participants were also ensured complete anonymity and informed that they could end their participation whenever they liked.
Correlational analysis
Depression showed positive, significant relationships with anxiety, stress and negative affect. Table 1 presents the correlation coefficients, mean values and standard deviations ( sd ), as well as Cronbach ' s α for all the variables in the study.
- PPT PowerPoint slide
- PNG larger image
- TIFF original image
https://doi.org/10.1371/journal.pone.0073265.t001
Mediation analysis
Regression analyses were performed in order to investigate if anxiety mediated the effect of stress, self-esteem, and affect on depression (aim 1). The first regression showed that stress ( B = .03, 95% CI [.02,.05], β = .36, t = 4.32, p <.001), self-esteem ( B = −.03, 95% CI [−.05, −.01], β = −.24, t = −3.20, p <.001), and positive affect ( B = −.02, 95% CI [−.05, −.01], β = −.19, t = −2.93, p = .004) had each an unique effect on depression. Surprisingly, negative affect did not predict depression ( p = 0.77) and was therefore removed from the mediation model, thus not included in further analysis.
The second regression tested whether stress, self-esteem and positive affect uniquely predicted the mediator (i.e., anxiety). Stress was found to be positively associated ( B = .21, 95% CI [.15,.27], β = .47, t = 7.35, p <.001), whereas self-esteem was negatively associated ( B = −.29, 95% CI [−.38, −.21], β = −.42, t = −6.48, p <.001) to anxiety. Positive affect, however, was not associated to anxiety ( p = .50) and was therefore removed from further analysis.
A hierarchical regression analysis using depression as the outcome variable was performed using stress and self-esteem as predictors in the first step, and anxiety as predictor in the second step. This analysis allows the examination of whether stress and self-esteem predict depression and if this relation is weaken in the presence of anxiety as the mediator. The result indicated that, in the first step, both stress ( B = .04, 95% CI [.03,.05], β = .45, t = 6.43, p <.001) and self-esteem ( B = .04, 95% CI [.03,.05], β = .45, t = 6.43, p <.001) predicted depression. When anxiety (i.e., the mediator) was controlled for predictability was reduced somewhat but was still significant for stress ( B = .03, 95% CI [.02,.04], β = .33, t = 4.29, p <.001) and for self-esteem ( B = −.03, 95% CI [−.05, −.01], β = −.20, t = −2.62, p = .009). Anxiety, as a mediator, predicted depression even when both stress and self-esteem were controlled for ( B = .05, 95% CI [.02,.08], β = .26, t = 3.17, p = .002). Anxiety improved the prediction of depression over-and-above the independent variables (i.e., stress and self-esteem) (Δ R 2 = .03, F (1, 198) = 10.06, p = .002). See Table 2 for the details.
https://doi.org/10.1371/journal.pone.0073265.t002
A Sobel test was conducted to test the mediating criteria and to assess whether indirect effects were significant or not. The result showed that the complete pathway from stress (independent variable) to anxiety (mediator) to depression (dependent variable) was significant ( z = 2.89, p = .003). The complete pathway from self-esteem (independent variable) to anxiety (mediator) to depression (dependent variable) was also significant ( z = 2.82, p = .004). Thus, indicating that anxiety partially mediates the effects of both stress and self-esteem on depression. This result may indicate also that both stress and self-esteem contribute directly to explain the variation in depression and indirectly via experienced level of anxiety (see Figure 1 ).
Changes in Beta weights when the mediator is present are highlighted in red.
https://doi.org/10.1371/journal.pone.0073265.g001
For the second aim, regression analyses were performed in order to test if stress mediated the effect of anxiety, self-esteem, and affect on depression. The first regression showed that anxiety ( B = .07, 95% CI [.04,.10], β = .37, t = 4.57, p <.001), self-esteem ( B = −.02, 95% CI [−.05, −.01], β = −.18, t = −2.23, p = .03), and positive affect ( B = −.03, 95% CI [−.04, −.02], β = −.27, t = −4.35, p <.001) predicted depression independently of each other. Negative affect did not predict depression ( p = 0.74) and was therefore removed from further analysis.
The second regression investigated if anxiety, self-esteem and positive affect uniquely predicted the mediator (i.e., stress). Stress was positively associated to anxiety ( B = 1.01, 95% CI [.75, 1.30], β = .46, t = 7.35, p <.001), negatively associated to self-esteem ( B = −.30, 95% CI [−.50, −.01], β = −.19, t = −2.90, p = .004), and a negatively associated to positive affect ( B = −.33, 95% CI [−.46, −.20], β = −.27, t = −5.02, p <.001).
A hierarchical regression analysis using depression as the outcome and anxiety, self-esteem, and positive affect as the predictors in the first step, and stress as the predictor in the second step, allowed the examination of whether anxiety, self-esteem and positive affect predicted depression and if this association would weaken when stress (i.e., the mediator) was present. In the first step of the regression anxiety ( B = .07, 95% CI [.05,.10], β = .38, t = 5.31, p = .02), self-esteem ( B = −.03, 95% CI [−.05, −.01], β = −.18, t = −2.41, p = .02), and positive affect ( B = −.03, 95% CI [−.04, −.02], β = −.27, t = −4.36, p <.001) significantly explained depression. When stress (i.e., the mediator) was controlled for, predictability was reduced somewhat but was still significant for anxiety ( B = .05, 95% CI [.02,.08], β = .05, t = 4.29, p <.001) and for positive affect ( B = −.02, 95% CI [−.04, −.01], β = −.20, t = −3.16, p = .002), whereas self-esteem did not reach significance ( p < = .08). In the second step, the mediator (i.e., stress) predicted depression even when anxiety, self-esteem, and positive affect were controlled for ( B = .02, 95% CI [.08,.04], β = .25, t = 3.07, p = .002). Stress improved the prediction of depression over-and-above the independent variables (i.e., anxiety, self-esteem and positive affect) (Δ R 2 = .02, F (1, 197) = 9.40, p = .002). See Table 3 for the details.
https://doi.org/10.1371/journal.pone.0073265.t003
Furthermore, the Sobel test indicated that the complete pathways from the independent variables (anxiety: z = 2.81, p = .004; self-esteem: z = 2.05, p = .04; positive affect: z = 2.58, p <.01) to the mediator (i.e., stress), to the outcome (i.e., depression) were significant. These specific results might be explained on the basis that stress partially mediated the effects of both anxiety and positive affect on depression while stress completely mediated the effects of self-esteem on depression. In other words, anxiety and positive affect contributed directly to explain the variation in depression and indirectly via the experienced level of stress. Self-esteem contributed only indirectly via the experienced level of stress to explain the variation in depression. In other words, stress effects on depression originate from “its own power” and explained more of the variation in depression than self-esteem (see Figure 2 ).
https://doi.org/10.1371/journal.pone.0073265.g002
Moderation analysis
Multiple linear regression analyses were used in order to examine moderation effects between anxiety, stress, self-esteem and affect on depression. The analysis indicated that about 52% of the variation in the dependent variable (i.e., depression) could be explained by the main effects and the interaction effects ( R 2 = .55, adjusted R 2 = .51, F (55, 186) = 14.87, p <.001). When the variables (dependent and independent) were standardized, both the standardized regression coefficients beta (β) and the unstandardized regression coefficients beta (B) became the same value with regard to the main effects. Three of the main effects were significant and contributed uniquely to high levels of depression: anxiety ( B = .26, t = 3.12, p = .002), stress ( B = .25, t = 2.86, p = .005), and self-esteem ( B = −.17, t = −2.17, p = .03). The main effect of positive affect was also significant and contributed to low levels of depression ( B = −.16, t = −2.027, p = .02) (see Figure 3 ). Furthermore, the results indicated that two moderator effects were significant. These were the interaction between stress and negative affect ( B = −.28, β = −.39, t = −2.36, p = .02) (see Figure 4 ) and the interaction between positive affect and negative affect ( B = −.21, β = −.29, t = −2.30, p = .02) ( Figure 5 ).
https://doi.org/10.1371/journal.pone.0073265.g003
Low stress and low negative affect leads to lower levels of depression compared to high stress and high negative affect.
https://doi.org/10.1371/journal.pone.0073265.g004
High positive affect and low negative affect lead to lower levels of depression compared to low positive affect and high negative affect.
https://doi.org/10.1371/journal.pone.0073265.g005
The results in the present study show that (i) anxiety partially mediated the effects of both stress and self-esteem on depression, (ii) that stress partially mediated the effects of anxiety and positive affect on depression, (iii) that stress completely mediated the effects of self-esteem on depression, and (iv) that there was a significant interaction between stress and negative affect, and positive affect and negative affect on depression.
Mediating effects
The study suggests that anxiety contributes directly to explaining the variance in depression while stress and self-esteem might contribute directly to explaining the variance in depression and indirectly by increasing feelings of anxiety. Indeed, individuals who experience stress over a long period of time are susceptible to increased anxiety and depression [30] , [31] and previous research shows that high self-esteem seems to buffer against anxiety and depression [32] , [33] . The study also showed that stress partially mediated the effects of both anxiety and positive affect on depression and that stress completely mediated the effects of self-esteem on depression. Anxiety and positive affect contributed directly to explain the variation in depression and indirectly to the experienced level of stress. Self-esteem contributed only indirectly via the experienced level of stress to explain the variation in depression, i.e. stress affects depression on the basis of ‘its own power’ and explains much more of the variation in depressive experiences than self-esteem. In general, individuals who experience low anxiety and frequently experience positive affect seem to experience low stress, which might reduce their levels of depression. Academic stress, for instance, may increase the risk for experiencing depression among students [34] . Although self-esteem did not emerged as an important variable here, under circumstances in which difficulties in life become chronic, some researchers suggest that low self-esteem facilitates the experience of stress [35] .
Moderator effects/interaction effects
The present study showed that the interaction between stress and negative affect and between positive and negative affect influenced self-reported depression symptoms. Moderation effects between stress and negative affect imply that the students experiencing low levels of stress and low negative affect reported lower levels of depression than those who experience high levels of stress and high negative affect. This result confirms earlier findings that underline the strong positive association between negative affect and both stress and depression [36] , [37] . Nevertheless, negative affect by itself did not predicted depression. In this regard, it is important to point out that the absence of positive emotions is a better predictor of morbidity than the presence of negative emotions [38] , [39] . A modification to this statement, as illustrated by the results discussed next, could be that the presence of negative emotions in conjunction with the absence of positive emotions increases morbidity.
The moderating effects between positive and negative affect on the experience of depression imply that the students experiencing high levels of positive affect and low levels of negative affect reported lower levels of depression than those who experience low levels of positive affect and high levels of negative affect. This result fits previous observations indicating that different combinations of these affect dimensions are related to different measures of physical and mental health and well-being, such as, blood pressure, depression, quality of sleep, anxiety, life satisfaction, psychological well-being, and self-regulation [40] – [51] .
Limitations
The result indicated a relatively low mean value for depression ( M = 3.69), perhaps because the studied population was university students. These might limit the generalization power of the results and might also explain why negative affect, commonly associated to depression, was not related to depression in the present study. Moreover, there is a potential influence of single source/single method variance on the findings, especially given the high correlation between all the variables under examination.
Conclusions
The present study highlights different results that could be arrived depending on whether researchers decide to use variables as mediators or moderators. For example, when using meditational analyses, anxiety and stress seem to be important factors that explain how the different variables used here influence depression–increases in anxiety and stress by any other factor seem to lead to increases in depression. In contrast, when moderation analyses were used, the interaction of stress and affect predicted depression and the interaction of both affectivity dimensions (i.e., positive and negative affect) also predicted depression–stress might increase depression under the condition that the individual is high in negative affectivity, in turn, negative affectivity might increase depression under the condition that the individual experiences low positive affectivity.
Acknowledgments
The authors would like to thank the reviewers for their openness and suggestions, which significantly improved the article.
Author Contributions
Conceived and designed the experiments: AAN TA. Performed the experiments: AAN. Analyzed the data: AAN DG. Contributed reagents/materials/analysis tools: AAN TA DG. Wrote the paper: AAN PR TA DG.
- View Article
- Google Scholar
- 3. MacKinnon DP, Luecken LJ (2008) How and for Whom? Mediation and Moderation in Health Psychology. Health Psychol 27 (2 Suppl.): s99–s102.
- 4. Aaroe R (2006) Vinn över din depression [Defeat depression]. Stockholm: Liber.
- 5. Agerberg M (1998) Ut ur mörkret [Out from the Darkness]. Stockholm: Nordstedt.
- 6. Gilbert P (2005) Hantera din depression [Cope with your Depression]. Stockholm: Bokförlaget Prisma.
- 8. Tabachnick BG, Fidell LS (2007) Using Multivariate Statistics, Fifth Edition. Boston: Pearson Education, Inc.
- 10. Beck AT (1967) Depression: Causes and treatment. Philadelphia: University of Pennsylvania Press.
- 21. Eskin M, Parr D (1996) Introducing a Swedish version of an instrument measuring mental stress. Stockholm: Psykologiska institutionen Stockholms Universitet.
- 22. Rosenberg M (1965) Society and the Adolescent Self-Image. Princeton, NJ: Princeton University Press.
- 23. Lindwall M (2011) Självkänsla – Bortom populärpsykologi & enkla sanningar [Self-Esteem – Beyond Popular Psychology and Simple Truths]. Lund:Studentlitteratur.
- 25. Blascovich J, Tomaka J (1991) Measures of self-esteem. In: Robinson JP, Shaver PR, Wrightsman LS (Red.) Measures of personality and social psychological attitudes San Diego: Academic Press. 161–194.
- 30. Eysenck M (Ed.) (2000) Psychology: an integrated approach. New York: Oxford University Press.
- 31. Lazarus RS, Folkman S (1984) Stress, Appraisal, and Coping. New York: Springer.
- 32. Johnson M (2003) Självkänsla och anpassning [Self-esteem and Adaptation]. Lund: Studentlitteratur.
- 33. Cullberg Weston M (2005) Ditt inre centrum – Om självkänsla, självbild och konturen av ditt själv [Your Inner Centre – About Self-esteem, Self-image and the Contours of Yourself]. Stockholm: Natur och Kultur.
- 34. Lindén M (1997) Studentens livssituation. Frihet, sårbarhet, kris och utveckling [Students' Life Situation. Freedom, Vulnerability, Crisis and Development]. Uppsala: Studenthälsan.
- 35. Williams S (1995) Press utan stress ger maximal prestation [Pressure without Stress gives Maximal Performance]. Malmö: Richters förlag.
- 37. Garcia D, Kerekes N, Andersson-Arntén A–C, Archer T (2012) Temperament, Character, and Adolescents' Depressive Symptoms: Focusing on Affect. Depress Res Treat. DOI:10.1155/2012/925372.
- 40. Garcia D, Ghiabi B, Moradi S, Siddiqui A, Archer T (2013) The Happy Personality: A Tale of Two Philosophies. In Morris EF, Jackson M-A editors. Psychology of Personality. New York: Nova Science Publishers. 41–59.
- 41. Schütz E, Nima AA, Sailer U, Andersson-Arntén A–C, Archer T, Garcia D (2013) The affective profiles in the USA: Happiness, depression, life satisfaction, and happiness-increasing strategies. In press.
- 43. Garcia D, Nima AA, Archer T (2013) Temperament and Character's Relationship to Subjective Well- Being in Salvadorian Adolescents and Young Adults. In press.
- 44. Garcia D (2013) La vie en Rose: High Levels of Well-Being and Events Inside and Outside Autobiographical Memory. J Happiness Stud. DOI: 10.1007/s10902-013-9443-x.
- 48. Adrianson L, Djumaludin A, Neila R, Archer T (2013) Cultural influences upon health, affect, self-esteem and impulsiveness: An Indonesian-Swedish comparison. Int J Res Stud Psychol. DOI: 10.5861/ijrsp.2013.228.
Mediation Analysis in Experimental Research
- Reference work entry
- First Online: 03 December 2021
- Cite this reference work entry
- Nicole Koschate-Fischer 4 &
- Elisabeth Schwille 4
7592 Accesses
1 Citations
This chapter introduces the conceptual and statistical basics of mediation analysis in the context of experimental research. Adopting the respective terminology, mediation analysis can be referred to as an array of quantitative methods developed to investigate the causal mechanism(s) through which an independent variable influences a dependent variable. The chapter takes a regression-based approach to mediation analysis and focuses on mediation models likely to be tested in experiments (i.e., the single mediator model, parallel and serial multiple mediator models, and conditional process models). Yet, the scope of mediation analysis beyond an experimental setting will also be touched upon. Furthermore, the chapter addresses the question how to strengthen causal inference in mediation analysis through design, the collection of additional evidence, and statistical methods. It closes with a discussion of common topics of relevance when implementing mediation analysis such as sample size and power, mean centering in conditional process analysis, coding of categorical independent variables, advantages and disadvantages of a regression-based approach to mediation analysis, and software options to perform mediation analysis.
This is a preview of subscription content, log in via an institution to check access.
Access this chapter
- Available as PDF
- Read on any device
- Instant download
- Own it forever
- Available as EPUB and PDF
- Durable hardcover edition
- Dispatched in 3 to 5 business days
- Free shipping worldwide - see info
Tax calculation will be finalised at checkout
Purchases are for personal use only
Institutional subscriptions
Baron, R. M., & Kenny, D. A. (1986). The moderator-mediator variable distinction in social psychological research: Conceptual, strategic, and statistical considerations. Journal of Personality and Social Psychology, 51 (6), 1173–1182.
Google Scholar
Berger, J. (2014). Word of mouth and interpersonal communication: A review and directions for future research. Journal of Consumer Psychology, 24 (4), 586–607.
Bollen, K. A. (1989). Structural equations with latent variables . New York: Wiley.
Book Google Scholar
Bollen, K. A., & Stine, R. (1990). Direct and indirect effects: Classical and bootstrap estimates of variability. Sociological Methodology, 20 (1), 115–140.
Bullock, J. G., Green, D. P., & Ha, S. E. (2010). Yes, but what’s the mechanism? (Don’t expect an easy answer). Journal of Personality and Social Psychology, 98 (4), 550–558.
Cavanaugh, L. A. (2014). Because I (don’t) deserve it: How relationship reminders and deservingness influence consumer indulgence. Journal of Marketing Research, 51 (2), 218–232.
Chandon, P., Wansink, B., & Laurent, G. (2000). A benefit congruency framework of sales promotion effectiveness. Journal of Marketing, 64 (4), 65–81.
Cole, D. A., & Maxwell, S. E. (2003). Testing mediational models with longitudinal data: Questions and tips in the use of structural equation modeling. Journal of Abnormal Psychology, 112 (4), 558–577.
Cole, D. A., & Preacher, K. J. (2014). Manifest variable path analysis: Potentially serious and misleading consequences due to uncorrected measurement error. Psychological Methods, 19 (2), 300–315.
Cook, T. D., & Campbell, D. T. (1979). Quasi-experimentation: Design and analysis for field settings . Boston: Houghton Mifflin.
Dalal, D. K., & Zickar, M. J. (2012). Some common myths about centering predictor variables in moderated multiple regression and polynomial regression. Organizational Research Methods, 15 (3), 339–362.
Darlington, R. B., & Hayes, A. F. (2017). Regression analysis and linear models: Concepts, applications, and implementation . New York: Guilford Press.
Echambadi, R., & Hess, J. D. (2007). Mean-centering does not alleviate collinearity problems in moderated multiple regression models. Marketing Science, 26 (3), 438–445.
Edwards, J. R., & Lambert, L. S. (2007). Methods for integrating moderation and mediation: A general analytical framework using moderated path analysis. Psychological Methods, 12 (1), 1–22.
Efron, B. (1987). Better bootstrap confidence intervals. Journal of the American Statistical Association, 82 (397), 171–185.
Fairchild, A. J., & MacKinnon, D. P. (2009). A general model for testing mediation and moderation effects. Prevention Science, 10 (2), 87–99.
Frazier, P., Tix, A. P., & Barron, K. E. (2004). Testing moderator and mediator effects in counseling psychology research. Journal of Counseling Psychology, 51 (1), 115–134.
Fritz, M. S., & MacKinnon, D. P. (2007). Required sample size to detect the mediated effect. Psychological Science, 18 (3), 233–239.
Fritz, M. S., Taylor, A. B., & MacKinnon, D. P. (2012). Explanation of two anomalous results in statistical mediation analysis. Multivariate Behavioral Research, 47 (1), 61–87.
Fritz, M. S., Cox, M. G., & MacKinnon, D. P. (2015). Increasing statistical power in mediation models without increasing sample size. Evaluation & the Health Professions, 38 (3), 343–366.
Fritz, M. S., Kenny, D. A., & MacKinnon, D. P. (2016). The combined effects of measurement error and omitting confounders in the single-mediator model. Multivariate Behavioral Research, 51 (5), 681–697.
Hair, J. F., Black, W. C., Babin, B. J., Anderson, R. E., & Tatham, R. L. (2006). Multivariate data analysis . Upper Saddle River: Pearson Prentice Hall.
Hansen, W. B., & McNeal, R. B. (1996). The law of maximum expected potential effect: Constraints placed on program effectiveness by mediator relationships. Health Education Research, 11 (4), 501–507.
Hayes, A. F. (2009). Beyond Baron and Kenny: Statistical mediation analysis in the new millennium. Communication Monographs, 76 (4), 408–420.
Hayes, A. F. (2018). Introduction to mediation, moderation, and conditional process analysis: A regression-based approach . New York: Guilford Press.
Hayes, A. F. (2015). An index and test of linear moderated mediation. Multivariate Behavioral Research, 50 (1), 1–22.
Hayes, A. F. (2017). Partial, conditional, and moderated moderated mediation: Quantification, inference, and interpretation. Communication Monographs . https://doi.org/10.1080/03637751-2017-1352100 .
Hayes, A. F., & Cai, L. (2007). Using heteroskedasticity-consistent standard error estimators in OLS regression: An introduction and software implementation. Behavior Research Methods, 39 (4), 709–722.
Hayes, A. F., & Preacher, K. J. (2010). Quantifying and testing indirect effects in simple mediation models when the constituent paths are nonlinear. Multivariate Behavioral Research, 45 (4), 627–660.
Hayes, A. F., & Preacher, K. J. (2014). Statistical mediation analysis with a multicategorical independent variable. British Journal of Mathematical and Statistical Psychology, 67 (3), 451–470.
Hayes, A. F., & Scharkow, M. (2013). The relative trustworthiness of inferential tests of the indirect effect in statistical mediation analysis does method really matter? Psychological Science, 24 (10), 1918–1927.
Hayes, A. F., Montoya, A. K., & Rockwood, N. J. (2017). The analysis of mechanisms and their contingencies: PROCESS versus structural equation modeling. Australasian Marketing Journal, 25 (1), 76–81.
Hoyle, R. H., & Kenny, D. A. (1999). Sample size, reliability, and tests of statistical mediation. In R. H. Hoyle (Ed.), Statistical strategies for small sample research (pp. 195–222). Thousand Oaks: Sage.
Iacobucci, D. (2012). Mediation analysis and categorical variables: The final frontier. Journal of Consumer Psychology, 22 (4), 582–594.
Iacobucci, D., Saldanha, N., & Deng, X. (2007). A meditation on mediation: Evidence that structural equations models perform better than regressions. Journal of Consumer Psychology, 17 (2), 139–153.
Imai, K., Keele, L., & Tingley, D. (2010). A general approach to causal mediation analysis. Psychological Methods, 15 (4), 309–334.
Imai, K., Keele, L., Tingley, D., & Yamamoto, T. (2011). Unpacking the black box of causality: Learning about causal mechanisms from experimental and observational studies. American Political Science Review, 105 (4), 765–789.
Imai, K., Tingley, D., & Yamamoto, T. (2013). Experimental designs for identifying causal mechanisms. Journal of the Royal Statistical Society: Series A (Statistics in Society), 176 (1), 5–51.
Jacoby, J., & Sassenberg, K. (2011). Interactions do not only tell us when, but can also tell us how: Testing process hypotheses by interaction. European Journal of Social Psychology, 41 (2), 180–190.
James, L. R., Mulaik, S. A., & Brett, J. M. (2006). A tale of two methods. Organizational Research Methods, 9 (2), 233–244.
Jose, P. E. (2013). Doing statistical mediation and moderation . New York: Guilford Press.
Judd, C. M., & Kenny, D. A. (1981). Process analysis estimating mediation in treatment evaluations. Evaluation Review, 5 (5), 602–619.
Kelley, K. (2007). Methods for the behavioral, educational, and social sciences: An R package. Behavior Research Methods, 39 (4), 979–984.
Kenny, D. A. (2008). Reflections on mediation. Organizational Research Methods, 11 (2), 353–358.
Kenny, D. A., & Judd, C. M. (2014). Power anomalies in testing mediation. Psychological Science, 25 (2), 334–339.
Kisbu-Sakarya, Y., MacKinnon, D. P., & Miočević, M. (2014). The distribution of the product explains normal theory mediation confidence interval estimation. Multivariate Behavioral Research, 49 (3), 261–268.
Koschate-Fischer, N., & Schandelmeier, S. (2014). A guideline for designing experimental studies in marketing research and a critical discussion of selected problem areas. Journal of Business Economics, 84 (6), 793–826.
Koschate-Fischer, N., Stefan, I. V., & Hoyer, W. D. (2012). Willingness to pay for cause-related marketing: The impact of donation amount and moderating effects. Journal of Marketing Research, 49 (6), 910–927.
Koschate-Fischer, N., Huber, I. V., & Hoyer, W. D. (2016). When will price increases associated with company donations to charity be perceived as fair? Journal of the Academy of Marketing Science, 44 (5), 608–626.
Koschate-Fischer, N., Hoyer, W. D., Stokburger-Sauer, N. E., & Engling, J. (2017). Do life events always lead to change in purchase? The mediating role of change in consumer innovativeness, the variety seeking tendency, and price consciousness. Journal of the Academy of Marketing Science . https://doi.org/10.1007/s11747-017-0548-3 .
Kraemer, H. C., Wilson, G. T., Fairburn, C. G., & Agras, W. S. (2002). Mediators and moderators of treatment effects in randomized clinical trials. Archives of General Psychiatry, 59 (10), 877–883.
Kraemer, H. C., Kiernan, M., Essex, M., & Kupfer, D. J. (2008). How and why criteria defining moderators and mediators differ between the Baron & Kenny and MacArthur approaches. Health Psychology, 27 (2S), 101–108.
Lemmer, G., & Gollwitzer, M. (2017). The “true” indirect effect won’t (always) stand up: When and why reverse mediation testing fails. Journal of Experimental Social Psychology, 69 , 144–149.
Lichtenstein, D. R., Netemeyer, R. G., & Burton, S. (1995). Assessing the domain specificity of deal proneness: A field study. Journal of Consumer Research, 22 (3), 314–326.
MacKinnon, D. P. (2008). Introduction to statistical mediation analysis . New York: Routledge.
MacKinnon, D. P., & Dwyer, J. H. (1993). Estimating mediated effects in prevention studies. Evaluation Review, 17 (2), 144–158.
MacKinnon, D. P., & Pirlott, A. G. (2015). Statistical approaches for enhancing causal interpretation of the M to Y relation in mediation analysis. Personality and Social Psychology Review, 19 (1), 30–43.
MacKinnon, D. P., Warsi, G., & Dwyer, J. H. (1995). A simulation study of mediated effect measures. Multivariate Behavioral Research, 30 (1), 41–62.
MacKinnon, D. P., Krull, J. L., & Lockwood, C. M. (2000). Equivalence of the mediation, confounding and suppression effect. Prevention Science, 1 (4), 173–181.
MacKinnon, D. P., Lockwood, C. M., Hoffman, J. M., West, S. G., & Sheets, V. (2002). A comparison of methods to test mediation and other intervening variable effects. Psychological Methods, 7 (1), 83–104.
MacKinnon, D. P., Lockwood, C. M., & Williams, J. (2004). Confidence limits for the indirect effect: Distribution of the product and resampling methods. Multivariate Behavioral Research, 39 (1), 99–128.
MacKinnon, D. P., Fritz, M. S., Williams, J., & Lockwood, C. M. (2007a). Distribution of the product confidence limits for the indirect effect: Program PRODCLIN. Behavior Research Methods, 39 (3), 384–389.
MacKinnon, D. P., Fairchild, A. J., & Fritz, M. S. (2007b). Mediation analysis. Annual Review of Psychology, 58 , 593–614.
MacKinnon, D. P., Kisbu-Sakarya, Y., & Gottschall, A. C. (2013). Developments in mediation analysis. In T. D. Little (Ed.), The Oxford handbook of quantitative methods in psychology: Volume 2: Statistical analysis (pp. 338–360). New York: Oxford University Press.
Mathieu, J. E., & Taylor, S. R. (2006). Clarifying conditions and decision points for mediational type inferences in organizational behavior. Journal of Organizational Behavior, 27 (8), 1031–1056.
Maxwell, S. E., & Cole, D. A. (2007). Bias in cross-sectional analyses of longitudinal mediation. Psychological Methods, 12 (1), 23–44.
Maxwell, S. E., Cole, D. A., & Mitchell, M. A. (2011). Bias in cross-sectional analyses of longitudinal mediation: Partial and complete mediation under an autoregressive model. Multivariate Behavioral Research, 46 (5), 816–841.
Miller, G. A., & Chapman, J. P. (2001). Misunderstanding analysis of covariance. Journal of Abnormal Psychology, 110 (1), 40–48.
Montoya, A. K., & Hayes, A. F. (2017). Two condition within-participant statistical mediation analysis: A path-analytic framework. Psychological Methods, 22 (1), 6–27.
Morgan-Lopez, A. A., & MacKinnon, D. P. (2006). Demonstration and evaluation of a method for assessing mediated moderation. Behavior Research Methods, 38 (1), 77–87.
Muller, D., Judd, C. M., & Yzerbyt, V. Y. (2005). When moderation is mediated and mediation is moderated. Journal of Personality and Social Psychology, 89 (6), 852–863.
Muthén, L. K., & Muthén, L. (1998). Mplus [computer software] . Los Angeles: Muthén & Muthén.
Pek, J., & Hoyle, R. H. (2016). On the (in) validity of tests of simple mediation: Threats and solutions. Social and Personality Psychology Compass, 10 (3), 150–163.
Pieters, R. (2017). Meaningful mediation analysis: Plausible causal inference and informative communication. Journal of Consumer Research, 44 (3), 692–716.
Pirlott, A. G., & MacKinnon, D. P. (2016). Design approaches to experimental mediation. Journal of Experimental Social Psychology, 66 , 29–38.
Preacher, K. J. (2015). Advances in mediation analysis: A survey and synthesis of new developments. Annual Review of Psychology, 66 (1), 825–852.
Preacher, K. J., & Hayes, A. F. (2008). Asymptotic and resampling strategies for assessing and comparing indirect effects in multiple mediator models. Behavior Research Methods, 40 (3), 879–891.
Preacher, K. J., & Kelley, K. (2011). Effect size measures for mediation models: Quantitative strategies for communicating indirect effects. Psychological Methods, 16 (2), 93–115.
Preacher, K. J., & Selig, J. P. (2012). Advantages of Monte Carlo confidence intervals for indirect effects. Communication Methods and Measures, 6 (2), 77–98.
Preacher, K. J., Rucker, D. D., & Hayes, A. F. (2007). Addressing moderated mediation hypotheses: Theory, methods, and prescriptions. Multivariate Behavioral Research, 42 (1), 185–227.
Revelle, W. (2016). psych: Procedures for psychological, psychometric, and personality research (Version 1.6.12). http://personality-project.org/r, http://personality-project.org/r/psych-manual.pdf . Accessed 24 July 2017.
Rosseel, Y. (2012). Lavaan: An R package for structural equation modeling. Journal of Statistical Software, 48 (2), 1–36.
Rucker, D. D., Preacher, K. J., Tormala, Z. L., & Petty, R. E. (2011). Mediation analysis in social psychology: Current practices and new recommendations. Social and Personality Psychology Compass, 5 (6), 359–371.
Savary, J., Goldsmith, K., & Dhar, R. (2014). Giving against the odds: When tempting alternatives increase willingness to donate. Journal of Marketing Research, 52 (1), 27–38.
Shrout, P. E., & Bolger, N. (2002). Mediation in experimental and nonexperimental studies: New procedures and recommendations. Psychological Methods, 7 (4), 422–445.
Sobel, M. E. (1982). Asymptotic confidence intervals for indirect effects in structural equation models. Sociological Methodology, 13 , 290–312.
Spencer, S. J., Zanna, M. P., & Fong, G. T. (2005). Establishing a causal chain: Why experiments are often more effective than mediational analyses in examining psychological processes. Journal of Personality and Social Psychology, 89 (6), 845–851.
Stone-Romero, E. F., & Rosopa, P. J. (2008). The relative validity of inferences about mediation as a function of research design characteristics. Organizational Research Methods, 11 (2), 326–352.
Taylor, A. B., MacKinnon, D. P., & Tein, J.-Y. (2008). Tests of the three-path mediated effect. Organizational Research Methods, 11 (2), 241–269.
Thoemmes, F. (2015). Reversing arrows in mediation models does not distinguish plausible models. Basic and Applied Social Psychology, 37 (4), 226–234.
Thoemmes, F., MacKinnon, D. P., & Reiser, M. R. (2010). Power analysis for complex mediational designs using Monte Carlo methods. Structural Equation Modeling, 17 (3), 510–534.
Tingley, D., Yamamoto, T., Hirose, K., Keele, L., & Imai, K. (2014). Mediation: R package for causal mediation analysis. Journal of Statistical Software, 59 (5), 1–38.
Tofighi, D., & MacKinnon, D. P. (2011). RMediation: An R package for mediation analysis confidence intervals. Behavior Research Methods, 43 (3), 692–700.
Tofighi, D., & Thoemmes, F. (2014). Single-level and multilevel mediation analysis. The Journal of Early Adolescence, 34 (1), 93–119.
Touré-Tillery, M., & McGill, A. L. (2015). Who or what to believe: Trust and the differential persuasiveness of human and anthropomorphized messengers. Journal of Marketing, 79 (4), 94–110.
Valeri, L., & VanderWeele, T. J. (2013). Mediation analysis allowing for exposure-mediator interactions and causal interpretation: Theoretical assumptions and implementation with SAS and SPSS macros. Psychological Methods, 18 (2), 137–150.
VanderWeele, T. J. (2015). Explanation in causal inference: Methods for mediation and interaction . New York: Oxford University Press.
VanderWeele, T. J., & Vansteelandt, S. (2014). Mediation analysis with multiple mediators. Epidemiologic Methods, 2 (1), 95–115.
Wen, Z., & Fan, X. (2015). Monotonicity of effect sizes: Questioning kappa-squared as mediation effect size measure. Psychological Methods, 20 (2), 193–203.
Williams, J., & MacKinnon, D. P. (2008). Resampling and distribution of the product methods for testing indirect effects in complex models. Structural Equation Modeling: A Multidisciplinary Journal, 15 (1), 23–51.
Yuan, Y., & MacKinnon, D. P. (2014). Robust mediation analysis based on median regression. Psychological Methods, 19 (1), 1–20.
Zhao, X., Lynch, J. G., & Chen, Q. (2010). Reconsidering Baron and Kenny: Myths and truths about mediation analysis. Journal of Consumer Research, 37 (2), 197–206.
Download references
Author information
Authors and affiliations.
University of Erlangen-Nuremberg, Nuremberg, Germany
Nicole Koschate-Fischer & Elisabeth Schwille
You can also search for this author in PubMed Google Scholar
Corresponding author
Correspondence to Nicole Koschate-Fischer .
Editor information
Editors and affiliations.
Department of Business-to-Business Marketing, Sales, and Pricing, University of Mannheim, Mannheim, Germany
Christian Homburg
Department of Marketing & Sales Research Group, Karlsruhe Institute of Technology (KIT), Karlsruhe, Germany
Martin Klarmann
Marketing & Sales Department, University of Mannheim, Mannheim, Germany
Arnd Vomberg
Rights and permissions
Reprints and permissions
Copyright information
© 2022 Springer Nature Switzerland AG
About this entry
Cite this entry.
Koschate-Fischer, N., Schwille, E. (2022). Mediation Analysis in Experimental Research. In: Homburg, C., Klarmann, M., Vomberg, A. (eds) Handbook of Market Research. Springer, Cham. https://doi.org/10.1007/978-3-319-57413-4_34
Download citation
DOI : https://doi.org/10.1007/978-3-319-57413-4_34
Published : 03 December 2021
Publisher Name : Springer, Cham
Print ISBN : 978-3-319-57411-0
Online ISBN : 978-3-319-57413-4
eBook Packages : Business and Management Reference Module Humanities and Social Sciences Reference Module Business, Economics and Social Sciences
Share this entry
Anyone you share the following link with will be able to read this content:
Sorry, a shareable link is not currently available for this article.
Provided by the Springer Nature SharedIt content-sharing initiative
- Publish with us
Policies and ethics
- Find a journal
- Track your research
CONCEPTUAL ANALYSIS article
On the interpretation and use of mediation: multiple perspectives on mediation analysis.
- 1 Department of Psychology, Ohio State University, Columbus, OH, United States
- 2 Division of Epidemiology, College of Public Health, Ohio State University, Columbus, OH, United States
- 3 Department of Psychology, KU Leuven, Leuven, Belgium
Mediation analysis has become a very popular approach in psychology, and it is one that is associated with multiple perspectives that are often at odds, often implicitly. Explicitly discussing these perspectives and their motivations, advantages, and disadvantages can help to provide clarity to conversations and research regarding the use and refinement of mediation models. We discuss five such pairs of perspectives on mediation analysis, their associated advantages and disadvantages, and their implications: with vs. without a mediation hypothesis, specific effects vs. a global model, directness vs. indirectness of causation, effect size vs. null hypothesis testing, and hypothesized vs. alternative explanations. Discussion of the perspectives is facilitated by a small simulation study. Some philosophical and linguistic considerations are briefly discussed, as well as some other perspectives we do not develop here.
Introduction
Without respect to a given statistical model, mediation processes are framed in terms of intermediate variables between an independent variable and a dependent variable, with a minimum of three variables required in total: X , M , and Y , where X is the independent variable (IV), Y is the dependent variable (DV), and M is the (hypothesized) mediator variable that is supposed to transmit the causal effect of X to Y . The total effect of X on Y is referred to as the total effect ( TE ), and that effect is then partitioned into a combination of a direct effect (DE) of X on Y , and an indirect effect ( IE ) of X on Y that is transmitted through M . In other words, the relationship between X and Y is decomposed into a direct link and an indirect link.
While the conceptual model of mediation is straight-forward, applying it is much less so ( Bullock et al., 2010 ). There are multiple schools of thought and discussions regarding mediation that provide detailed arguments and criteria regarding mediation claims for specific models or sets of assumptions (e.g., Baron and Kenny, 1986 ; Kraemer et al., 2002 ; Jo, 2008 ; Pearl, 2009 ; Imai et al., 2010 ). As still further evidence of the difficulty of making mediation claims, parameter bias, and sensitivity have emerged as common concerns (e.g., Sobel, 2008 ; Imai et al., 2010 ; VanderWeele, 2010 ; Fritz et al., 2016 ), as has statistical power for testing both indirect (e.g., Shrout and Bolger, 2002 ; Fritz and MacKinnon, 2007 ; Preacher and Hayes, 2008 ) and total effects ( Kenny and Judd, 2014 ; Loeys et al., 2015 ; O'Rourke and MacKinnon, 2015 ).
Relatively untouched is that there are cross-cutting concerns related to the fact that what is considered appropriate for a mediation claim depends not only on statistical and theoretical criteria, but also on the experience, assumptions, needs, and general point of view of a researcher. Some perspectives may be more often correct than others (e.g., more tenable assumptions, better clarification of what constitutes a mediator, etc.), but all perspectives and models used by researchers are necessarily incomplete and unable to fully capture all considerations necessary for conducting research, leaving some approaches ill-suited for certain tasks. This is in line with a recent article by Gelman and Hennig (2017) , who note that while the tendency in the literature is to find and formulate one best approach based on seemingly objective criteria there is nonetheless unavoidable subjectivity involved in any statistical decision. Researchers always view only a subset of reality, and rather than denying this it is advantageous—even necessary—to embrace that there are multiple perspectives relevant to any statistical discussion.
The aim of the article is not to propose new approaches or to criticize existing approaches, but to explain that the existence and use of multiple perspectives is both useful and sensible for mediation analysis. We use the term mediation in the general sense that a mediation model explains values of Y as indirectly caused by values of X , without favoring any specific statistical model or set of identifying assumptions. The three variables may be exhaustive, or a subset of much larger set of variables. As we discuss, there can be value in different and divergent considerations and convergence is not required or uniformly advantageous. Our points here are more general than any specific statistical model (and their IE, DE , and TE estimates and tests), but there are a few points that require we first review simple mediation models as estimated by ordinary least squares linear regression. We will then take the concept of mediation to an extreme with a time-series example, using the example to illustrate and discuss the various perspectives, not as a representative case but to clarify some issues.
Mediation with Linear Regression
Within a regression framework, the population parameters a, b, c , and c ′ (Figures 1 , 2 ) are estimated not with a single statistical model, but rather a set of either two or three individual regression models. We say two or three because the first, Model 1, is somewhat controversial and is not always necessary ( Kenny and Judd, 2014 ). This model yields the sample regression weight c as an estimate of the TE:
Models 2 and 3 are used to estimate the DE and IE . Specifically, the DE is presented as the path from X to Y , c ′. The IE is estimated by the product of the path from X to M (Model 2) and the path from M to Y (Model 3), i.e., the product of the regression weights a and b . The equations for these two models are as follows:
Together, these two models yield the direct effect, c ′, as well as the indirect effect ab . Further, the summation of these two effects is equal to the total effect, i.e., c = c ′+ ab . Assuming no missing data and a saturated model (as in the case of Equations 2 and 3) this value of c is equal to that provided by Model 1.
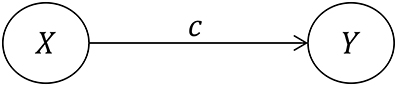
Figure 1 . Effect of X and Y without considering mediation.
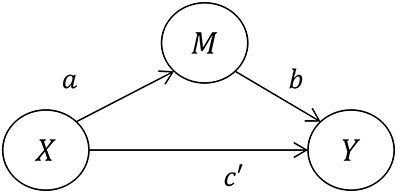
Figure 2 . Effect of X on Y including mediation.
The total effect can then be inferred in two different ways, either based on Figure 1 (Model 1) or on Figure 2 (a combination of Models 2 and 3), but as we will discuss there are important conceptual differences between these two numerically identical total effects. We will refer to the TE associated with Figure 1 as TE 1 , and the TE associated with Figure 2 as TE 2 .
A Time Series Example
To take the concept of mediation to an extreme, imagine a stationary autoregressive process for T equidistant time points (e.g., T consecutive days) with a lag of 1 as in the most simple autoregressive time series model, i.e., AR (1). In such a model the expected correlation between consecutive observations is stable (stationary), and the model is equivalent with a full and exclusively serial mediation model without any direct effect. X is measured at t = 1 and Y is a measured at t = T . The independent variable X has an effect on M t = 2 , which in turn has an effect on M t = 3 , and so on up to M t = T−1 having an effect on Y at t = T . In mediation terms, there are T -2 mediators, from M t = 2 to M T −1 , with an effect only on the next mediator and finally on Y . Although this kind of mediation is an extreme case compared with the typical simple mediation model, it is nonetheless mediation in the sense that all effects are transmitted by way of an intervening effect. As a result, regardless of the time scale, the TE always equals the IE . Although extreme, such a model is a reasonable one for some time series data, e.g., it seems quite realistic that one's general mood (as distinct from ephemeral emotional states) of today mediates between one's mood of yesterday and one's mood of tomorrow. For some variables, there may be also an effect from earlier values than the previous measurement, i.e., longer lags, but such a more complex process is still a mediation process.
To help make our points more concrete we conducted a small-scale simulation. We generated data for 3, 10, 50, or 100 time points with a constant correlation of 0.10, 0.50, or 0.90 between consecutive time points, and with N = 10, 50, or 100 for each, for a total of 36 conditions. Initial time points were drawn from a standard normal distribution. We generated 500 replications per condition. All tests were done using 5,000 bootstraps and α = 0.05. These results are shown in Table 1 . One can easily see that rejections of the null hypothesis for the total effect TE 1 scarcely exceed the α level in nearly all conditions, which is unsurprising because of the near zero magnitude of the total effect. The only exceptions to these low rejections rates were for N = 10—but this is due to bootstrapping underestimating the standard error here for such small sample sizes—and for cases where the TE was of appreciable magnitude, i.e., for T = 3 and r = 0.5 or 0.9 or T = 10 and r = 0.9 ( TE = IE = 0.25, 0.81, and 0.38742, respectively). For such large effects the TE 1 is easily rejected. In contrast, the indirect effect is almost always significant, and the rejection rates are always greater than those of the TE 1 , even when the true size of the indirect effect is extremely small (as small as the true total effect). For nearly all cases where r = 0.5 or 0.9 the test of the IE exhibited higher power than the test of the TE 1 , with the minor caveat that for r = 0.5 and N = 10 the difference was minimal. In total, for 20 conditions of the 36 we considered here, rejection rates were 89–100%, with the observed power advantage for the IE relative to the TE 1 as great as 94% higher (6 vs. 100%) when the TE 1 is small, e.g., when T = 50 or 100. We will use this illustration to elaborate on the different perspectives on mediation, and specific aspects of the results will be focused on as necessary for the perspectives we discuss.
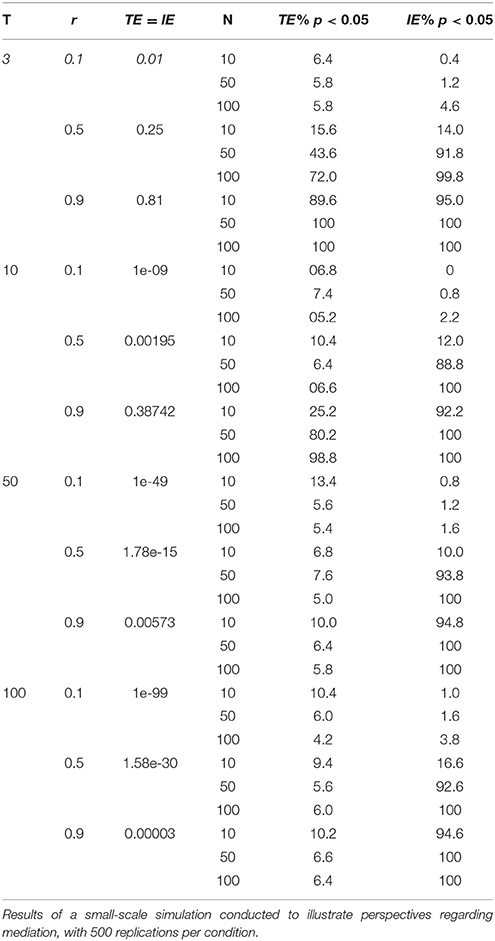
Table 1 . Simulation results.
Five Pairs of Perspectives
Each of the five pairs of perspectives we discuss here offers a choice regarding how to view, use, and study mediation models. Each of the perspectives we discuss here has its own merits, and we do not mean to imply that any perspective or approach we discuss here is “better”—there are simply too many criteria to exhaust to evaluate such a claim, and researchers must work within the context of the problem at hand to decide what is most appropriate.
We dichotomize and treat each perspective both within and between pairs as largely independent for the purposes of explication, but there are many points of intersection and we do not wish to imply an absence of a middle ground or that each perspective from a given pair cannot be meaningfully integrated. The perspectives we discuss here are not meant to be exhaustive, and were selected because of their relevance to common topics in the mediation literature. No pair of perspectives is strictly limited to any one topic, as the various discussions regarding mediation are each better understood when looked at from multiple angles. A brief summary of each pair of perspectives we discuss is provided in Table 2 , as well as a few example areas of research where the perspectives are relevant.
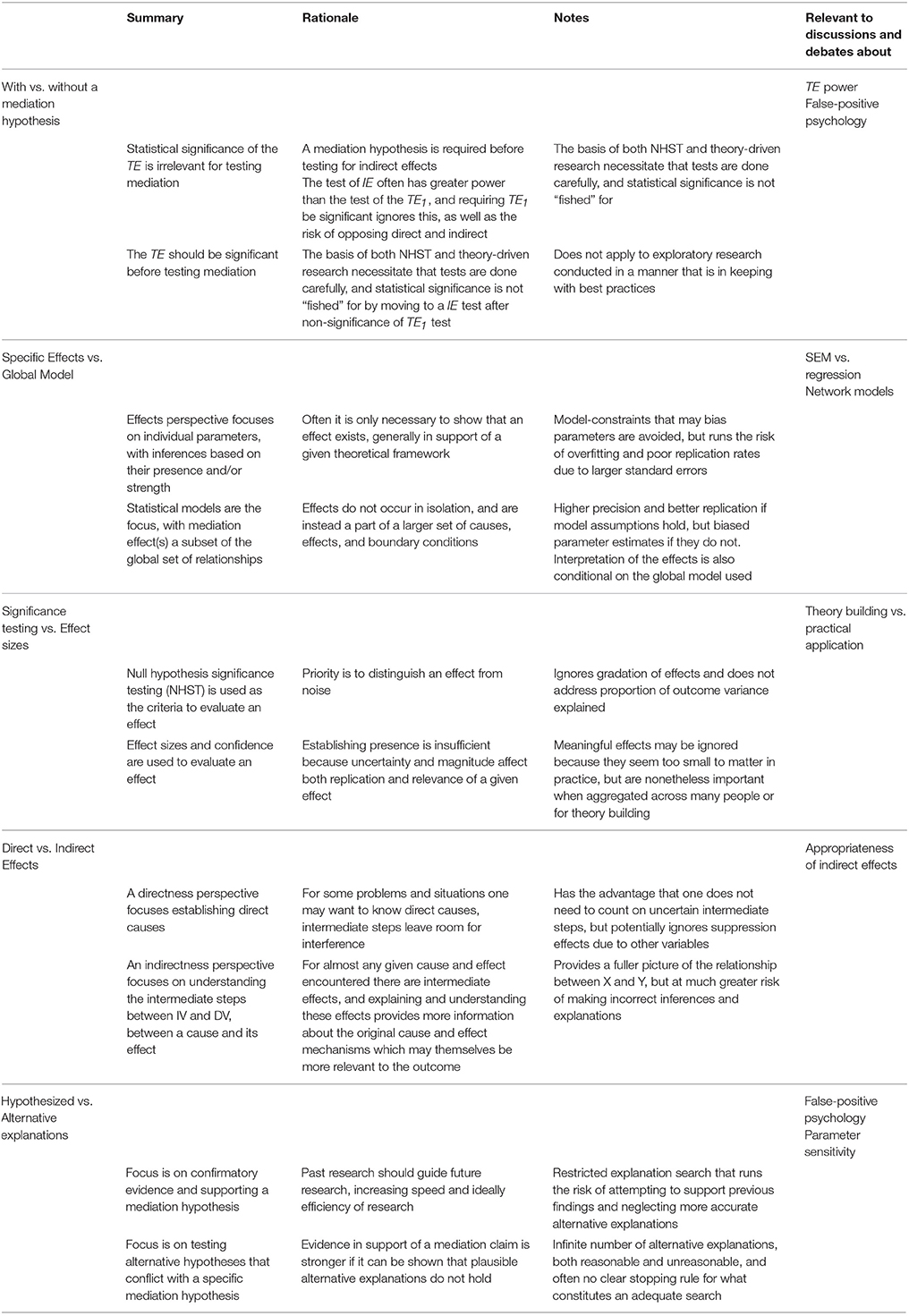
Table 2 . Comparison of perspectives.
With vs. Without a Mediation Hypothesis
A common concern that has emerged in the mediation literature is whether or not TE 1 should be required before testing indirect effects. Given that the reason researchers use mediation analysis is to test for indirect effects, whether or not there is a total effect can seem an irrelevant preliminary condition. Our time-series example is one example of why the presence of TE 1 is not required for an indirect effect to be detected with a null hypothesis test, but even in more mundane cases involving three variables the IE test has greater power than the TE 1 test under some parameter configurations ( Rucker et al., 2011 ; Kenny and Judd, 2014 ; Loeys et al., 2015 ; O'Rourke and MacKinnon, 2015 ). Further, two competing effects can suppress each other ( MacKinnon et al., 2000 ) such that two roughly equal (and potentially large) direct and indirect effects of opposing direction can result in a near-zero total effect. As can be seen in Table 1 , a large proportion of the tests of the IE were significant even when the corresponding test of the TE 1 was not significant. These are not new findings, but they illustrate that even for extremely small effect sizes such as, at the bottom of Table 1 (e.g., 1.58e-30) the IE is significant. Given a mediation hypothesis there is then no need to consider the significance of the TE 1 because it is irrelevant to the presence of an IE , as the IE is estimated by different statistical models than TE 1 is and a mediation hypothesis refers solely to the IE (though a more general causal relationship may be hypothesized to include both).
However, such work should not be taken as a blanket justification for testing the IE in the absence of TE 1 if there is not an a priori hypothesized indirect effect. While there is great value and need for exploratory research (with later replication and validation in a separate study) and we do not wish to discourage such practices, if the XY relationship is not significant based on Model 1 then one is likely better served by staying with the null hypothesis of no relationship because of the increased risk of false positives associated with so-called “fishing expeditions” ( Wagenmakers et al., 2011 ). Although a non-significant relationship does not exclude the possibility that there is a true and perhaps mediated relationship between X and Y —the world is full of relationships that cannot be differentiated from noise without consideration of indirect effects—a preference for parsimony and a desire to avoid false positives would suggest that one does not generate additional explanations for relationships that are not significant when first tested. Although the results shown in Table 1 show that a large proportion of indirect are significant in the absence of a significant TE 1 it would not be a good idea to follow up all non-significant correlations, regression weights, F -tests, t -tests, etc. with a post-hoc mediation analysis and then attempting to explain it after the results are known ( Kerr, 1998 ). When working with real data there are simply too many alternative explanations to consider. Absent an a priori hypothesis, the Judd and Kenny (1981) and Baron and Kenny (1986) condition requiring that the relationship between X and Y be significant makes sense.
The two perspectives represent two different and contrasting lines of reasoning and motivations—either the study is based on a mediation hypothesis or it is not. If it is, there is no preliminary condition regarding the total effect because it is irrelevant to whether or not an indirect effect may be present. It is simply necessary to conduct the appropriate test for the indirect effect. If however there was no pre-specified hypothesis, the logic of null hypothesis significance testing (NHST) requires that one stays with the conclusion of no relationship if the null hypothesis is not rejected by the data rather than conducting additional unplanned tests (with the caveat that appropriate corrections for multiple comparisons may be employed).
Specific Effects vs. Global Model
To put it colloquially, this pair of perspectives refers to whether one is interested in the forest or in the tree when investigating mediation. An effect-focused approach implies that a global model for all relationships is less important, and that one focuses instead on the tests of the effects of interest. These effects can be tested within a global statistical model (i.e., one can be interested in specific effects while still estimating all relationships), or from separate regression models. In the latter case, the global model is then primarily a conceptual one because there is not one statistical model to be used for estimation of the effects. For example, when using separate regressions the indirect effect is the product of two parameters from different statistical models, and while TE 1 is an effect in one model, TE 2 is a composite of two effects that stem from two separate models.
In contrast, a globally focused approach implies formulating and testing a global model for all variables, evaluating it based on relevant criteria (e.g., model fit, theoretical defensibility). The various examples of network models are examples of global models ( Salter-Townshend et al., 2012 ), but most commonly in the social sciences global models are realized using a structural equation model approach (SEM) for the covariance of the three variables, with or without making use of any latent variables ( Iacobucci et al., 2007 ; MacKinnon, 2008 ). If latent variables are used then there is the advantage of correcting for measurement error, but it is not necessary to use latent variables in a global model. Within the model, the specific mediation effect can be derived as a product of single path effects (e.g., Rijnhart et al., 2017 ).
The choice between, and discussions regarding, these two approaches comes with a few relevant considerations. First, there is the matter of model saturation (i.e., the same number of estimated parameters as there are variables). For the simple situation of one mediator variable and thus three variables in total, and effects described by a, b , and c ′, the global model is a saturated model, and as a result the point estimate of the indirect effect is the same whether one uses different regression models or one global SEM. To some degree then the matter of specific effects vs. the global model distinction is irrelevant because simple mediation models are saturated. However, when the mediation relationships are more complex the global model is no longer necessarily a saturated model. For example, a two-mediator model is either a serial or parallel mediator model, with the former having a path between the two mediators and the latter not ( Hayes, 2013 ). As such, a parallel two-mediator model is not saturated whereas a serial two-mediator model is. In general, from a global model perspective one would first want to test the goodness of fit of the global model, before a particular mediation effect is considered at all because the effects are conditional on the model.
Second, the power anomaly discussed in recent work reflects an effect-focused perspective based on separate regressions and vanishes when one focuses on the effect within a global statistical model, where the covariance between X and Y is simply a descriptive statistic used for model estimation and not a parameter (i.e., not a total effect to estimate). The total effect is estimated through two within-model effects. TE 1 is one observed covariance among the other observed covariance measures to be explained with the model. Further, instead of two separate TE estimates (stemming from separate regressions), there is only one TE to be considered: TE 2 as estimated from the model TE model :
Where a *, b *, and c ′* are model parameters. Of course, when c ′* = 0, then T E S E M = a * × b * .
Although the point estimates of TE 1 and TE 2 are equal for a simple mediation model, neither their associated models nor their sampling distributions are. For example, it is well known that the sampling distribution of the indirect effect estimate is skewed unless the sample size is extremely large ( MacKinnon et al., 2004 ) and this also applies when estimated from a global model (the product of a * and b *). The skewness is inherent to the distribution of a product, and this transfers to the distribution of TE 2 whether estimated based on a global model or through separate regressions. In contrast, there is no reason to expect skewness in the sampling distribution of TE 1 because it is a simple parameter in Equation (2) and Figure 1 , and not a product of two parameters.
The study of mediation is almost entirely effect-focused because the substantive hypotheses are mostly about particular mediation effects and their presence or not (typically defined by statistical significance), and so a global model test makes less sense from that perspective. This is particularly true because perfect model fit for the covariance of the variables is guaranteed in a simple mediation model with just the three variables X, M , and Y , despite a simple mediation model being almost certainly incomplete ( Baron and Kenny, 1986 ; Sobel, 2008 ). If one is primarily interested in the effects, it further makes sense to be liberal on the model side because model constraints can lead to bias in the parameter estimates (e.g., forcing a genuine DE to be equal to 0 will bias the IE estimate) and the standard errors.
In contrast, one can expect a model testing approach to prevail in a global process theory that describes the set of variable relationships as a whole. In such a case an SEM makes more sense, and within the model one or more indirect effects are tested (e.g., van Harmelen et al., 2016 ). The time series example is another case where a global model approach makes sense. From an effects perspective the mediation effect for a series of 100 would be a product of 99 parameters and the direct effect would span 99 time intervals, but these would be of relatively little interest or importance. Instead it is the model that matters, and within the model the autoregressive parameter is of interest (and not the IE as a product of all these autoregressive parameters as we did for the simulation study). In a simple autoregressive model with lag 1, i.e., AR ( 1 ), a = b (and so on, depending on the number of time points), and c ′ = 0. The AR(1) autoregressive model characterizes the relevant system, e.g., mood, self-esteem, etc.
As before, the two perspectives are both meaningful. One can either be interested in a global model for the relationships or one can give priority to the effects and minimize the importance of the overall model. The fewer modeling assumptions associated with an effects-perspective may lead to poorer precision and replication (e.g., larger standard errors and greater risk of overfitting), but model-based constraints are avoided. Conversely, making more assumptions leads to better precision and possibly to better replication (if the model constraints are valid). One can also make the statistical model more in line with the theoretical model in order to impose a stronger test of a theory. However, the assumptions are made at the risk of distorted parameter estimates, and the effect estimates are also conditional on the global model they belong to, which can complicate interpretation somewhat. Therefore, it can make sense to stay with separate regression analyses without a test of the global model.
Effect Size vs. Null Hypothesis Testing
Based on criticism of NHST (e.g., Kline, 2004 ), effect size and confidence intervals have been proposed as an alternative approach to statistical analyses (e.g., Cumming, 2012 ). These points have emerged in the mediation literature as well, with mediation-specific effect sizes discussed and proposed (e.g., Kraemer et al., 2008 ; Preacher and Kelley, 2011 ), and bootstrapped confidence intervals are now the standard for testing indirect effects (e.g., Shrout and Bolger, 2002 ; Hayes, 2013 ; Hayes and Scharkow, 2013 ).
Numerous effect size indices have been proposed for the IE , and these indices may take the form of either variance in the DV explained or in terms of the relative effects as in the case of the ratio ab/c ′ (an excellent review may be found in Preacher and Kelley, 2011 ; note however the specific effect size proposed by these authors was later shown to be based on incorrect calculations; Wen and Fan, 2015 ). As it is not our intention to promote one particular measure, but rather to make a general point regarding effect size vs. null hypothesis testing perspectives, we simply use the product of the standardized a and b coefficients.
In the largest time series model illustrated previously, the indirect effect is a product of 99 terms, and as a result the expected effect size with an autoregressive coefficient of 0.90 is still a negligible 0.00003. Even so, this extremely small effect can easily lead to a rejection of the null hypothesis when the IE is tested, as illustrated in Table 1 . The confidence intervals are very narrow for such a small effect, but they do not include zero. In practice, such an example would represent mediation from the NHST perspective (supported by the confidence intervals) and it could potentially be a very meaningful finding, but from the effect size perspective the effect may seem too small to be accepted or worth consideration for any practical decisions. Both points of view make sense. There is clearly mediation in the time series example, but the resulting effect is negligible in terms of the variance explained at time 100. The distance between X and Y is too large for a difference in X to make a difference for Y while in fact the underlying process is clearly a mediation process with possibly a very large magnitude from time point to time point (i.e., as small as 0.9).
As before, neither perspective is strictly superior because both perspectives have advantages and disadvantages. One possible problem when approaching mediation from the NHST perspective is that it is perhaps too attractive to look for possible mediators between X and Y after failing to reject the initial null hypothesis because of the work showing that a test of the IE has higher power, in particular given the high rates at which the TE is not rejected but the IE is as shown in Table 1 (to be clear, a strict NHST perspective would not permit such an approach, as discussed previously). Other problems are the dichotomous view on mediation (mediation vs. no mediation) while effects are in fact graded ( Cumming, 2012 ), and the fact that rejection of the null hypothesis does not speak to how well the variance of Y is explained.
The effect size logic has its own drawbacks as well, of course. Competing indirect effects, regardless of size, can cancel each other out (note this holds true for all effects in a mediation model, e.g., a may be small because of competing effects from X to M ). Another issue is that the effect size is commonly expressed in a relative way (e.g., in terms of the standard deviation of the DV or a percentage explained variance) and therefore it depends on the variance in the sample and on other factors in the study that raise questions about the appropriateness of many mediation effect sizes ( Preacher and Kelley, 2011 ). What constitutes a relevant effect size is also not always immediately clear, as it depends immensely on the problem at hand, e.g., what the dependent variable is, how easily manipulated the independent variable(s) are, etc. A further complicating factor is that most psychological variables have arbitrary units, such as, units on a point-scale or response option numerical anchors for a questionnaire. For variables with natural units, such as, the number of deadly accidents on the road or years of life after a medical intervention, one would not need a standard deviation or a percentage of variance to express the effect size in a meaningful way.
As with the previous perspectives, these two perspectives throw light on two relevant but different aspects of the same underlying reality. The null hypothesis test is a test of a hypothesized process and whether it can be differentiated from noise, whereas the effect size and confidence intervals tell us how large the result of the process is and what the width of the uncertainty is. Not all processes have results of a substantial size—and this is clear in the time-series example we showed previously—but even an extremely small effect can be meaningful as the indication of a process.
Directness vs. Indirectness
Another pair of perspectives depends upon the semantics of causality. In both linguistics (e.g., Shibatani, 2001 ) and in law (e.g., Hart and Honore, 1985 ), directness is an enhancer of causal interpretation, and a remote cause is considered less of a cause or even no cause at all. In contrast, in the psychological literature a causal interpretation is supported when there is evidence for an intermediate psychological or biological process and thus for some indirectness. Causality claims seem supported if one can specify through which path the causality flows.
From the directness perspective, a general concern is that temporal distance allows for additional, unconsidered (e.g., unmodeled) effects to occur, and so the TE is emphasized. Regardless of the complexity of a model, a model is always just a model and by definition it does not capture all aspects of the variable relationships ( Edwards, 2013 ). In reality there are always intervening events such that with increasing time between measurements the chances are higher that unknown events are the proper causes of the dependent variable, rather than the mediator(s). Though a full discussion is too complex to engage in here, a similar view has been taken by philosophers such as, Woodwarth (2003) . The inclusion of a mediator necessarily increases the minimum distance between X and Y , and the associated paths are necessarily correlational and require additional model assumptions, and if these assumptions do not hold then the estimates of the IE and DE are biased ( Sobel, 2008 ). Additionally, one can manipulate X but not M at the same time without likely interfering with the proposed mediation process and thus potentially destroying it, and so the link between M and Y remains a correlational one.
Network models are an interesting example of an indirectness perspective on causation, and one that is taken to a relative extreme. In such models, a large number of variables cause one another, and possibly mutually so, e.g., insomnia may result in concentration difficulties and then work problems, which may then aggravate the insomnia due to excess worry, before ultimately resulting in a depressed state ( Borsboom and Cramer, 2013 ). Another example of an indirectness perspective can be found in relation to climate change: Lakoff (2012) posted an interesting discussion and introduced the term “systemic causation” for causation in a network with chains of indirect causation. Many mediation models one can find in the psychological literature would qualify for the label of systemic causation, both in terms of the model (e.g., multiple connected mediators) and in terms of the underlying processes (e.g., changes in neurotransmitters underlying changes in behavior). Somewhat akin to the effect vs. model testing perspectives, if the additional statistical and theoretical assumptions hold then the benefit is a fuller and more precise picture of the variable relationships, but if they do not then statistical analyses will yield biased estimates and the inferences drawn made suspect.
The two perspectives make sense for the example application from the simulation study. From the directness perspective, as the number of time points increases it becomes increasingly difficult to claim that X has a causal effect on Y . It is easy to make such claims for T = 3, but for a large number of time points such as, T = 50 or 100, claims of causation are most relevant to the mediators most proximal to Y (alternatively, to those shortly following X ). In contrast, for the indirectness perspective, a systems interpretation of causality makes perfect sense for time series. The autoregressive process does have causal relevance, and the identification of such a long chain of effects would likely be considered compelling evidence of causation.
Thus, indirectness and distance make a causal interpretation stronger from one perspective, whereas they make a causal interpretation less convincing from another perspective. These two perspectives are not in direct contradiction—they simply focus on different aspects of the same reality and reflect different needs and concerns. In the case of directness, the criterion is a minimizing ambiguity about whether or not there is an effect of X on Y . In contrast, in the case of adopting an indirectness perspective, the primary criterion is maximizing information about the process and thus about intermediate steps because it makes the causal process more understandable.
Hypothesized vs. Alternative Explanations
Our final pair of perspectives refers to whether one is primarily interested in a confirmatory test of a mediation hypothesis about the relationship between two variables or whether one would rather test one or more other explanations that would undermine a mediation claim. Loosely, the difference between these two perspectives is that the former focuses on showing that a mediation explanation is appropriate, and the latter focuses on showing that alternative explanations are not.
In practice this distinction can be a subtle one, as it is always necessary to control for confounders, but there are considerable differences in the information acquired and required for these two perspectives, as well as the amount of effort invested and what is attended to Rouder et al. (2016) .
For mediation, researchers generally work with a theory-derived mediation hypothesis and collect data that allows them to test the null hypothesis of no mediation. It is a search for a well-defined form of information, and further the search is considered complete when that information is obtained. If the null hypothesis of no relationship is rejected, the mediation claim is considered to be supported and the case closed. If it is not rejected, explanations are generated as to why the study failed, and the hypothesis is tested again (ideally in a separate study, but this also manifests as including unplanned covariates in the statistical models). Alternative explanations are often not generated or tested if the null hypothesis of mediation is rejected. This is an intriguing asymmetry between the two possible outcomes of a study—supportive results are accepted, unsupportive results are retested.
A somewhat different approach is to formulate alternative explanations for a significant effect that are in conflict with a mediation claim. The simplest and most common means of doing this is to include additional covariates in Models 2 and 3 that are competing explanations for the relationships between the three variables, or to experimentally manipulate these explanations as well. In cases where temporal precedence is not clear such as, in observational data or when there are only two time points, it is also useful to consider alternative variable orders, e.g., treating X as M or M as Y . Another approach is to assume that there are unmeasured confounders that bias the estimates and necessitate examining parameter sensitivity ( VanderWeele, 2010 ). Still another is to test the proposed mediator as a moderator instead (a distinction which is itself often unclear; Kraemer et al., 2008 ) or as a hierarchical effect ( Preacher et al., 2010 ).
Referring to the time series example, it was simply a test of an autoregressive model with a single lag and the power to detect such small effects in a constrained serial mediation model, but in practice it would also make sense to consider a moving-average model, where the value of an observation depends on the mean of the variable and on a coefficient associated with the error term ( Brockwell and Davis, 2013 ). Loosely, the residuals might “cause” the values of subsequent time points, and are not simply measurement errors but new and unrelated inputs specific for the time point in question.
As with each previous pair of perspectives, both perspectives have advantages and disadvantages. Focusing on confirmation has the general advantages of simplicity and expediency by utilizing past research to direct future research, with a relatively clearly defined set of criteria for what counts as supporting evidence. There are also cases where it is not necessary to exhaust all alternatives, and instead simplicity and sufficiency of an explanation are valued more strongly. However, this perspective comes with the risk of increased false-positives and a narrow search for explanations for relationships between variables because what is considered is determined in part by what is easy to consider. Finding that one explanation works does not prove there are no other—and possibly better—explanations, and a model is always just a model ( Edwards, 2013 ).
Focusing on competing hypotheses has the advantage of potentially providing stronger evidence for a mediation claim by way of providing evidence that competing hypotheses are not appropriate. Conversely, when a competing hypothesis cannot be ruled out easily, it may turn out to be a better explanation than a mediation model upon further research. However, there are a few very strong limitations regarding competing evidence. The first is that for every explanation, there are an infinite number of competing explanations that are all equally capable of describing a covariance matrix. Some are ignorable due to their sheer absurdity, but there are still an infinite number of reasonable alternative explanations (for example, it is easy to generate a very long list of explanations for why self-esteem and happiness correlate) and criteria for evaluating these explanations are often unclear or extremely difficult to satisfy. Further, it is often impossible to estimate alternative statistical models because of the limited information provided by only a small set of variables (e.g., factors are difficult to estimate with a small number of indicators). Similarly, estimating a very large number of complicated interacting variable relationships may require sample sizes that are not realistic.
A Note Regarding Philosophical Considerations
Before turning to our discussion, we wish to note that philosophical views on causality differ with respect to whether a total effect is implied or necessary, and that there is substantial overlap between the philosophical views and our discussion of directness vs. indirectness distinction. We rely on a chapter by Psillos (2009) in the Oxford Handbook of Causality for a brief discussion of philosophical views, but see White (1990) for an introduction for psychologists.
In Humean regularity theories, X is a cause if it is regularly followed by Y . This suggests a total effect as a condition for X being a cause of Y . In a deductive-nomological view attributed to Hempel and Oppenheim, for X to be a cause it needs to be connected to Y through one or more laws so that X is sufficient for Y . Sufficiency would again imply a total effect, albeit possibly a very small one, because there may be multiple sufficient conditions. Only when a condition is at the same time sufficient and necessary can one expect a clear relationship.
Another view is formulated in the complex regularity view of Mackie (1974) and his INUS conditions. According to this view a cause is an I nsufficient but N on-redundant part of a condition which is itself U nnecessary but S ufficient for the effect. In other words, a cause is a term (e.g., A ) in a conjunctive bundle (e.g., A and B and C ), and there can be many such conjunctive bundles that are each sufficient for the effect. This expression is called the disjunctive normal form (e.g., Y if and only if A and B and C or D and E or F and G or H or I ). This form does not imply a total effect of X on Y (e.g., A as X ), because the disjunctive normal form may be highly complex and may therefore not lead to X and Y being correlated, while X is still accepted as a cause because it is part of that form. In other words, the relationship between a cause and the event to be explained is such that a cause can occur either with or without the event and vice versa. The INUS view is consistent with indirectness and systemic causation, whereas Humean regularity theory is better in agreement with directness of causes.
From the above discussion of the various perspectives we wish to conclude that there is not just one way to look at mediation. Researchers may approach mediation with or without an a priori hypothesis, or may focus on either a global model or a specific effect that derives either from the global model or that is estimated from separate regression analyses. A researcher may value directness or indirectness as causal evidence, or may prefer effect-focused or significance-focused tests. Researchers may further focus on hypothesized or competing alternative explanations when testing for mediation. Each pair of perspectives has associated advantages and disadvantages, and which is to be preferred depends on the nature of a given study or topic of interest.
The perspectives we have discussed here do not exhaust all common perspectives. Another common pair is a practical vs. a theoretical goal for testing a mediation claim. The aim of a mediation study can either be to find ways to change the level of the dependent variable, or the aim can be to understand the process through which the independent variable affects the dependent variable, or the purpose of the research may be prediction. Mediation can help to understand a process and advance a theoretical goal even when the total effect is negligible, but from a practical point of view, mediation is not helpful for such a case unless there is an easily addressed suppression effect or Y represents an important outcome such as, death. For applied settings where affecting change by way of an intervention of some sort, a direct effect or an unsuppressed large indirect effect is in general much more useful.
Another example is that the concept of mediation remains somewhat ambiguous despite the clarification provided by Baron and Kenny (1986) . That mediation explains the relationship between X and Y can mean two things: (1) Mediation explains values of Y as indirectly caused by values of X . (2) Mediation causes the relationship between X and Y . Following the second interpretation, the relationship itself (or absence of relationship) is explained by values of M . Here, we have interpreted the concept of mediation in the first sense. Note that the second way of understanding mediation is also commonly considered to be moderation , where M is supposed to explain why there sometimes is a relationship between X and Y and sometimes there is not (or why the strength of the relationship varies). The MacArthur approach provides some clarification regarding the latter sense (the approach is named after a foundation; Kraemer et al., 2002 , 2008 ), and notably it adds an interaction term between X and M to Model 3. The approach specifies that if X precedes M , there is an association between X and M , and there is either an interaction between X and M or a main effect of M on Y then M is said to mediate Y . In contrast, if there is an interaction between X and M , but no main effect of M on Y , then X is said to moderate M . In short, the approach specifies that a statistical interaction can still reflect mediation (see also Muller et al., 2005 ; Preacher et al., 2007 ). The approach further focuses on effect sizes over NHST, and states that causal inferences should not be drawn from observational data for reasons similar to those we provide in the discussion of the hypothesized vs. alternative explanations section. The approach also explicitly treats the indirect effect as only potentially causal, arguing that the Baron and Kenny approach to mediation and moderation can potentially bias the search for explanations because of its assumption that the causal process is already known but must only be tested. The MacArthur approach then seems to favor (or is at least mindful of) some of the specific perspectives we have discussed here, and it remains to be seen what the impact is of the approach on mediation and moderation practice and theory.
We have discussed mediation at a rather abstract, general level, and some of the details of the different perspectives we have discussed here are not always relevant to specific statistical analyses. In keeping with common practices we have utilized parametric mean and covariance-based approaches for our discussion, but median-based approaches to mediation have been proposed (e.g., Yuan and MacKinnon, 2014 ), and for such approaches the notion of global model testing by way of comparing the fit of different SEMs is largely irrelevant in a frequentist framework (though it may be done within a Bayesian framework; Wang et al., 2016 ). For network analysis, the strong focus on indirectness of effects within a larger system with a very large number of variables that each may be treated as X, M , or Y , renders the issue of a specific mediation hypothesis or a total effect irrelevant.
On the other hand, while we have discussed each perspective as independent views, there are obvious intersections between them and ample reasons to adopt the opposing perspective in some cases, or even both for the same study. For example, when working with a global model, specific effects within the model vary in how trustworthy they may be considered. Those effects that are considered less trustworthy can be interpreted more from a directness perspective because of the ambiguity regarding their effects, and those that are uncontroversial can be interpreted from an indirectness perspective. Confidence intervals and NHST also make use of the same information and if interpreted dichotomously (reject vs. not reject) the results will not differ. There are also intersections across pairs as well, e.g., testing competing explanations is facilitated by adopting a global model-focused approach, and the issue competing explanations in general provides much of the rationale for preferring a directness perspective on causation.
We wish to include a cautionary note concerning causality before concluding. A mediation hypothesis is a causal hypothesis ( James and Brett, 1984 ), but we realize that a causal relationship is difficult if not impossible to prove in general, let alone in the complex world of the social sciences ( Brady, 2008 ). Further, the statistical models used to test mediation are not inherently causal—they are simply predictive or descriptive, and the b path is necessarily correlational ( Sobel, 2008 ). That the data are in line with the hypothesis and even that several alternative explanations can be eliminated does not prove causality. It does not follow from the combination of the two premises “If A then B” (if M mediates then the null hypothesis of no indirect effect is rejected) and “B is the case” (null hypothesis rejected) that “A is the case.” (M mediates; i.e., the fallacy known as affirming the consequent). Instead, modus tollens (i.e., “B is not the case”) is a valid argument for the absence of A, so that one may want to believe that A is ruled out in the absence of B. Although the reasoning is logically correct, the problem with mediation analysis is that “B is not the case” in practice is simply a probabilistic non-rejection of a null hypothesis and does not directly implicate the truth of any other claim.
Human behavior and psychology emerges from dynamic and complicated systemic effects that are impossible to capture completely, and researchers choose what must be understood for a given problem—what fraction of the network of interacting variables is most relevant—and so which perspective to adopt. Ultimately, mediation analysis is simply a tool used for describing, discovering, and testing possible causal relationships. How the tool is used (or not used) and what information is most relevant depends on the problem to be solved and the question to be answered.
Author Contributions
RA was responsible for most of the writing, in particular any revisions and the introduction and discussion. PD provided most of the core points involved in the discussion of each perspective.
Conflict of Interest Statement
The authors declare that the research was conducted in the absence of any commercial or financial relationships that could be construed as a potential conflict of interest.
Baron, R. M., and Kenny, D. A. (1986). The moderator-mediator variable distinction in social psychological research: conceptual, strategic, and statistical considerations. J. Pers. Soc. Psychol. 51, 1175–1182. doi: 10.1037/0022-3514.51.6.1173
PubMed Abstract | CrossRef Full Text | Google Scholar
Borsboom, D., and Cramer, A. O. (2013). Network analysis: an integrative approach to the structure of psychopathology. Annu. Rev. Clin. Psychol. 9, 91–121. doi: 10.1146/annurev-clinpsy-050212-185608
Brady, H. E. (2008). Causation and Explanation in Social Science . Oxford, UK: Oxford University Press.
Google Scholar
Brockwell, P. J., and Davis, R. A. (2013). Time Series: Theory and Methods . New York, NY: Springer-Verlag.
Bullock, J. G., Green, D. P., and Ha, S. E. (2010). Yes, but what's the mechanism? (don't expect an easy answer). J. Pers. Soc. Psychol. 98, 550–558. doi: 10.1037/a0018933
Cumming, G. (2012). Understanding the New Statistics: Effect Sizes, Confidence Intervals, and Meta-Analysis . New York, NY: Routledge.
Edwards, M. C. (2013). Purple unicorns, true models, and other things i've never seen. Meas. Interdiscipl. Res. Perspect. 11, 107–111. doi: 10.1080/15366367.2013.835178
CrossRef Full Text | Google Scholar
Fritz, M. S., Kenny, D. A., and MacKinnon, D. P. (2016). The combined effects of measurement error and omitting confounders in the single mediator model. Multivariate Behav. Res. 51, 681–697. doi: 10.1080/00273171.2016.1224154
Fritz, M. S., and MacKinnon, D. P. (2007). Required sample size to detect the mediated effect. Psychol. Sci. 18, 233–239. doi: 10.1111/j.1467-9280.2007.01882.x
Gelman, A., and Hennig, C. (2017). Beyond subjective and objective in statistics. J. R. Stat. Soc . 180, 1–31. doi: 10.1111/rssa.12276
Hart, H. L. A., and Honore, A. M. (1985). Causation in the Law . Oxford, UK: Clarendon Press.
Hayes, A. F. (2013). Introduction to Mediation, Moderation, and Conditional Process Analysis: A Regression-Based Approach . New York, NY: Guilford.
Hayes, A. F., and Scharkow, M. (2013). The relative trustworthiness of inferential tests of the indirect effect in statistical mediation analysis: does method really matter? Psychol. Sci. 24, 1918–1927. doi: 10.1177/0956797613480187
Iacobucci, D., Saldanha, N., and Deng, X. (2007). A meditation on mediation: evidence that structural equation models perform better than regressions. J. Consum. Psychol. 17, 140–154. doi: 10.1016/S1057-7408(07)70020-7
Imai, K., Keele, L., and Yamamoto, T. (2010). Identification, inference and sensitivity analysis for causal mediation effects. Stat. Sci. 25, 51–71. doi: 10.1214/10-STS321
James, L. R., and Brett, J. M. (1984). Mediators, moderators, and tests for mediation. J. Appl. Psychol. 69, 307–321. doi: 10.1037/0021-9010.69.2.307
Jo, B. (2008). Causal inference in randomized experiments with mediational processes. Psychol. Methods 13, 314–336. doi: 10.1037/a0014207
Judd, C. M., and Kenny, D. A. (1981). Process analysis: estimating mediation in treatment evaluation. Eval. Rev. 5, 602–619. doi: 10.1177/0193841X8100500502
Kenny, D. A., and Judd, C. M. (2014). Power anomalies in testing mediation. Psychol. Sci. 25, 334–339. doi: 10.1177/0956797613502676
Kerr, N. L. (1998). HARKing: hypothesizing after the results are known. Pers. Soc. Psychol. Rev. 2, 196–217. doi: 10.1207/s15327957pspr0203_4
Kline, R. B. (2004). Beyond Significance Testing. Reforming Data Analysis Methods in Behavioral Research. Washington DC: APA Books.
Kraemer, H. C., Kiernan, M., Essex, M., and Kupfer, D. J. (2008). How and why criteria defining moderators and mediators differ between the Baron & Kenny and MacArthur approaches. Health Psychol. 27, 101–108. doi: 10.1037/0278-6133.27.2(Suppl.).S101
Kraemer, H. C., Wilson, G. T., Fairburn, C. G., and Agras, W. S. (2002). Mediators and moderators of treatment effects in randomized clinical trials. Arch. Gen. Psychiatry 59, 877–883. doi: 10.1001/archpsyc.59.10.877
Lakoff, G. (2012). Global Warming Systemically Caused Hurricane Sandy . Available online at: http://blogs.berkeley.edu/2012/11/05/global-warming-systemically-caused-hurricane-sandy/
Loeys, T., Moerkerke, B., and Vansteelandt, S. (2015). A cautionary note on the power of the test for the indirect effect in mediation analysis. Front. Psychol. 5:1549. doi: 10.3389/fpsyg.2014.01549
Mackie, J. L. (1974). The Cement of the Universe . Oxford, UK: Clarendon Pres.
MacKinnon, D. (2008). Introduction to Statistical Mediation Analysis . New York, NY: Lawrence Erlbaum.
MacKinnon, D. P., Krull, J. L., and Lockwood, C. M. (2000). Equivalence of the mediation, confounding and suppression effect. Prev. Sci. 1, 173–181. doi: 10.1023/A:1026595011371
MacKinnon, D. P., Lockwood, C. M., and Williams, J. (2004). Confidence limits for the indirect effect. Distribution of the product and resampling methods. Multivariate Behav. Res. 39, 99–128. doi: 10.1207/s15327906mbr3901_4
Muller, D., Judd, C. M., and Yzerbyt, V. Y. (2005). When moderation is mediated and mediation is moderated. J. Pers. Soc. Psychol. 89:852. doi: 10.1037/0022-3514.89.6.852
O'Rourke, H. P., and MacKinnon, D. P. (2015). When the test of mediation is more powerful than the test of the total effect. Behav. Res. Methods , 47:424. doi: 10.3758/s13428-014-0481-z
Pearl, J. (2009). Causality . Cambridge University Press.
PubMed Abstract | Google Scholar
Preacher, K. J., and Hayes, A. F. (2008). Asymptotic and resampling strategies for assessing and comparing indirect effects in multiple mediator models. Behav. Res. Methods 40, 879–891. doi: 10.3758/BRM.40.3.879
Preacher, K. J., and Kelley, K. (2011). Effect measures for mediation models. Quantitative strategies for communication indirect effects. Psychol. Methods 16, 93–115. doi: 10.1037/a0022658
Preacher, K. J., Rucker, D. D., and Hayes, A. F. (2007). Addressing moderated mediation hypotheses: theory, methods, and prescriptions. Multivariate Behav. Res. 42, 185–227. doi: 10.1080/00273170701341316
Preacher, K. J., Zyphur, M. J., and Zhang, Z. (2010). A general multilevel SEM framework for assessing multilevel mediation. Psychol. Methods 15, 209–233. doi: 10.1037/a0020141
Psillos, S. (2009). “Regularity theories,” in Oxford Handbook of Causation , eds H. Bebee, P. Menzies, and C. Hitchcock (New York, NY: Oxford University Press), 131–157.
Rijnhart, J. J., Twisk, J. W., Chinapaw, M. J., de Boer, M. R., and Heymans, M. W. (2017). Comparison of methods for the analysis of relatively simple mediation models. Contemp. Clin. Trials Commun . 7, 130–135. doi: 10.1016/j.conctc.2017.06.005
Rouder, J. N., Morey, R. D., Verhagen, J., Province, J. M., and Wagenmakers, E. J. (2016). Is there a free lunch in inference?. Top. Cogn. Sci. 8, 520–547. doi: 10.1111/tops.12214
Rucker, D. D., Preacher, K. J., Tormala, Z. L., and Petty, R. E. (2011). Mediation analysis in social psychology: current practices and new recommendations. Soc. Pers. Psychol. Compass 5, 359–371. doi: 10.1111/j.1751-9004.2011.00355.x
Salter-Townshend, M., White, A., Gollini, I., and Murphy, T. B. (2012). Review of statistical network analysis: models, algorithms, and software. Statist. Anal. Data Mining 5, 243–264. doi: 10.1002/sam.11146
Shibatani, M. (2001). “Some basic issues in the grammar of causation,” in Grammar of Causation and Interpersonal Manipulation , ed M. Shibatani (Philadelphia, PA: John Benjamins Publishing Company), 1–22.
Shrout, P. E., and Bolger, N. (2002). Mediation in experimental and nonexperimental studies: new procedures and recommendations. Psychol. Methods 7, 422–455. doi: 10.1037/1082-989X.7.4.422
Sobel, M. E. (2008). Identification of causal parameters in randomized studies with mediating variables. J. Educ. Behav. Stat. 33, 230–251. doi: 10.3102/1076998607307239
van Harmelen, A. L., Gibson, J. L., St Clair, M. C., Owens, M., Brodbeck, J., Dunn, V., et al. (2016). Friendships and family support reduce subsequent depressive symptoms in at-risk adolescents. PLoS ONE 11:e0153715. doi: 10.1371/journal.pone.0153715
VanderWeele, T. J. (2010). Bias formulas for sensitivity analysis for direct and indirect effects. Epidemiology 21, 540–551. doi: 10.1097/EDE.0b013e3181df191c
Wagenmakers, E. J., Wetzels, R., Borsboom, D., and Van Der Maas, H. L. (2011). Why psychologists must change the way they analyze their data: the case of psi: comment on Bem (2011). J. Pers. Soc. Psychol. 100, 426–432. doi: 10.1037/a0022790
Wang, Y., Feng, X. N., and Song, X. Y. (2016). Bayesian quantile structural equation models. Struct. Equ. Model. Multidiscipl. J. 23, 246–258. doi: 10.1080/10705511.2015.1033057
Wen, Z., and Fan, X. (2015). Monotonicity of effect sizes: questioning kappa-squared as mediation effect size measure. Psychol. Methods 20, 193–203. doi: 10.1037/met0000029
White, P. (1990). Ideas about causation in philosophy and psychology. Psychol. Bull. 108, 3–18. doi: 10.1037/0033-2909.108.1.3
Woodwarth, J. (2003). Making Things Happen. A Theory of Causal Explanation . Oxford: Oxford University Press.
Yuan, Y., and MacKinnon, D. P. (2014). Robust mediation analysis based on median regression. Psychol. Methods 19, 1–20. doi: 10.1037/a0033820
Keywords: mediation, causation, total effect, direct effect, indirect effect
Citation: Agler R and De Boeck P (2017) On the Interpretation and Use of Mediation: Multiple Perspectives on Mediation Analysis. Front. Psychol . 8:1984. doi: 10.3389/fpsyg.2017.01984
Received: 06 July 2017; Accepted: 30 October 2017; Published: 15 November 2017.
Reviewed by:
Copyright © 2017 Agler and De Boeck. This is an open-access article distributed under the terms of the Creative Commons Attribution License (CC BY) . The use, distribution or reproduction in other forums is permitted, provided the original author(s) or licensor are credited and that the original publication in this journal is cited, in accordance with accepted academic practice. No use, distribution or reproduction is permitted which does not comply with these terms.
*Correspondence: Robert Agler, [email protected]
Disclaimer: All claims expressed in this article are solely those of the authors and do not necessarily represent those of their affiliated organizations, or those of the publisher, the editors and the reviewers. Any product that may be evaluated in this article or claim that may be made by its manufacturer is not guaranteed or endorsed by the publisher.
- Introduction
- Conclusions
- Article Information
A confounder of the association between an exposure and a mediator or between an exposure and an outcome is a preexposure variable that is associated with the exposure and with the mediator or outcome, respectively. A confounder of the association between a mediator and an outcome is a premediator variable (possibly affected by the exposure) that is associated with the mediator and outcome. Because confounders can distort associations, controlling for confounders of the exposure-mediator, exposure-outcome, and mediator-outcome associations is important in mediation analyses. A collider on a path in the causal directed acyclic graph between 2 variables is a variable that is affected by both variables. Standard adjustment for a collider typically introduces selection bias and special care may be needed when controlling for colliders. Effect modification (interaction) cannot be depicted in a standard directed acyclic graph.
eFigure 1. Flow diagram of AGReMA checklist development process
eFigure 2. Decision tree to guide selection of appropriate version of AGReMA
eTable 1. Contributors to the development of AGReMA
eTable 2. Characteristics of Delphi study and consensus meeting participants
eAppendix 1. Decision rules that were used to guide the Consensus Meeting, and a summary of anonymized meeting notes
eAppendix 2. Decision rules that were used to guide the selection of AGReMA Short Form items and their item level inclusion ratings
- A Reporting Guideline for Mediation Analyses JAMA Editorial September 21, 2021 Kabir Yadav, MDCM, MS, MSHS; Roger J. Lewis, MD, PhD
- Causal Directed Acyclic Graphs JAMA JAMA Guide to Statistics and Methods March 15, 2022 This JAMA Guide to Statistics and Methods discusses the basics of causal directed acyclic graphs, which are useful tools for communicating researchers’ understanding of the potential interplay among variables and are commonly used for mediation analysis. Ari M. Lipsky, MD, PhD; Sander Greenland, MA, MS, DrPH
- Reporting Findings From Mediation Analyses JAMA Editor's Note September 21, 2021 Phil B. Fontanarosa, MD, MBA
See More About
Select your interests.
Customize your JAMA Network experience by selecting one or more topics from the list below.
- Academic Medicine
- Acid Base, Electrolytes, Fluids
- Allergy and Clinical Immunology
- American Indian or Alaska Natives
- Anesthesiology
- Anticoagulation
- Art and Images in Psychiatry
- Artificial Intelligence
- Assisted Reproduction
- Bleeding and Transfusion
- Caring for the Critically Ill Patient
- Challenges in Clinical Electrocardiography
- Climate and Health
- Climate Change
- Clinical Challenge
- Clinical Decision Support
- Clinical Implications of Basic Neuroscience
- Clinical Pharmacy and Pharmacology
- Complementary and Alternative Medicine
- Consensus Statements
- Coronavirus (COVID-19)
- Critical Care Medicine
- Cultural Competency
- Dental Medicine
- Dermatology
- Diabetes and Endocrinology
- Diagnostic Test Interpretation
- Drug Development
- Electronic Health Records
- Emergency Medicine
- End of Life, Hospice, Palliative Care
- Environmental Health
- Equity, Diversity, and Inclusion
- Facial Plastic Surgery
- Gastroenterology and Hepatology
- Genetics and Genomics
- Genomics and Precision Health
- Global Health
- Guide to Statistics and Methods
- Hair Disorders
- Health Care Delivery Models
- Health Care Economics, Insurance, Payment
- Health Care Quality
- Health Care Reform
- Health Care Safety
- Health Care Workforce
- Health Disparities
- Health Inequities
- Health Policy
- Health Systems Science
- History of Medicine
- Hypertension
- Images in Neurology
- Implementation Science
- Infectious Diseases
- Innovations in Health Care Delivery
- JAMA Infographic
- Law and Medicine
- Leading Change
- Less is More
- LGBTQIA Medicine
- Lifestyle Behaviors
- Medical Coding
- Medical Devices and Equipment
- Medical Education
- Medical Education and Training
- Medical Journals and Publishing
- Mobile Health and Telemedicine
- Narrative Medicine
- Neuroscience and Psychiatry
- Notable Notes
- Nutrition, Obesity, Exercise
- Obstetrics and Gynecology
- Occupational Health
- Ophthalmology
- Orthopedics
- Otolaryngology
- Pain Medicine
- Palliative Care
- Pathology and Laboratory Medicine
- Patient Care
- Patient Information
- Performance Improvement
- Performance Measures
- Perioperative Care and Consultation
- Pharmacoeconomics
- Pharmacoepidemiology
- Pharmacogenetics
- Pharmacy and Clinical Pharmacology
- Physical Medicine and Rehabilitation
- Physical Therapy
- Physician Leadership
- Population Health
- Primary Care
- Professional Well-being
- Professionalism
- Psychiatry and Behavioral Health
- Public Health
- Pulmonary Medicine
- Regulatory Agencies
- Reproductive Health
- Research, Methods, Statistics
- Resuscitation
- Rheumatology
- Risk Management
- Scientific Discovery and the Future of Medicine
- Shared Decision Making and Communication
- Sleep Medicine
- Sports Medicine
- Stem Cell Transplantation
- Substance Use and Addiction Medicine
- Surgical Innovation
- Surgical Pearls
- Teachable Moment
- Technology and Finance
- The Art of JAMA
- The Arts and Medicine
- The Rational Clinical Examination
- Tobacco and e-Cigarettes
- Translational Medicine
- Trauma and Injury
- Treatment Adherence
- Ultrasonography
- Users' Guide to the Medical Literature
- Vaccination
- Venous Thromboembolism
- Veterans Health
- Women's Health
- Workflow and Process
- Wound Care, Infection, Healing
Others Also Liked
- Download PDF
- X Facebook More LinkedIn
- CME & MOC
Lee H , Cashin AG , Lamb SE, et al. A Guideline for Reporting Mediation Analyses of Randomized Trials and Observational Studies : The AGReMA Statement . JAMA. 2021;326(11):1045–1056. doi:10.1001/jama.2021.14075
Manage citations:
© 2024
- Permissions
A Guideline for Reporting Mediation Analyses of Randomized Trials and Observational Studies : The AGReMA Statement
- 1 Centre for Statistics in Medicine, Nuffield Department of Orthopaedics, Rheumatology, and Musculoskeletal Sciences, University of Oxford, Oxford, England
- 2 School of Medicine and Public Health, University of Newcastle, Callaghan, Australia
- 3 Prince of Wales Clinical School, Faculty of Medicine, University of New South Wales, Sydney, Australia
- 4 Neuroscience Research Australia, Sydney
- 5 College of Medicine and Health, University of Exeter Medical School, Exeter, England
- 6 Department of Applied Mathematics, Computer Science, and Statistics, Ghent University, Ghent, Belgium
- 7 Department of Medical Statistics, London School of Hygiene and Tropical Medicine, London, England
- 8 Departments of Epidemiology and Biostatistics, T. H. Chan School of Public Health, Harvard University, Boston, Massachusetts
- 9 Department of Psychology, Arizona State University, Phoenix
- 10 College of Health and Life Sciences, Aston University, Birmingham, England
- 11 NIHR Oxford Biomedical Research Centre, Oxford University Hospitals NHS Foundation Trust, Oxford, England
- 12 JAMA Editorial Office, Chicago, Illinois
- 13 Division of General Internal Medicine and Geriatrics, Department of Preventive Medicine, Feinberg School of Medicine, Northwestern University, Chicago, Illinois
- 14 School of Health Sciences, Faculty of Medicine, University of New South Wales, Sydney, Australia
- Editorial A Reporting Guideline for Mediation Analyses Kabir Yadav, MDCM, MS, MSHS; Roger J. Lewis, MD, PhD JAMA
- Editor's Note Reporting Findings From Mediation Analyses Phil B. Fontanarosa, MD, MBA JAMA
- JAMA Guide to Statistics and Methods Causal Directed Acyclic Graphs Ari M. Lipsky, MD, PhD; Sander Greenland, MA, MS, DrPH JAMA
Question What information should be reported in studies that include mediation analyses of randomized trials and observational studies?
Findings An international Delphi and consensus process (using the Enhancing Quality and Transparency of Health Research methodological framework) generated a 25-item reporting guideline for primary reports of mediation analyses and a 9-item short form for secondary reports of mediation analyses.
Meaning Using the 25-item or 9-item reporting guideline may facilitate peer review and could help ensure that studies using mediation analyses are completely, accurately, and transparently reported.
Importance Mediation analyses of randomized trials and observational studies can generate evidence about the mechanisms by which interventions and exposures may influence health outcomes. Publications of mediation analyses are increasing, but the quality of their reporting is suboptimal.
Objective To develop international, consensus-based guidance for the reporting of mediation analyses of randomized trials and observational studies (A Guideline for Reporting Mediation Analyses; AGReMA).
Design, Setting, and Participants The AGReMA statement was developed using the Enhancing Quality and Transparency of Health Research (EQUATOR) methodological framework for developing reporting guidelines. The guideline development process included (1) an overview of systematic reviews to assess the need for a reporting guideline; (2) review of systematic reviews of relevant evidence on reporting mediation analyses; (3) conducting a Delphi survey with panel members that included methodologists, statisticians, clinical trialists, epidemiologists, psychologists, applied clinical researchers, clinicians, implementation scientists, evidence synthesis experts, representatives from the EQUATOR Network, and journal editors (n = 19; June-November 2019); (4) having a consensus meeting (n = 15; April 28-29, 2020); and (5) conducting a 4-week external review and pilot test that included methodologists and potential users of AGReMA (n = 21; November 2020).
Results A previously reported overview of 54 systematic reviews of mediation studies demonstrated the need for a reporting guideline. Thirty-three potential reporting items were identified from 3 systematic reviews of mediation studies. Over 3 rounds, the Delphi panelists ranked the importance of these items, provided 60 qualitative comments for item refinement and prioritization, and suggested new items for consideration. All items were reviewed during a 2-day consensus meeting and participants agreed on a 25-item AGReMA statement for studies in which mediation analyses are the primary focus and a 9-item short-form AGReMA statement for studies in which mediation analyses are a secondary focus. These checklists were externally reviewed and pilot tested by 21 expert methodologists and potential users, which led to minor adjustments and consolidation of the checklists.
Conclusions and Relevance The AGReMA statement provides recommendations for reporting primary and secondary mediation analyses of randomized trials and observational studies. Improved reporting of studies that use mediation analyses could facilitate peer review and help produce publications that are complete, accurate, transparent, and reproducible.
Quiz Ref ID Health interventions and exposures often work through biological, psychological, and social mechanisms. These mechanisms can be quantitatively evaluated using mediation analyses (an analytic method commonly used in medicine, epidemiology, psychology, and the social sciences). 1 , 2 The principal aim of mediation analyses is to estimate the extent to which an intervention or exposure may affect an outcome through a potential causal mechanism. The findings from mediation analyses can advance theory, inform policy, optimize interventions, and facilitate the implementation of policies and interventions to clinical and public health practice. The value of mediation analyses of randomized trials and observational studies has been recognized by national funding organizations such as the US National Institutes of Health and the UK National Institute for Health Research. 3 , 4 Most mediation analyses are reported within the primary publication of a randomized trial or observational study, or as a separate report with reference to the primary publication. Even though the number of such publications has increased since 2014, 5 recent reviews have shown that reporting is varied and often incomplete. 6 , 7
The aim of this initiative was to develop an evidence- and consensus-based reporting guideline for studies reporting mediation analyses (A Guideline for Reporting Mediation Analyses; AGReMA). The AGReMA project aimed to produce a long and short form to support primary or secondary reports of mediation analyses. This Special Communication describes the methods that were used to develop the guideline, provides long- and short-form checklists to be used when writing research reports, presents brief explanations for each reporting item, and provides guidance on how to use AGReMA.
A glossary of terms used in this article and in the long- and short-form checklists appears in the Box . Terms such as direct effect , indirect effect , and path-specific effects are conventional terminology for mediation analyses because the purpose of these analyses is to test hypotheses about potential causal effects. However, caution is warranted in interpreting estimated effects as causal inferences because causal assumptions (ie, there was sufficient control for mediator-outcome confounding) may be unmet, even in the context of a randomized trial of a treatment.
Glossary of Conventional Terms Used in Mediation Analyses
Action theory: A theory that supports the hypothesized relationship between an intervention or an exposure and a given mediator.
Collider: In the context of mediation analyses, a collider is a variable that is caused by the intervention or exposure and mediator, by the intervention or exposure and outcome, or by the mediator and outcome. Conditioning on a collider by design or analysis may induce selection bias.
Conceptual theory: A theory that supports the hypothesized relationship between a mediator and a given outcome.
Confounder: In the context of mediation analyses, a confounder is a variable that causes the intervention or exposure and mediator, the intervention or exposure and outcome, or the mediator and outcome. Uncontrolled confounders can induce confounding bias.
Consensus panel: A group of experts representing relevant methodologists, statisticians, clinical trialists, epidemiologists, psychologists, clinical researchers, clinicians, implementation scientists, evidence synthesis experts, representatives from the Enhancing Quality and Transparency of Health Research Network, and journal editors.
Controlled direct effect: The exposure’s effect on the outcome if a given mediator were fixed at a constant level uniformly across the entire study population.
Causal directed acyclic graph: A graphic approach for representing causal relationships between variables and a method for identifying confounding variables that should be adjusted when estimating causal effects (see Figure).
Disjunctive cause criterion: A criterion that recommends adjusting for all covariates that are causes of the exposure, outcome, or both when the underlying causal structure is unknown and only limited knowledge is available.
Mechanism: The causal process by which an exposure causes an outcome.
Mediation analysis: An empirical method used to explain how an exposure causes an outcome.
Mediator: A variable that may be affected by an exposure and may in turn affect an outcome.
Moderator: A variable that alters the direction or magnitude of the effect of an exposure on an outcome.
Natural direct effect: The exposure’s effect on the outcome if a given mediator were fixed at its natural value (defined as the value it would take under a given fixed level of the exposure).
Natural indirect effect: An effect on the outcome that is caused by the exposure’s effect on a given mediator and that mediator’s subsequent effect on the outcome.
Path-specific effect: An effect that captures how much of the exposure’s effect on a given outcome is mediated through intermediate variables along 1 or multiple pathways.
Spillover effect: When the outcome of a participant in a study is affected by the intervention status of other participants in the same study.
Total effect: The entire effect of the exposure on the outcome that encompasses all indirect and direct effects.
Unmeasured confounder: An unmeasured variable that is associated with the exposure, mediator, or outcome.
The AGReMA initiative followed the Enhancing Quality and Transparency of Health Research (EQUATOR) methodological framework for the development of reporting guidelines, 8 which included: (1) review of systematic reviews of reporting practices; (2) conducting a Delphi survey; (3) having a consensus meeting with methodologists, statisticians, clinical trialists, epidemiologists, psychologists, clinical researchers, clinicians, implementation scientists, evidence synthesis experts, representatives from the EQUATOR Network, and journal editors (n = 19; June-November 2019); and (4) conducting an external review and pilot test. This section provides a summary of the methods (a flow diagram of the checklist development process appears in eFigure 1 in the Supplement ). Additional details can be found in the protocol. 9 The University of New South Wales human research ethics advisory panel provided ethical approval (HC16599). All participants provided electronic informed consent prior to commencing the first Delphi round.
Quiz Ref ID A previously reported overview of 54 systematic reviews of studies that used mediation analyses found that incomplete reporting impeded interpretation, quality appraisal, reproducibility, and meta-analytic synthesis. 6 These findings were supported by other systematic reviews of mediation analyses of randomized trials 7 and observational studies, 10 , 11 and thus demonstrated the need for a reporting guideline. With assistance from a medical librarian, we conducted a separate scoping search of MEDLINE, PsycINFO, and PubMed (each database searched from inception to March 2019) to identify textbooks and reports that provide guidance on the reporting of mediation studies. We also searched the reference lists of included articles for relevant reports. These reviews, textbooks, and reports were used to identify poorly reported items that were summarized and categorized into themes to be considered by the Delphi panel.
Forty international experts in developing methodological frameworks for mediation analyses or in developing application of mediation analyses for clinical research were invited to participate in a Delphi survey. Nineteen experts agreed to participate and contributed to all 3 Delphi rounds (eTables 1-2 in the Supplement ). The Delphi panelists were asked (1) to rate the importance of a list of items generated from the previous systematic reviews, textbooks, reports, and existing reporting guidance for inclusion in AGReMA; (2) to contribute additional items when possible; and (3) to provide suggestions for item refinement. The panel reached consensus on 34 reporting items for study design, analytic procedures, and effect estimates; 3 items were rated as “optional”; and 60 qualitative comments were provided for item refinement and prioritization. 12 The detailed methods and results of the Delphi study have been reported. 13
A face-to-face consensus meeting was organized to consolidate the final list of reporting items. Due to international travel restrictions imposed by the COVID-19 pandemic, the planned face-to-face meeting was replaced with an online meeting held over 2 days (April 28-29, 2020). A purposeful sample of 15 key experts in methodological development, application of mediation analyses, or reporting of guideline development participated in the meeting (eTables 1-2 in the Supplement ). All items from the Delphi survey were reviewed alongside newly suggested items from the consensus panel. The decision rules that were used to guide the consensus meeting and a summary of the anonymized meeting notes appear in eAppendix 1 in the Supplement .
Mediation analyses are often secondary analyses (eg, after primary analysis of a randomized clinical trial) and may be reported within the primary article or as stand-alone reports. To reflect this distinction, we created 25-item (long form) and 9-item (short form) checklists. The long form is intended for reports that primarily focus on the results of mediation analyses, and the short form is intended for reports that primary focus on the principal findings of a randomized trial or observational study along with a short section for mediation analyses. The consensus group rated the importance of each AGReMA item for inclusion in the 9-item short form using a 10-point Likert scale (0 = not important; 9 = critically important), and participants were invited to provide comments as free text.
We calculated the median scores for each item and plotted the distribution of the ratings using histograms. We included items that had a median score greater than 7 and excluded items with a median score of 7 or less. Detailed results appear in eAppendix 2 in the Supplement . This process was not prespecified in the protocol because the idea of creating a short-form checklist was introduced during the development process.
After reaching consensus, draft versions of the long- and short-form checklists were circulated to all members for comments and edits. The checklists were then pilot tested in November 2020 among peers of the internal steering committee and externally reviewed by 21 expert methodologists and potential users of AGReMA for clarification and specific checklist item wording. During the pilot testing, we asked participants to use the checklists and to provide general feedback on accessibility and usability, and to identify possible reporting items that might have been overlooked. We also asked for specific feedback about the utility and understandability of each item. The characteristics of the participants for the external review and pilot testing appear in eTable 1 and eTable 2 in the Supplement . After this process, all AGReMA members approved and agreed on the final AGReMA statement.
The international consensus process produced a 25-item AGReMA checklist statement and a 9-item AGReMA short-form (AGReMA-SF). The AGReMA-SF is a subset of items from the standard checklist that were considered essential for reporting mediation analyses within reports of randomized trials or observational studies. A decision tree to help users select the appropriate checklist version of AGReMA appears in eFigure 2 in the Supplement .
All items of the AGReMA checklist statement appear in Table 1 . The following section provides brief explanations for each AGReMA item and, when possible, evidence that supports the inclusion of each item is referenced. When evidence was not available, the inclusion of the item was supported by the expert consensus panel. The items that are included in the AGReMA-SF checklist appear in Table 2 and are marked with an asterisk (objectives, effects of interest, causal assumptions, measurement, statistical methods, participants, outcomes and estimates, limitations, and interpretation). Excerpts of exemplar reporting will be provided on a public website ( https://agrema-statement.org ) as reporting standards improve.
Identify that the study uses mediation analyses.
Readers should be able to identify from the title that the study used mediation analyses. Including terms such as mediation analysis (Medical Subject Headings term), mediation , or mediator in the title or as keywords can ensure that mediation studies will be appropriately indexed and identified in literature searches.
Provide a structured summary of the objectives, methods, results, and conclusions specific to mediation analyses.
It is recommended that authors describe (at minimum) the study objectives (ideally supported by a brief statement of background and rationale for the mechanisms of interest), methods (ideally including the setting, participants, sample size, exposure, mediator, outcome, and analytic approach for mediation analyses), results (including point estimates and uncertainty estimates), and the main conclusion.
Describe the study background and theoretical rationale for investigating the mechanisms of interest. Include supporting evidence or the theoretical rationale for why the intervention or exposure might affect the proposed mediators and why the mediators might affect the outcomes.
A concise description of the study background should be included to provide context for the subject matter and clinical setting of the study. Most often, mediation analyses will be used to understand the mechanisms by which an intervention or exposure might affect an outcome. It is recommended that authors make clear why mediation analyses helps to answer the substantive scientific question. Describing the theory that underpins the proposed mechanisms of interest, stating why the exposure or intervention is expected to affect the proposed mediator (action theory), and why the mediator is expected to affect the outcome (conceptual theory) is recommended. 12 This type of rationale should reflect each objective and, when possible, should be supported with empirical or qualitative evidence.
State the objectives of the study specific to the mechanisms of interest. The objectives should specify whether the study aims to test or estimate the mechanistic effects.
The background section should end with a clear statement of the main objectives of mediation analyses. The objectives should specify whether the aim is (1) to test the presence of an indirect or direct effect or (2) to estimate the magnitude of an indirect or direct effect. The objectives can also help to declare whether the aim of mediation analyses is explanatory (to explain what mediates a causal relationship) or interventional (to ask questions about possible causal mechanisms of hypothetical interventions that target the exposure or mediator). 5 When mediation analyses are used to answer a secondary question, authors should clearly state the objectives but note that the objective of mediation analyses is secondary and place it within the context of the primary objective.
If applicable, provide references to any protocols or study registrations specific to mediation analyses and highlight any deviations from the planned protocol.
If the protocol for the mediation analyses is registered (either within an overall analysis plan or as a separate secondary analysis plan), authors should report the name of the register, repository, or journal where the protocol was registered and provide the registration number or digital object identifier. If the study is not registered or linked to a published protocol, authors should explicitly declare the exploratory nature of the mediation analyses.
Specify the design of the original study that was used in the mediation analyses and where the details can be accessed, supported by a reference. If applicable, describe study design features that are relevant to mediation analyses.
Mediation analyses are often applied to data from randomized trials and observational (cohort and case-control) studies. 1 It is important for the mediation study to provide sufficient detail on design features, preferably with reference to a publication that contains detail about the original study that generated the data. In rare instances in which the original randomized trial or observational study cannot be referenced, the report for mediation analyses should provide greater detail on the study design and data sources.
Different study designs require different sets of assumptions for the estimation of indirect and direct effects in mediation analyses (see item 11). For example, in a randomized trial, it would be considered appropriate to assume that the intervention-mediator effects and the intervention-outcome effects are not confounded because of random allocation of the intervention. This is generally not the case in observational designs. Design variations within observational studies, such as case-control and cohort designs, can require different analytic approaches that each require different assumptions. 14 Therefore, it is important to provide a clear description of the original study design and data sources so the potential risks of bias can be assessed.
Describe the target population, eligibility criteria specific to mediation analyses, study locations, and study dates (start of participant enrollment and end of follow-up).
Quiz Ref ID Like most inferential studies, mediation analyses will study a sample of a defined target population. To provide an indication of representativeness, authors are recommended to provide a clear definition of the target population, factors that determine eligibility and recruitment into the study sample, and where (eg, geographic location and setting) and when (eg, range of dates) the study took place. Doing so will allow readers to gauge whether the findings from the mediation analyses are generalizable to the target population of interest and assist systematic reviewers in assessing study heterogeneity.
State whether a sample size calculation was conducted for the mediation analyses. If so, explain how it was calculated.
Sample size calculations for mediation analyses are not commonly conducted or reported, 6 , 7 partly because sample size calculations are complex and dependent on study design and analytic methods. 15 If a sample size calculation was conducted, authors should report the calculation method and the estimates used in the calculation (eg, the effect of the exposure on the mediator and residual mediator variance, the effects of the exposure and the mediator on the outcome and residual outcome variance, significance level, and desired power) along with any assumptions. If possible, providing a reference to the software that was used can facilitate reproducibility.
Specify the effects of interest.
Depending on the research question and the study objectives, investigators will aim to test or estimate 1 or more of the following possible effects: exposure-mediator effect, mediator-outcome effect, controlled direct effect, natural direct and indirect effects, 16 interventional direct and indirect effects, 17 or path-specific effects. 1 For example, Boers et al 18 reported a clinical definition of a natural indirect effect as the possible causal relationship between endovascular therapy and functional outcome that is explained by a treatment-related reduction in follow-up infarct volume.
As a more detailed definition, Stensrud and Strohmaier 19 reported their natural indirect effect as a comparison of the risk of a cardiovascular event when blood pressure values were those that would occur with intensive therapy vs the risk of a cardiovascular event when blood pressure values were those that would occur with standard therapy but in fact occurred during receipt of intensive therapy.
Because the chosen effect of interest will require a specific set of assumptions, drive the analytic method, and guide interpretation, it is essential for authors to clearly report the hypothesized effect that is most relevant to the study objectives (item 4). 5 In some instances, investigators will have multiple study objectives and multiple effects of interest. If so, it is recommended that authors link the study objectives to the possible effects of interest.
Include a graphic representation of the assumed causal model including the exposure, mediator, outcome, and possible confounders.
For most mediation analyses, investigators will apply field-specific knowledge, theories, and assumptions to propose an assumed causal model. The assumed causal model should be transparently described because it can influence how mediation analyses are conducted, and thereby influence the results and their interpretation. One practical and effective method of communicating the assumed causal model is the use of causal directed acyclic graphs ( Figure ). 20
Quiz Ref ID Causal directed acyclic graphs for mediation analyses should include nodes that represent the intervention or exposure, the mediator, the outcome, possible confounders of the relationships between these variables, and unidirectional arrows that depict the assumed causal relationships between the displayed variables. It is often useful to include both measured and unmeasured variables when there may be confounding by both types and to specify which variables were adjusted for in the analysis. It is also important to indicate possible collider variables are represented in the assumed causal model because conditioning on a collider can induce selection bias.
Specify assumptions about the causal model.
It is important to be explicit about the assumptions of a causal model because they guide the analytic approach, expose possible sources of bias, and help determine the extent to which an estimate can be interpreted as a possible causal relationship. For example, stating which unmeasured confounders of the exposure-mediator, exposure-outcome, and mediator-outcome relationships are considered important and could guide the sensitivity analyses (see item 15) and allow the reader to gauge how unmeasured confounders would influence the interpretation of the estimates.
Clearly outlining the temporal precedence of the variables in a mediation model is also important for assessing the direction of hypothesized causal relationships and the possibility of reverse causation. Critical assumptions in mediation analyses, such as no unmeasured confounding, can be expressed in the form of causal directed acyclic graphs (item 10), 21 whereas assumptions such as effect modification, positivity, and consistency will be better expressed as written statements. 22
Clearly describe the interventions or exposures, mediators, outcomes, confounders, and moderators that were used in the analyses. Specify how and when they were measured, the measurement properties, and whether blinded assessment was used.
All variables included in mediation analyses, such as the interventions or exposures, mediators, outcomes, and confounders, should be clearly identified and unambiguously defined. Authors should state how each variable was measured and describe the measurement tool (eg, a survey instrument such as the 36-Item Short Form Health Survey) that was used. Authors should clearly specify the beginning of follow-up (time zero) relative to when individuals met the eligibility criteria and when the intervention or exposure was initiated, 23 and report the relative timing of the exposure, mediator, and outcome measurements so that the possibility of immortal time bias and temporal precedence can be assessed.
The goal should be to provide sufficient detail so that others can replicate the study using the same variables and systematic reviewers can include or exclude studies or group studies based on the measured variables. When the exposure is an intervention, the Template for Intervention Description and Replication checklist 24 should be used with the AGReMA checklist. Because measurement error can introduce bias in mediation analyses, 25 it is important to report relevant measurement properties of the assessment or measure that was used (eg, reliability). In addition, authors should describe the extent to which participants and study personnel were masked to the intervention allocation or exposure level. This detail will allow for the assessment of observer and detection bias. 26
If relevant, describe the levels at which the exposure, mediator, and outcome were measured.
In some situations, mediation analyses will be applied to settings in which individuals are clustered within groups such as households, schools, hospitals, and countries. For example, in a cluster-randomized trial, researchers may study the effect of a hospital-level intervention on mediators and outcomes measured at the individual level. The data are considered multilevel or clustered because the data from individuals within 1 hospital may be more similar to each other than those from other hospitals and thus correlated. In these settings, authors should describe whether the exposures, mediators, and outcomes were assigned or measured at the group or individual level. Authors are also encouraged to describe how clustering was accounted for with regard to within- and between-cluster heterogeneity, 27 and possible spillover effects if relevant, 28 for the estimation of direct and indirect effects.
Describe the statistical methods used to estimate the causal relationships of interest. This description should specify the analytic strategies used to reduce confounding, model building procedures, justification for the inclusion or exclusion of possible interaction terms, modeling assumptions, and the methods used to handle missing data. Provide a reference to the statistical software and package used.
Broadly there are 2 major traditions for conducting mediation analyses: those deriving from the causal steps of Baron and Kenny or with a product and difference-of-coefficients framework 29 and those from the counterfactual-based framework. 1 , 30 Authors might indicate which 1 of these 2 frameworks were used in their mediation analyses. They also should clearly specify which specific methods within the chosen framework were used (eg, by providing a reference). Reporting the name and version of the statistical software and any specific packages can be useful for reproducing analyses.
Most mediation analyses will use a theory-driven approach to identify and adjust for a sufficient set of confounders of the exposure-mediator, exposure-outcome, and mediator-outcome associations. Authors should report how confounders were identified, for example, through the use of causal directed acyclic graphs, 21 the disjunctive cause criterion, 31 or when data-driven, use of variable selection procedures such as stepwise testing strategies or penalization methods in models for the mediator and outcome. It is also useful to report confounders that were identified in the assumed causal model but were not measured or adjusted for (see items 10 and 11).
Most mediation analyses will use regression models for the mediator and the outcome. Depending on the nature of these variables, investigators will select the most appropriate regression model, such as Cox regression for time-to-event mediators and outcomes or logistic regression for binary mediators and outcomes. Authors should clearly report the functional form and specification of the regression models that were used to model the mediators and outcomes and report any modeling assumptions that were made. If a variable selection procedure was used or if interactions were modeled to improve model flexibility, authors should report these so that readers can assess the appropriateness of the models that eventually inform the estimation of the direct and indirect effects.
Similar to most applied research, missing data are common in mediation analyses, and the way in which missing data are handled can affect the estimates of the direct and indirect effects. Depending on the amount of missing data and missingness patterns, various imputation methods may be used. It is important that authors state whether the data were imputed and, if so, report detailed information about the selected method for handling missing data. 32
Describe any sensitivity analyses that were used to explore causal assumptions, statistical assumptions, or both, and the influence of missing data.
Broadly, there are 2 types of assumptions in mediation analyses: causal and statistical. The causal assumptions refer to the underlying theoretical model being investigated (items 10 and 11). For example, investigators might assume that there is no residual confounding of the exposure-mediator, exposure-outcome, and mediator-outcome relationships. It is also common to make assumptions about the direction of causal relationships between mediators or the absence of common causes of multiple mediators. 33 If sensitivity analyses (such as the mediational E-value 34 ) are used to explore violation of such assumptions, authors should describe and cite the approach that was used.
Although most causal assumptions cannot be empirically verified, statistical assumptions that are inherent to modeling procedures can be empirically verified. For example, determining how well a selected model fits the observed data is often assessed using residual plots. To enable readers to understand how model fit was assessed, authors should report which goodness-of-fit assessment was used to assess the working models. Because the results from mediation analyses may vary depending on the imputation method used to account for missing data, any sensitivity analyses used to assess the method of handling missing data should be reported.
Name the institutional research board or ethics committee that approved the study and provide a description of participant informed consent or an ethics committee waiver of informed consent.
It is expected that most studies that use mediation analyses will have sought ethical approval from an institutional research board or ethics committee. This may be approval for the original randomized trial or observational study, or a separate approval for the mediation analyses. The details of the approval and how informed consent was obtained or waived should be clearly reported.
Describe the baseline characteristics of the participants included in the mediation analyses and report the total sample size and the number of participants lost during follow-up or with missing data.
To allow readers of mediation analyses to understand the characteristics of the sample and to gauge the generalizability of the findings, the baseline characteristics of the sample (demographics, clinical features, mediator, and outcome) should be reported. It is also important to report the total sample size and the number of participants lost during follow-up along with the amount and pattern of missing data for the mediators, outcomes, and possible confounders because losses to follow-up and missing data can introduce bias (see item 14). Reporting how the baseline characteristics of those lost to follow-up or with missing data compared with the participants analyzed can provide readers with a sense of how likely it is for selection bias to influence the results.
When mediation analyses are embedded in randomized trials or observational studies, it may not be sufficient to describe only the overall participants included in the primary study because the variables required for the mediation analyses may have been collected only in a subsample of the primary study sample (by intention or because of missing data). In these circumstances, it is important to report the subsample that is included in mediation analyses. It may also be helpful to report the total effect (exposure-outcome association without considering the mediator) obtained from the primary study sample compared with the total effect from the subsample used in the mediation analyses. When mediation analyses are reported as secondary analyses within a main report of a randomized trial or observational study, or when word count is limited in the main text, it may be sufficient to report this item within a supplement.
Report point estimates and uncertainty estimates for the exposure-mediator and mediator-outcome relationships. If inference concerning the causal relationship of interest is considered feasible given the causal assumptions, report the point estimate and uncertainty estimate.
Selecting which causal relationships to report from mediation analyses will depend on the study objectives (item 4). In most cases, the natural direct and indirect effects are recommended when the aim is to explain the causal relationship between an exposure and an outcome through 1 or more mediators (eg, the natural indirect effect of intensive blood pressure therapy on cardiovascular events mediated through low diastolic blood pressure had a hazard ratio of 1.12 [95% CI, 1.06-1.18] and the natural direct effect not mediated through low diastolic blood pressure had a hazard ratio of 0.63 [95% CI, 0.50-0.78]). 19 If the study objective is to estimate the causal relationship between an exposure and an outcome while a mediator is fixed at a constant level uniformly across the population, the controlled direct effect is recommended (eg, the causal relationship between ablation surgery and returning to sinus rhythm if no patient in the target population had the left atrial appendage removed had a hazard ratio of 0.14 [95% CI, 0.02-0.25] on the probability difference scale). 35
The estimation of exposure-mediator and mediator-outcome relationships will often require weaker assumptions than the estimation of direct and indirect effects. For this reason, as well as to provide more insight into the possible mechanisms of interest, authors should always report relevant estimates for the exposure-mediator and mediator-outcome relationships. When the necessary causal assumptions are thought to be plausible, authors should report unstandardized estimates, standardized estimates, or both, of direct and indirect effects along with their standard errors or 95% CIs. 36 The scale on which these effects are measured (eg, mean difference, risk difference, risk ratio, odds ratio, hazard ratio) must also be clearly reported. Authors may choose to report the proportion mediated (or eliminated) along with their 95% CIs as a descriptive summary of the results. Because there can be considerable uncertainty around the proportion mediated, especially in small samples, keeping the focus on the indirect and direct effects of interest is recommended.
Report the results from any sensitivity analyses used to assess the robustness of causal assumptions, statistical assumptions, or both, and the influence of missing data.
Quiz Ref ID The validity of most mediation analyses will depend on unverifiable causal assumptions. The main assumption is no unmeasured confounding. Reporting the results of any analyses that explore the sensitivity of the results regarding violation of the no unmeasured confounding assumption can allow the reader to judge the robustness of the findings. Several metrics can be reported, such as the mediational E-value, 34 or sensitivity parameters that quantify how much residual confounding there would need to be to invalidate the estimated direct and indirect effect. 30 Authors should be clear about the metric used and provide a brief interpretation in the context of the main findings.
If other sensitivity analyses are used to explore assumptions about the study design, measurement tools, statistical models, and missing data, the results of these analyses should be reported in the supplementary material. This will help readers gauge the plausibility of the assumptions and the robustness of the findings.
Discuss the limitations of the study, including potential sources of bias.
Studies that use mediation analyses may have a number of limitations such as failure to account for unmeasured confounding, 37 measurement error, 25 model misspecification, 38 selection bias, 39 and missing data. 40 Authors should state any limitations and comment on how they might affect the validity and veracity of the main findings.
If a sensitivity analysis was used to explore the effect of a limitation (items 15 and 19), the results should be discussed considering the main findings. Limitations should be clearly stated, and when relevant, discussed in the context of other studies. When mediation analyses are reported as secondary analyses within a main report, or when the word count is limited in the main text, it may be sufficient to report the limitations in a supplement.
Interpret the estimated effects considering their magnitude and uncertainty, plausibility of the causal assumptions, limitations, generalizability of the findings, and results from relevant studies.
The main findings with respect to the main objectives should be summarized in a concise paragraph. An important aspect of interpreting estimates from mediation analyses is appraising whether the estimate can have a possible causal interpretation. This will depend on how reasonable the causal assumptions are (item 11), possibly supplemented with results from sensitivity analyses (item 19) and other limitations (item 20).
Authors should provide a balanced discussion of these issues to allow the reader to judge whether the estimates can be given a causal interpretation. The interpretation should also be set in the context of any previously identified theoretical or evidence-based rationale for mediation analyses, particularly when the findings support or challenge theory. The generalizability of the overall findings should also be discussed to guide the application of the findings into clinical practice, if appropriate. When the mediation analyses are part of the secondary study objective, the interpretation might focus on the direct and indirect effects of interest in the context of the primary findings.
Discuss the implications of the overall results for clinical practice, policy, and science.
Authors should consider discussing whether the findings may influence clinical practice, policy, or future research while considering the limits of mediation analyses. These implications may, for example, suggest how an intervention or policy could be delivered to specifically target (or avoid targeting) particular mediators. Implications for research might suggest how interventions could be refined to improve efficiency or efficacy in future studies.
List all sources of funding or sponsorship for mediation analyses and the role of the funders/sponsors in the conduct of the study, writing of the manuscript, and decision to submit the manuscript for publication.
Information about study funding and support is important for helping readers identify potential conflicts of interest or possible influence. Authors should identify and declare all sources of study funding and support. Authors should report the name of the persons or entities supported, the name of the funder, and the grant or award number if available. Authors should explicitly outline the roles and responsibilities of the funder/sponsor in the study design, conduct, data analysis and interpretation, manuscript writing, and dissemination of results and should describe whether the funder/sponsor had input into the final decision regarding any of these aspects. If the funder/sponsor was not involved or had no influence, authors should specifically report this.
State any conflicts of interest and financial disclosures for all authors.
Conflicts of interests can be a source of bias. 41 These conflicts include financial relationships (such as employment, consultancies, stock ownership or options, honoraria, patents, and paid expert testimony), personal relationships or rivalries, academic competition, and intellectual beliefs. Financial conflicts of interest are associated with publication of research outcomes that favor the financial interest. Although the presence of a relationship or activity does not always indicate a problematic influence, conflicts should be transparently declared to allow readers to make their own judgments.
All authors should disclose any relationships or activities that might bias the study conduct and reporting. The International Committee of Medical Journal Editors has developed a disclosure form to facilitate and standardize authors’ disclosures.
Authors are encouraged to provide a statement for sharing data and code for mediation analyses.
Availability of data and code is essential for reproducing and replicating study findings. Open access to data and code facilitates validation of analytic methods during and after peer review. Furthermore, with the availability of various analytic options for mediation analyses, sharing data and code makes modeling procedures, assumptions, and estimation procedures transparent to the reviewer and reader.
If possible, data should be shared in an accessible, secure, and reliable database. Shared data should adhere to the Findable, Accessible, Interoperable and Reusable guiding principles 42 and, when possible, have a corresponding digital object identifier. At a minimum, a data and code availability statement should be provided within the report.
The AGReMA statement provides international consensus-based guidance on items that should be reported in studies that use mediation analyses. The scope of the AGReMA statement covers primary and secondary mediation analyses of randomized trials and observational studies, and it is intended to be general so that it can guide the reporting of most mediation analyses. Earlier approaches to mediation analyses, including the causal steps of Baron and Kenny or the product and difference-of-coefficients framework, 29 are valid under restricted conditions (linear models without interactions). In contrast, causal mediation analyses based on the counterfactual-based framework can be valid under general conditions (arbitrary linear and nonlinear models) and explicitly outline the causal assumptions that are required for making causal inferences. 1 , 30 Although terms such as direct effect , indirect effect , and path-specific effects are conventional terminology for mediation analyses, they should be interpreted with caution in both observational designs and randomized trials because causal assumptions may be unmet and it may not be possible to establish causal inferences.
The AGReMA project was designed to provide a minimum set of recommendations for reporting. Therefore, authors are encouraged to report additional details that are relevant to their study and readership when possible. The AGReMA-SF checklist is composed of essential items and was developed to guide the reporting of secondary mediation analyses that are reported within randomized trial or observational study reports. However, when possible (and especially when the total effect has been reported in a separate article), it is better to use the long-form checklist.
The purpose of the AGReMA statement is to improve completeness, consistency, and accuracy in reporting. It is not designed to guide conduct or to be used as a risk of bias tool. However, it could enable systematic reviewers to assess risk of bias by improving the reporting of relevant information. The AGReMA working group will aim to maximize the awareness and uptake of AGReMA by liaising with relevant journal editors and funding agencies to encourage the endorsement of the AGReMA checklists. To improve accessibility, the AGReMA checklists will be made available on an open web domain ( https://agrema-statement.org ) and indexed in the EQUATOR Network website.
This guideline and the guideline development process have several limitations. First, participants of the Delphi process and consensus meetings were purposefully selected based on expertise and familiarity with mediation analyses and scientific reporting. Although this select group of participants may not represent potential users of AGReMA, the consolidated checklist was externally reviewed and pilot tested by a broad group of 21 experts and potential users (eTables 1-2 in the Supplement ), and their feedback was used to adjust the guideline.
Second, approaches to mediation analyses are grounded in 2 distinct traditions. Proponents of both analytic traditions were included as participants and AGReMA aims to provide guidance for both approaches. Even though the intention was to include equal representation of participants from both analytic traditions, the emphasis in the reporting guidance may have been influenced by the composition of the panel.
Third, because of travel and social contact restrictions from the COVID-19 pandemic, the consensus meeting was conducted online rather than face-to-face. This format may have inhibited a more detailed and fluid discussion, but attempts were made to mitigate these issues by structuring the meeting so that participants were encouraged to discuss, introduce, and remove items. Smaller group discussions also took place after the 2-day meeting.
The AGReMA statement provides recommendations for reporting primary and secondary mediation analyses of randomized trials and observational studies. Improved reporting of studies that use mediation analyses could facilitate peer review and help produce publications that are complete, accurate, transparent, and reproducible.
Accepted for Publication: August 4, 2021.
Corresponding Author: Hopin Lee, PhD, Botnar Research Centre, Nuffield Department of Orthopaedics Rheumatology and Musculoskeletal Sciences, University of Oxford, Windmill Road, Oxford OX3 7LD, England ( [email protected] ).
The AGReMA group authors: A. Russell Localio, PhD; Ludo van Amelsvoort, PhD; Eliseo Guallar, PhD; Judith Rijnhart, PhD; Kimberley Goldsmith, PhD; Amanda J. Fairchild, PhD; Cara C. Lewis, PhD; Steven J. Kamper, PhD; Christopher M. Williams, PhD; Nicholas Henschke, PhD.
Affiliations of The AGReMA group authors: School of Medicine and Public Health, University of Newcastle, Callaghan, Australia (Williams); Department of Biostatistics, Epidemiology, and Informatics, Perelman School of Medicine, University of Pennsylvania, Philadelphia (Localio); Associate Editor, Annals of Internal Medicine (Localio); Faculty of Health, Medicine, and Life Sciences, Maastricht University, Maastricht, the Netherlands (van Amelsvoort); Assoicate Editor, Journal of Clinical Epidemiology (van Amelsvoort); Welch Center for Prevention, Epidemiology, and Clinical Research, Bloomberg School of Public Health, Johns Hopkins University, Baltimore, Maryland (Guallar); Deputy Editor, Annals of Internal Medicine (Guallar); Department of Epidemiology and Data Science, Amsterdam Public Health Research Institute, Amsterdam University Medical Center, Amsterdam, the Netherlands (Rijnhart); Institute of Psychiatry, Psychology, and Neuroscience, King’s College London, London, England (Goldsmith); Department of Psychology, University of South Carolina, Columbia (Fairchild); Kaiser Permanente Washington Health Research Institute, Seattle (Lewis); School of Health Sciences, University of Sydney, Sydney, Australia (Kamper); Nepean Blue Mountains Local Health District, Kingswood, Australia (Kamper); School of Public Health, University of Sydney, Sydney, Australia (Henschke).
Author Contributions: Drs Lee and Cashin had full access to all of the data in the study and take responsibility for the integrity of the data and the accuracy of the data analysis.
Concept and design: Lee, Cashin, Lamb, Hopewell, VanderWeele, MacKinnon, Mansell, Golub, McAuley, Localio, van Amelsvoort, Guallar, Fairchild, Kamper, Williams, Henschke.
Acquisition, analysis, or interpretation of data: Lee, Cashin, Lamb, Hopewell, Vansteelandt, VanderWeele, MacKinnon, Collins, McAuley, Localio, van Amelsvoort, Rijnhart, Goldsmith, Lewis, Williams, Henschke.
Drafting of the manuscript: Lee, Cashin, Lamb, Hopewell, Mansell, Collins, Golub, McAuley.
Critical revision of the manuscript for important intellectual content: All authors.
Statistical analysis: Lee, MacKinnon, Collins, Localio.
Obtained funding: Lee, McAuley, Kamper, Henschke.
Administrative, technical, or material support: Lee, Cashin, VanderWeele, Fairchild, Henschke.
Supervision: Lee, Lamb, Vansteelandt, MacKinnon, McAuley, Kamper, Williams, Henschke.
Conflict of Interest Disclosures: Dr Lamb reported being a member of boards for the Health Technology Assessment (additional capacity funding board, end of life care and add-on studies board, prioritization group board, and trauma board). Dr VanderWeele reported receiving personal fees from Statistical Horizons. Dr Localio reported receiving grants from the Annals of Internal Medicine . Dr Guallar reported receiving personal fees from the American College of Physicians ( Annals of Internal Medicine ). Dr Kamper reported receiving grants from the National Health and Medical Research Council of Australia Fellowship. No other disclosures were reported.
Funding/Support: This work was supported by project funding from the University of California, Berkeley, Initiative for Transparency in the Social Sciences, a program of the Center for Effective Global Action, with support from the Laura and John Arnold Foundation. Dr Lee was supported by the Neil Hamilton Fairley Early Career Fellowship award APP1126767 from the National Health and Medical Research Council. Dr VanderWeele reported receiving grant R01CA222147 from the National Cancer Institute. Dr MacKinnon was supported by grant R37DA09757 from the National Institute on Drug Abuse. Dr Collins was supported by the NIHR Oxford Biomedical Research Centre and programme grant C49297/A27294 from Cancer Research UK.
Role of the Funders/Sponsors: The funders/sponsors had no role in the design and conduct of the study; collection, management, analysis, and interpretation of the data; preparation, review, or approval of the manuscript; and decision to submit the manuscript for publication.
Disclaimer: Dr Golub is Deputy Editor of JAMA , but he was not involved in any of the decisions regarding review of the manuscript or its acceptance.
Additional Contributions: We thank Anika Jamieson, BBA (Neuroscience Research Australia), for administrative support and Rob Froud, PhD (director and shareholder of Clinvivo), and the wider Clinvivo team for their services in executing the Delphi study. Ms Jamieson and Dr Froud were not compensated for their roles. The Clinvivo team was compensated for their role in the study. We acknowledge the contributions made by the Delphi panelists, the AGReMA international consensus meeting participants, the AGReMA external review experts (eTable 1 in the Supplement ), and the UK EQUATOR Centre for administrative support.
- Register for email alerts with links to free full-text articles
- Access PDFs of free articles
- Manage your interests
- Save searches and receive search alerts
- Open access
- Published: 25 October 2021
Mediation analysis methods used in observational research: a scoping review and recommendations
- Judith J. M. Rijnhart 1 ,
- Sophia J. Lamp 2 ,
- Matthew J. Valente 3 ,
- David P. MacKinnon 2 ,
- Jos W. R. Twisk 1 &
- Martijn W. Heymans 1
BMC Medical Research Methodology volume 21 , Article number: 226 ( 2021 ) Cite this article
41k Accesses
83 Citations
2 Altmetric
Metrics details
Mediation analysis methodology underwent many advancements throughout the years, with the most recent and important advancement being the development of causal mediation analysis based on the counterfactual framework. However, a previous review showed that for experimental studies the uptake of causal mediation analysis remains low. The aim of this paper is to review the methodological characteristics of mediation analyses performed in observational epidemiologic studies published between 2015 and 2019 and to provide recommendations for the application of mediation analysis in future studies.
We searched the MEDLINE and EMBASE databases for observational epidemiologic studies published between 2015 and 2019 in which mediation analysis was applied as one of the primary analysis methods. Information was extracted on the characteristics of the mediation model and the applied mediation analysis method.
We included 174 studies, most of which applied traditional mediation analysis methods ( n = 123, 70.7%). Causal mediation analysis was not often used to analyze more complicated mediation models, such as multiple mediator models. Most studies adjusted their analyses for measured confounders, but did not perform sensitivity analyses for unmeasured confounders and did not assess the presence of an exposure-mediator interaction.
Conclusions
To ensure a causal interpretation of the effect estimates in the mediation model, we recommend that researchers use causal mediation analysis and assess the plausibility of the causal assumptions. The uptake of causal mediation analysis can be enhanced through tutorial papers that demonstrate the application of causal mediation analysis, and through the development of software packages that facilitate the causal mediation analysis of relatively complicated mediation models.
Peer Review reports
Mediation analysis is increasingly being applied in many research fields [ 1 ], including the field of epidemiology. Mediation analysis decomposes the total exposure-outcome effect into a direct effect and an indirect effect through a mediator variable [ 2 , 3 , 4 ]. For example, mediation analysis can be used to investigate BMI as a mediator of the relation between smoking and insulin levels [ 5 ], or to investigate food expenditures as a mediator of the relation between socioeconomic status and healthiness of food choices [ 6 ]. Mediation analysis is therefore an important statistical tool for gaining insight into the mechanisms of exposure-outcome effects [ 3 ].
Throughout the years, various methods for mediation analysis have been described in the literature. Building on the path analysis method described by Sewall Wright [ 7 , 8 ], Judd and Kenny described the causal steps method in 1981 [ 9 ], followed by an adaptation of this method in 1986 by Baron and Kenny [ 10 ]. The causal steps method relies on a sequence of significance tests to determine the presence of a mediated effect. Later papers recommended estimating the indirect effect based on the product-of-coefficients method or the difference-in-coefficients method to determine the presence of a mediated effect [ 3 , 11 , 12 , 13 ]. Here we refer to these methods as ‘traditional mediation analysis’. In the last decade, causal mediation analysis gained popularity. Causal mediation analysis provides general definitions of causal direct, indirect, and total effects, which can be estimated using various estimation approaches [ 4 , 14 , 15 ]. Causal and traditional mediation analysis can provide the same effect estimates for mediation models estimated with linear regression [ 16 , 17 ], but this does not necessarily hold for mediation models estimated with non-linear regression [ 18 , 19 ]. Causal mediation analysis is preferred for the latter models, as for these models causal mediation analysis provides causal effect estimates, while traditional mediation analysis can in some situations only be used to test the presence of a mediated effect [ 19 ].
Although the theoretical definitions of the causal direct, indirect, and total effects are not new [ 4 , 14 , 15 ], the uptake of causal mediation analysis in practice has remained low for many years [ 20 ]. In the past decade, various software programs have been developed for the estimation of causal mediation effects, enabling researchers to perform causal mediation analysis in all major software packages (i.e., SAS, SPSS, Stata, R, and M plus ) [ 21 , 22 , 23 , 24 , 25 , 26 , 27 , 28 ]. However, it is not clear whether these software packages increased the uptake of causal mediation analysis in epidemiologic research. A recent review showed that traditional mediation analysis is still most frequently used to analyze data from randomized controlled trials [ 29 ]. It remains unclear whether this also holds for observational studies, which are common in the field of epidemiologic research.
The aim of this paper is to review the methodological characteristics of mediation analyses performed in observational epidemiologic studies published between 2015 and 2019 and to provide recommendations for the application of mediation analyses in future studies. In this paper we performed a scoping review, as the aim of this paper is relatively broad and concerns the collection of information on a range of methodological characteristics rather than information on a clearly defined substantive question [ 30 ]. In the next section, we first provide an overview of traditional and causal mediation analysis methods. Then we describe the methods and results of our scoping review. Finally, we provide recommendations for the application of mediation analysis in future studies.
Traditional mediation analysis
Traditional mediation analysis is based on the estimation of the four pathways shown in Fig. 1 [ 3 , 10 ]. In Fig. 1 A, the c path represents the total exposure-outcome effect. In Fig. 1 B, the a path represents the exposure-mediator effect, the b path represents the mediator-outcome effect, and the c’ path represents the direct exposure-outcome effect. When the mediator and outcome are both continuous, the paths in Fig. 1 are estimated using the following three linear regression eqs. (9):
where the c coefficient in eq. 1 represents the total exposure-outcome effect. The a coefficient in eq. 2 represents the exposure-mediator effect. The b coefficient in eq. 3 represents the mediator-outcome effect when adjusted for the exposure, and the c’ coefficient represents the direct exposure-outcome effect when adjusted for the mediator. The i 1 , i 2 , and i 3 terms represent intercepts and the ε 1 , ε 2 , and ε 3 terms represent residuals. Finally, Z represents a set of confounders. The inclusion of confounders in eqs. 1 , 2 , and 3 should always be considered when a mediation analysis is performed based on observational data, as the exclusion of confounders will result in biased effect estimates [ 3 ].
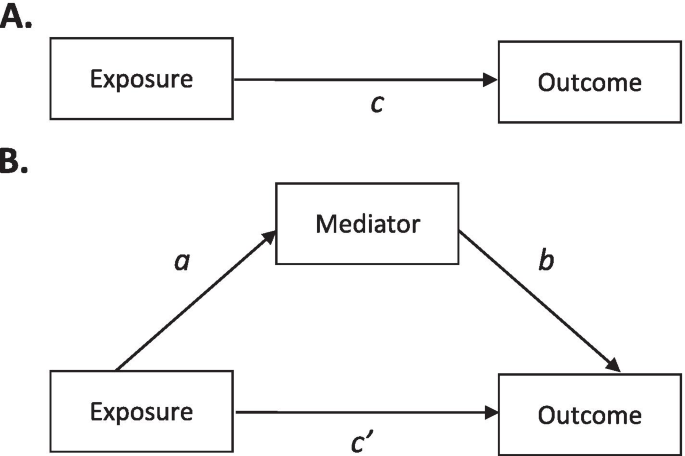
Path diagram of a single mediator model.
Traditional mediation analysis defines the direct, indirect, and total effects in terms of the linear regression coefficients from eqs. 1 , 2 , and 3 [ 3 , 12 ]. The total effect is defined and estimated as the c coefficient from eq. 1 and the direct effect is defined and estimated as the c’ coefficient from eq. 3 . The indirect effect is defined and estimated as the product of the a and b coefficients ( ab ) and as the difference between the c coefficient and the c’ coefficient ( c-c’ ). These two indirect effects are mathematically equivalent when the regression coefficients are estimated with linear regression [ 13 ]. The relative size of the mediated effect can be assessed using the proportion mediated, which represents the size of the indirect effect estimate relative to the total effect estimate, or by interpreting the standardized indirect effect estimate as a Cohen’s d [ 3 ].
Some of the first papers on mediation analysis recommended to assess the statistical significance of the indirect effect estimate with a z -test or a confidence interval based on the multivariate delta standard error [ 10 , 31 , 32 , 33 ]. However, these methods are not recommended, as they assume that the indirect effect estimate follows a normal sampling distribution, which often does not hold [ 34 ]. As a result, the z -test and confidence interval based on the multivariate delta standard error have relatively low power to detect a statistically significant indirect effect [ 35 , 36 , 37 ]. Confidence intervals that do take into account the nonnormal sampling distribution of the indirect effect estimator are therefore preferred, such as the distribution of the product confidence interval, Monte Carlo confidence interval, and bootstrap confidence intervals [ 34 , 36 , 38 ].
Mediation analysis is based on the assumption of temporal precedence of the exposure, mediator, and outcome, which means that changes in the exposure are assumed to precede changes in the mediator, and that changes in the mediator are assumed to precede changes in the outcome [ 3 , 39 ]. Furthermore, traditional mediation analysis is based on parametric regression assumptions. In other words, the residuals of the linear regression models are assumed to be normally distributed and homoscedastic across values of the independent variables in the model, the a , b , c , and c’ coefficients are assumed to represent their correct functional form (e.g., linear or quadratic), the observations are assumed to be independent, and it is assumed that there are no effect modifiers or omitted confounders of the estimated effects [ 3 , 40 ]. Effect modifiers can be taken into account by including interaction terms (i.e., exposure-by-covariate or mediator-by-covariate) in the models and by subsequently estimating the direct and indirect effects for different values of the effect modifier. This can, for example, be done by estimating the effects for specific categories of a categorical effect modifier or by centering a continuous effect modifier at a clinically relevant valu e [ 3 , 11 ]. The effect estimates can be adjusted for measured confounders by adding the confounder variables to all estimated regression equations.
Ambiguities arise when traditional mediation analysis is used to estimate the effects for mediation models with non-continuous mediator and outcome variables [ 12 , 41 , 42 ]. For example, the product-of coefficients and difference-in-coefficients methods provide different indirect effect estimates when based on the coefficients from non-linear regression models, such as logistic regression or Cox proportional-hazards regression [ 12 , 41 , 43 ]. Furthermore, although it has been recommended to assess the presence of exposure-mediator interactions in the traditional mediation analysis literature, guidance is scarce on the estimation and interpretation of effects for mediation models with an exposure-mediator interaction [ 3 , 9 ]. Recent papers have shown that group-mean centering of the continuous mediator variable in traditional mediation analysis yields effect estimates similar to the effect estimates from causal mediation analysis for mediation models with a continuous outcome and an exposure-mediator interaction [ 16 ], but not necessarily for mediation models with a binary outcome and an exposure-mediator interaction [ 18 ].
Causal mediation analysis
Causal mediation analysis clarifies the ambiguities that arise in traditional mediation analysis [ 16 , 18 , 44 ]. Causal mediation analysis is based on the counterfactual framework [ 4 , 14 , 15 ], and distinguishes causal effect definitions from causal effect estimation [ 45 ]. A strength of the causal effect definitions is that they are non-parametric and therefore can be applied to any type of mediation model to derive the causal effect estimates. This includes models with an exposure-mediator interaction and models with non-continuous mediator variables or non-continuous outcome variables [ 46 ].
Causal effect definitions
Causal mediation analysis defines causal effects as the difference between two counterfactual outcomes [ 47 , 48 ]. A counterfactual outcome is an individual’s outcome value that would be observed when exposed to a certain exposure value. In the remainder of this section we denote the outcome as Y, and the exposure values of interest as x and x* . In theory, two counterfactual outcomes can be observed for one individual over the same time period, one based on exposure value x and one based on exposure value x* [ 47 , 48 ]. The individual’s counterfactual outcome under exposure value x is denoted as Y i ( x ), and the individual’s counterfactual outcome under exposure value x* is denoted as Y i ( x* ). The causal exposure effect is defined as the difference between these two counterfactual outcomes observed for the same individual over the same time period, i.e., Y i ( x ) – Y i ( x ∗ ).
The counterfactual outcomes in a mediation model are not only dependent on exposure values, but also on mediator values [ 4 ]. We denote the mediator as M and the mediator values as m . The counterfactual notation for the outcome can be extended by including this mediator value. An individual’s counterfactual outcome under exposure value x and mediator value m is denoted as Y i ( x , m ), and the same individual’s counterfactual outcome under exposure value x* and mediator value m as Y i ( x* , m ). The difference between these two counterfactual outcomes observed for the same individual over the same time period is the controlled direct effect (CDE), i.e., Y i ( x , m ) – Y i ( x ∗ , m ). The CDE is the direct effect of changing an individual’s exposure value from x to x* , while holding the mediator value constant at m [ 4 ]. The mediator value m is determined by the researcher and reflects a value of clinical or policy relevance [ 4 ].
Instead of holding the mediator constant at a predetermined value, we can also let the mediator take on the value that would naturally be observed under exposure values x and x* [ 4 ]. Two counterfactual mediator values can be observed for an individual under the two exposure values x and x* : the counterfactual mediator value under exposure value x , i.e., M i ( x ), and the counterfactual mediator value under exposure value x* , i.e., M i ( x* ). We can now replace mediator value m with these two counterfactual mediator values, resulting in four nested counterfactual outcome values: Y i ( x , M i ( x )), Y i ( x , M i ( x ∗ )), Y i ( x ∗ , M i ( x )), and Y i ( x ∗ , M i ( x ∗ )) [ 4 , 49 ]. These four counterfactual outcomes are referred to as nested counterfactual outcomes, because the counterfactual mediator values are nested within the counterfactual outcomes values [ 4 ].
Five causal effects are defined based on the differences between these nested counterfactual outcomes: the pure natural direct effect (PNDE), the total natural direct effect (TNDE), the pure natural indirect effect (PNIE), the total natural indirect effect (TNIE), and the total effect (TE) [ 4 , 15 ]. Table 1 provides an overview of these causal effects and their respective interpretations. For the natural direct effects we block the effect through the mediator by holding each individual’s mediator constant at either M i ( x ) or M i ( x* ), while for the natural indirect effects we block the effect through the exposure by holding the exposure constant at either x and x* [ 1 , 50 ]. For the TE, we allow information to flow through both the exposure and mediator, varying both the exposure value and the counterfactual mediator value.
The causal effects are defined at the individual level, but in practice we are unable to observe multiple counterfactual outcomes for the same individual over the same time period [ 47 , 48 ]. Therefore, we are unable to estimate individual-level causal effects. This has been referred to as the fundamental problem of causal inference [ 47 ]. Instead, we can estimate the population-average causal effects based on the expected difference between two population-average (nested) counterfactual outcomes [ 4 , 14 , 47 ]. To ensure that the PNDE, TNDE, PNIE, and TNIE have a causal interpretation at the population-average level, the following four assumptions need to hold [ 4 , 46 ]:
no unmeasured confounding of the exposure-outcome effect;
no unmeasured confounding of the mediator-outcome effect;
no unmeasured confounding of the exposure-mediator effect;
no confounders of the mediator-outcome effect that are affected by the exposure.
Assumption 4 is also known as the cross-world independence assumption. In practice this is often a strong assumption [ 51 ], for example because often there will be multiple mediators of the exposure-outcome effect. For the CDE only assumptions 1 and 2 have to hold, and for the TE only assumption 1 has to hold. Finally, consistency is assumed, which means that the observed mediator and outcome values would also have been observed had the individual randomly been assigned the observed exposure and mediator values [ 46 , 52 ].
Causal effect estimation
Various estimation approaches have been developed to estimate the causal direct, indirect, and total effects at the population-average level, including simulations, numerical integration, multiple regression analysis, and natural effect models [ 19 , 23 , 53 , 54 , 55 ]. Most of these methods use eq. 2 and/or eq. 3 as input. Provided that the relevant parametric assumptions hold, the regression coefficients from eqs. 2 and 3 can be used to compute the causal mediation effects. To accommodate the estimation of pure and total natural direct and indirect effects, eq. 3 is typically extended with an exposure-mediator interaction term.
The simulation-based approach can be applied based on both parametric and non-parametric models [ 25 , 53 ]. The parametric simulation-based approach uses the sampling distributions of the estimated parameters from eqs. 2 and 3 to simulate the potential mediator and outcome values for each subject. Based on the simulated potential outcomes, the causal effects are computed for each subject. Subsequently, the causal effects are averaged to arrive at the population-average causal effects. The non-parametric simulation-based approach estimates possibly non-parametric models for the mediator and outcome variables within a prespecified number of bootstrap resamples. Based on these models the potential mediator and outcome values are simulated for each subject. Then based on these simulated potential outcomes, the causal effects are estimated and averaged to get the population-average causal effects.
Numerical integration uses eqs. 2 and 3 as input [ 4 , 23 ]. Based on these equations, average expected outcome values are estimated conditional on the two exposure levels of interest, i.e., x and x* , and all mediator values. These expected outcome values are weighted by the mediator distributions observed under x and x* to estimate the population-average nested potential outcomes, which are subsequently subtracted to get the population-average causal effect estimates.
The regression-based method estimates the average potential outcomes based on the regression coefficients in eqs. 2 and 3 [ 19 , 46 , 56 ]. These estimated potential outcomes are subsequently subtracted to estimate the population-average causal mediation effects. The regression-based effects for mediation models with a binary or time-to-event outcome were originally derived on the risk-ratio scale, therefore this method poses an additional rare outcome assumption when the causal effects are estimated on the odds-ratio scale or hazard-ratio scale [ 56 , 57 ]. This assumption requires the outcome prevalence to be low across all strata of the exposure and mediator variable [ 58 ]. When this assumption is violated, the effect estimates on the odds-ratio scale or hazard-ratio scale can still be used to assess the presence of a mediated effect, but they do not have a causal interpretation [ 56 ]. To ensure a causal interpretation, the effects can alternatively be estimated on the risk-ratio scale using log-linear regression or on the survival-time ratio scale using accelerated failure time models [ 28 , 57 ].
In natural effect models the natural direct effect and natural indirect effect are each represented by a single regression coefficient [ 25 ]. In contrast with the other estimation methods, natural effect models require the estimation of only one of the aforementioned regression equations, i.e., eqs. 2 and 3 , in addition to the natural effect model [ 59 ]. Natural effect models are estimated using a weighting-based approach or a imputation-based approach. The weighting-based approach creates an expanded dataset with weights for each subject based on eq. 2 [ 54 , 60 ]. The natural effects model is subsequently estimated by regressing the outcome on the two exposure values of interested, i.e., x and x* , and the covariates, while weighting each observation based on the computed weights. The imputation-based approach creates an expanded dataset in which the missing potential outcome values are imputed based on information from eq. 3 [ 55 ]. Based on this complete dataset, a natural effects model is estimated.
Traditional mediation analysis versus causal mediation analysis
For certain mediation models, traditional mediation analysis provides the same effect estimates as causal mediation analysis. Traditional mediation analysis provides the same effect estimates as causal mediation analysis for single mediator models with a continuous mediator and a continuous outcome [ 16 , 17 , 45 ]. This also means that traditional mediation analysis fails to provide causal effect estimates when the four no (unmeasured) confounding assumptions are violated. For mediation models with a binary or time-to-event outcome, traditional and causal mediation analysis do not necessarily provide the same effect estimates [ 16 , 18 ]. For these models, the effect estimation in traditional mediation analysis is most closely related to the regression-based estimation approach in causal mediation analysis, which also estimates the indirect effect using the product-of-coefficients method in the absence of exposure-mediator interaction. However, an important difference is the rare outcome assumption posed by causal mediation analysis for mediation models with a binary or time-to-event outcome. This rare outcome assumption clarifies that the traditional effect estimates based on logistic regression and Cox proportional hazards regression only have a causal interpretation when the outcome is rare.
When there are multiple mediators of the exposure-outcome effect, it is important to take into account all these mediators, because they may be correlated or they may influence one another violating the fourth no confounding assumption, i.e., no confounders of the mediator-outcome effect that are affected by the exposure. Causal mediation analysis clarifies the necessary additional causal assumptions for models with multiple mediators and various methods have been developed for the estimation of causal effects for multiple mediator models [ 25 , 61 , 62 , 63 ].
In recent years, various causal mediation software packages have been developed that enable researchers to apply causal mediation analysis based on only a few lines of code [ 21 , 22 , 23 , 24 , 25 , 26 , 27 , 64 ]. However, it remains unclear whether the availability of these causal mediation programs has increased the uptake of causal mediation analysis in practice. In the next section we describe the set-up of our scoping review in which we collected information on the methodological characteristics of mediation analyses in published observational studies, with a special focus on the mediation analysis method used.
Study design
This scoping review is reported in accordance with the Preferred Reporting Items for Systematic Reviews and Meta-Analyses (PRISMA) statement [ 65 ] and the PRISMA-ScR extension [ 66 ]. The PRISMA-ScR checklist can be found in supplementary appendix 1 . The protocol for this scoping review was not registered in the international register of systematic reviews, because we did not extract data on clinical outcomes [ 67 ].
Our search strategy is based on the MEDLINE search performed by Vo and colleagues [ 29 ] who conducted a review aimed to assess the methodological characteristics of mediation analyses conducted in randomized controlled trials between 2017 and 2018. We adapted the search conducted by Vo and colleagues [ 29 ] in four ways. First, we searched both the MEDLINE and EMBASE, as EMBASE has been shown to contain many unique references compared to MEDLINE when performing medically-oriented searches [ 68 ]. Second, we extended the search period to 5 years, including papers published between January 1st 2015 and December 31st 2019, as estimation methods for causal mediation analysis have been implemented in all major software packages since 2015 [ 21 , 22 , 23 , 24 , 25 , 26 , 27 , 28 ]. Third, in addition to the keywords “mediation analysis”, mediation, and mediator used by Vo and colleagues [ 29 ], we also included the following keywords to increase the chances of finding papers that conducted a mediation analysis: “mediation analys*”, mediators, “indirect effect”, “indirect effects”, “causal steps”, “product-of-coefficients”, and “difference-in-coefficients”. Fourth, we searched for observational studies only, as the earlier study performed by Vo and colleagues [ 29 ] examined the methodological characteristics of mediation analyses conducted in randomized controlled trials. The MEDLINE (accessed through PubMed) and EMBASE (accessed through embase.com ) searches were performed on May 20th 2020. The complete MEDLINE and EMBASE search strategies can be found in supplementary appendix 2 .
After removing duplicate records, two authors (JJMR and SJL) independently screened the titles and abstracts of the identified records for eligibility using Rayyan software [ 69 ]. Records were eligible for inclusion when published between 2015 and 2019, written in English, based on observational human subjects data, and the title or abstract indicated that it concerned an original research paper in which mediation analysis was performed. Full texts of the eligible records were obtained. When full texts were not available, full texts were requested from the corresponding author by email. Two authors (JJMR and SJL) independently screened the full texts for eligibility. Full texts in which mediation analysis was not performed as one of the primary analysis methods and conference abstracts were excluded, as we expected that these records did not contain a sufficient amount of details on the performed mediation analyses. Disagreements at any stage of the screening process were resolved by a third author (MJV).
A data extraction form was developed and pilot tested by one author, who subsequently extracted data from all eligible papers (JJMR). To ensure the quality of the extracted data, two authors (MJV and SJL) each independently extracted data from a random subsample of 12.5% of the eligible papers, i.e., 25% of the papers in total. Disagreements were resolved through discussion. The data extraction included the mediation analysis method used, publication year, study design, sample size, software used, the number of exposure, mediator, and outcome variables, each variable’s measurement level, use of a path diagram, use of repeated measurements, single or multiple mediator model, the types of estimated regression models, the type of confidence interval for the indirect effect estimates, the reporting of standard errors and p -values for the indirect effect estimates, use of effect size measures, inclusion of confounders in the analyses, use of sensitivity analyses for unmeasured confounders, assessment of exposure-mediator interaction, assessment of effect modifiers (i.e., exposure-by-covariate or mediator-by-covariate), and the discussion of the rare outcome assumption for mediation models with a binary or time-to-event outcome estimated based on traditional mediation analysis or regression-based causal mediation analysis. For papers based on longitudinal data we extracted the number of measurement waves included in the analyses and the type of longitudinal mediation model estimated. For multiple mediator models we extracted the type of multiple mediator model and the assessment of mediator-by-mediator interactions. The extracted data were summarized using descriptive statistics stratified by the mediation analysis method used. Categorical variables were summarized using frequencies and percentages, and continuous variables were summarized using medians and interquartile ranges.
The search returned 369 records through the MEDLINE database and 381 records through the EMBASE database (Fig. 2 ). After removing duplicates, 633 records remained for the title and abstract screening. Conflicting decisions were made for 25 records (3.9%) and were resolved by a third author. A total of 407 records were excluded after the title and abstract screening, with the most common reason for exclusion being that the title or abstract did not indicate that mediation analysis was performed ( n = 323). Two hundred twenty-six records were eligible for full-text screening. For one of the eligible records, no full text could be obtained. Conflicting decisions were made for 10 papers (4.4%) and were resolved by a third author. Based on the full text screening, another 43 records were excluded, of which 34 did not perform mediation analysis as one of the primary analyses, 11 were conference abstracts, 5 provided too little information for data extraction, and 1 paper was a methodological study. A total of 174 papers were included in the review. A complete list of included papers can be found in the supplementary appendix 3 and the dataset with the extracted data in supplementary appendix 4 .
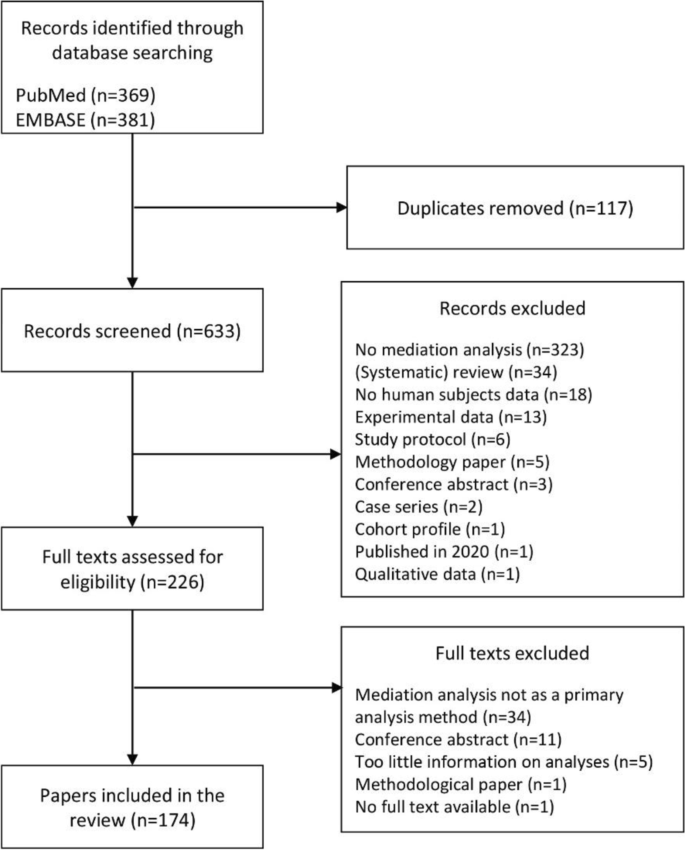
Flow diagram representing the process of identifying papers eligible for the review of the methodological characteristics of mediation analyses performed based on observational epidemiologic studies published between 2015 and 2019
Table 2 provides an overview of the methodological characteristics of the mediation analyses performed by the studies included in this scoping review. Of the 174 studies included in this scoping review, 123 used traditional mediation analysis (70.7%). Twenty-eight papers (16.1%) used the causal steps method ( n = 14), the change-in-coefficient method ( n = 9), or the test of joint significance ( n = 5). In line with a previous paper, we define the change-in-coefficient method as the assessment of the presence of a mediated effect based on the change in the exposure-outcome coefficient before and after inclusion of the mediator in the model [ 20 ]. The test of joint significance is based on the joint statistical significance of the exposure-mediator and mediator-outcome effect estimates. The causal steps method, change-in-coefficient method, and test of joint significance are similar in that they do not provide indirect effect estimates. Therefore, we collapsed the descriptive statistics in Table 2 across these three methods. Twenty-three papers used causal mediation analysis (13.2%), of which 10 used the regression-based estimation approach (43.5%), 7 used the simulation-based estimation approach (30.4%), 4 used natural effects models (17.4%), 1 used numerical integration (4.3%), and for 1 paper it remained unclear which estimation method was used.
Twenty-one studies were published in 2015 (12.1%), 29 in 2016 (16.7%), 27 in 2017 (15.5%), 47 in 2018 (27.0%), and 50 in 2019 (28.7%). The cross-sectional study design was the most common (48.3%), followed by the prospective cohort design (44.8%). The case-control design and retrospective cohort design were less common (4.0 and 2.9% respectively). Studies using causal mediation analysis were more often based on a case-control design and less often on a cross-sectional design than studies using other mediation analysis methods. The median number of participants eligible for analyses was 428.5 (interquartile range: 157.5–2026.0). SPSS was most commonly used to perform mediation analysis (38.5%), followed by Stata (15.5%), M plus (14.9%), SAS (12.1%), R (8.0%), and LISREL (0.6%). Thirteen studies did not mention the used software program (7.5%). Five studies mentioned the use of multiple software programs (2.9%).
Most studies considered one exposure variable (66.7%) or one outcome variable (72.4%). Eighty-six studies considered one mediator variable (49.4%), 35 studies considered two mediator variables (20.1%), and 53 studies considered three or more mediator variables (30.5%). The majority of studies performed mediation analysis based on continuous exposure, mediator, and outcome variables. Causal mediation analysis was used relatively often to analyze binary outcomes, but was never used to analyze latent variables. One-hundred-thirty studies reported a diagram of the mediation model (74.7%). Ten of these studies included confounders in the diagram (7.7%).
Forty-one studies performed mediation analysis based on repeated measurements of the variables in the mediation model (23.6%). The median amount of measurement waves among these studies was 2.0 (IQR: 2.0–4.0). The methodology used to analyze repeated measurements varied from adjustment for first-wave measurements to more complicated models, such as cross-lagged panel models, latent growth curve models, and multilevel models. A detailed table of the used methods to estimate mediation models based on repeated measurements can be found in supplementary appendix 5 .
One-hundred-fourteen studies reported single mediator models only (65.5%), 41 studies reported multiple mediator models only (23.6%), and 16 studies reported both single and multiple mediator models (9.2%). Of the 16 studies reporting both single and multiple mediator models, 10 studies reported parallel multiple mediator models in addition to single mediator models (62.5%), 5 studies reported serial multiple mediator models in addition to single mediator models (31.3%), and 1 study reported both parallel and serial multiple mediator models in additional to single mediator models (6.3%). Of all 57 studies reporting multiple mediator models, 37 studies reported parallel multiple mediator models (64.9%), 18 studies reported serial multiple mediator models (31.6%), and 2 studies reported both parallel and serial multiple mediator models (3.5%). None of these studies reported that they assessed mediator-by-mediator interactions. Most studies using causal mediation analysis reported single mediator models (87.5%).
Most studies used linear regression to estimate the mediator and outcome eqs. (70.1 and 62.6%, respectively). Of the 47 studies using a (traditional or causal) regression-based estimation approach for models with a binary or time-to-event outcome, 1 study discussed the rare outcome assumption (2.1%) and 3 studies estimated effects on the relative-risk scale or risk-difference scale (6.4%). The latter 4 studies all used causal mediation analysis. Of the 123 studies using traditional mediation analysis, 98 used the product-of-coefficients estimator (79.7%), 3 used the difference-in-coefficients estimator (2.4%), 16 did not specify the used method for calculating the indirect effect (13.0%), and 6 did not report indirect effect estimates (4.9%). Bias-corrected bootstrap confidence intervals were the most commonly reported type of confidence interval for the indirect effect estimates (20.1%). Thirty-seven studies reported a standard error for the indirect effect estimate (21.3%) and 62 studies reported a p -value for the indirect effect estimate (35.6%). The proportion mediated was the most commonly used effect size measure (37.5%). Six studies determined effect sizes by comparing standardized effect estimates with Cohen’s d (3.4%).
Most studies included confounders in the mediation analyses (71.8%). Only 3 studies performed sensitivity analyses for unmeasured confounders (1.7%), and 1 study discussed the no-unmeasured confounder assumptions and concluded that the estimated models were adjusted for all important confounders (0.6%). All studies performing or discussing sensitivity analyses for unmeasured confounders used causal mediation analysis. Most studies did not investigate moderated mediation (78.2%). Ten studies stratified the analyses a priori based on an effect modifier (5.7%). Twenty-eight studies investigated moderation by including interaction terms in the models (16.1%), of which 17 studies reported that the coefficient for the interaction term was not statistically significant. Of the 11 studies with statistically significant interaction effects, 5 studies reported overall effects (45.5%), 3 studies reported the estimated coefficient for the interaction term (27.3%), and 3 studies stratified the analyses based on the effect modifier (27.3%). Of the 17 studies that tested exposure-mediator interaction, 8 reported a statistically significant interaction (35.3%). Only 2 of these studies incorporated the exposure-mediator interaction in the effect estimates. Both of these studies used causal mediation analysis to estimate the effects.
The aim of this paper was to review the methodological characteristics of mediation analyses performed in observational epidemiologic studies published between 2015 and 2019 and to provide recommendations for the application of mediation analyses in future studies. This scoping review showed that traditional mediation analysis was frequently used in observational studies published between 2015 and 2019. A minority of studies used causal mediation analysis and compared to the other mediation analysis methods, causal mediation analysis was less often used to analyze relatively complex mediation models, such as models with latent variables and multiple mediator models. The majority of studies included measured confounders in their mediation analyses. However, sensitivity analyses for unmeasured confounding, exposure-mediator interaction, and the rare outcome assumption for binary and time-to-event outcomes were only discussed in a few papers, most of which used causal mediation analysis. Based on the findings in this scoping review, the next section provides recommendations for conducting mediation analysis based on real-life data.
Recommendations for conducting mediation analysis
Mediation analysis method.
Although the causal steps method, change-in-coefficient method, and the test of joint significance are relatively old methods for mediation analysis, they were still applied in over 15 % of the papers included in this scoping review. These methods are not preferred for mediation analysis, as they do not necessarily provide mediated effect estimates [ 70 ]. Furthermore, the causal steps method and the test of joint significance rely completely on the statistical significance of the estimated coefficients. The causal steps method does therefore not account for inconsistent mediation models in which the direct and indirect effect estimates have opposite signs, where the total effect estimate can approach zero [ 11 , 34 , 71 ]. Therefore, mediation effects might be missed when relying on the causal steps criteria. The change-in-coefficient method may result in biased conclusions for models with a binary or time-to-event outcome as the change in the coefficient may reflect a change in the scales of the effect estimates (i.e., non-collapsibility) instead of mediation [ 41 , 44 , 72 ].
Although traditional and causal mediation analysis provide the same effect estimates for some models, causal mediation analysis is generally preferred over traditional mediation analysis. Causal mediation analysis explicitly lays out all assumptions needed for the causal interpretation of the effect estimates [ 19 , 73 ]. Although some of these causal assumptions are the same as the parametric assumptions posed by the other mediation analysis methods, causal mediation analysis also provides guidance for when these assumptions do not hold [ 74 ]. For example, when there are unmeasured confounders, sensitivity analyses might be used to assess how the effect estimates change based on a range of plausible assumptions regarding the magnitude of the effect of the confounder on the variables in the mediation model [ 53 , 75 , 76 , 77 ]. The clarification of the causal assumptions is an important contribution of causal mediation analysis, as mediation models are inherently causal models.
Causal mediation analysis is also preferred over traditional mediation analysis as it provides causal effect definitions that can be used to estimate causal effects for any mediation model [ 45 ]. In contrast, the traditional estimators were originally derived based on linear regression coefficients [ 9 ], and are also applied based on the coefficients from other types of regression models, such as logistic regression and Cox regression [ 12 , 78 ]. Provided that the no (unmeasured) confounding assumptions hold, traditional mediation analysis provides causal effect estimates for mediation models estimated with linear regression [ 16 , 17 , 19 ]. However, when eq. 1 is estimated with linear regression and eq. 2 is estimated with non-linear regression, e.g., logistic regression or Cox proportional hazards regression, traditional and causal mediation analysis only provide the same effect estimates when the mediator follows a normal distribution, the outcome is rare, and interactions are absent [ 17 , 19 , 79 ]. When there is exposure-mediator interaction in a mediation model with a binary outcome variable, the traditional direct effect estimates map onto the causal CDE estimates, rather than the causal PNDE and TNDE estimates [ 18 ].
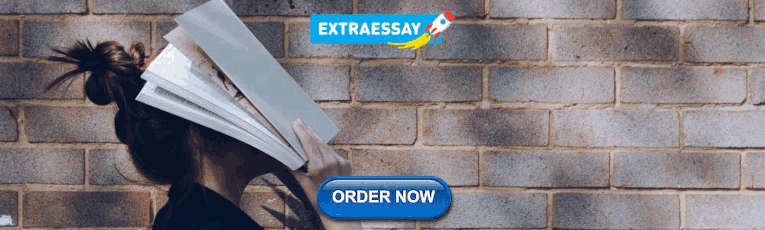
Parametric and causal assumptions
It is generally recommended to assess and discuss the relevant parametric and causal assumptions. The no (unmeasured) confounding assumptions are essential to ensure a causal interpretation of the effect estimates and are especially relevant for observational studies, as all paths in the mediation model are observational and adjustment for confounders is essential to ensure the causal interpretation of the effect estimates. Directed acyclic graphs (DAGs) can be used to help determine the confounders of the paths in the mediation model, as DAGs visualize the causal paths in the mediation model, including the confounders of these paths [ 49 , 80 ]. The majority of studies in this review reported a path diagram of the mediation model, but these path diagrams are different from DAGs, as path diagrams typically represent the statistical model, while DAGs represent the theoretical model including (unmeasured) confounders of each pathway in the mediation model [ 81 ]. Future studies could clarify the causal structure of their mediation model by reporting a DAG, possibly in addition to the path diagram. The potential impact of unmeasured confounders on the effect estimates can be assessed through sensitivity analyses [ 53 , 77 ]. When the fourth no confounding assumption is violated, multiple mediator models can be estimated to take into account the additional mediator variables [ 25 , 61 , 62 , 63 ].
The presence of covariate-exposure, covariate-mediator, exposure-mediator and mediator-mediator interactions can be assessed by adding interaction terms to the statistical models. This is important because the overall effects ignore important information on the direct and indirect effect estimates when statistically significant or clinically relevant interactions are not taken into account [ 28 , 82 ].
Finally, it is important to assess the rare-outcome assumption when using a regression-based estimation approach for the analysis of a mediation model with a binary or time-to-event outcome, as the effect estimates on the odds-ratio scale and hazard-ratio scale only have a causal interpretation when the outcome prevalence is low across all strata of the exposure and mediator variables [ 83 ]. When the rare-outcome assumption is violated it is advised to estimate the effects for models with a binary outcome with log-linear regression and the effects for models with a time-to-event outcome with accelerated failure time models [ 28 , 57 ].
Statistical inference
Over one-third of the papers in this scoping review determined the statistical significance of the indirect effect estimate based on a z -test, which has relatively low power to detect a statistically significant indirect effect [ 35 , 36 ]. Instead, it is recommended to determine the statistical significance of the indirect effect estimate based on a confidence interval that takes into account the nonnormal sampling distribution of the indirect effect estimator, such as the distribution of the product confidence interval, Monte Carlo confidence interval, and bootstrap confidence intervals, as these have higher power to detect a statistically significant indirect effect [ 34 , 36 , 38 , 84 , 85 , 86 ]. Although the bias-corrected bootstrap confidence interval was the most often reported confidence interval in the studies in this scoping review, percentile bootstrap confidence intervals generally perform best in terms of the balance between type I and type II error rates [ 36 , 87 , 88 ].
Relative effect size measures
In addition to the (natural) indirect effect estimates, over one-third of the studies in this scoping review reported the proportion mediated as a relative effect size measure for the mediated effect. Although the proportion mediated has an intuitive interpretation, it does suffer from a few important limitations. First, a previous simulation study showed that the proportion mediated is unstable in samples of less than 500 participants [ 13 ]. In this review, 21 papers with a sample of less than 500 participants estimated the proportion mediated. Second, the estimate of the proportion mediated can be below zero or above one when the mediation model is inconsistent [ 2 , 3 ]. In this situation, the proportion mediated does not have a meaningful interpretation. Third, the estimate of the proportion mediated can be misleading when the underlying effect estimates are small and clinically irrelevant, as the estimate of the proportion mediated can still be large in this situation. Therefore, it is advised to only estimate the proportion mediated when none of the aforementioned situations apply. If the aforementioned situations do apply, it may suffice to only report the natural indirect effect estimate with a confidence interval. However, when the indirect effect is estimated based on variables without a naturally meaningful interpretation, such as variables measured on a Likert scale, researchers may alternatively determine the relative effect size by comparing the standardized indirect effect estimate to Cohen’s d [ 89 , 90 ].
Recommendations for enhancing the uptake of causal mediation analysis
Although most of the seminal articles on causal mediation analysis were published between 2009 and 2012 [ 45 , 46 , 53 , 56 ], and various causal mediation software packages have been developed in the last decade [ 21 , 22 , 23 , 24 , 25 , 26 , 28 ], the uptake of causal mediation analysis in applied research remains relatively low. A first reason for this low uptake might be the high level of technical details in the causal mediation analysis literature [ 20 , 29 ]. To enhance the uptake of causal mediation analysis, Vo et al. [ 29 ] suggested that there is a need for detailed tutorial papers. As binary and time-to-event outcomes are common in epidemiology and causal mediation analysis clarifies the ambiguities that arise when these outcomes are analyzed with traditional mediation analysis, future tutorial papers could demonstrate the application of causal estimators and the interpretation of causal effect estimates based on real-life data for models with non-continuous mediator variables or non-continuous outcome variables. Another potential topic for a tutorial paper could be the demonstration of the importance of testing the plausibility of the causal assumptions, as this review and previous reviews found that most studies fail to address the plausibility of all causal assumptions [ 20 , 29 , 91 ].
A second reason for the low uptake of causal mediation analysis might be that currently available causal mediation software packages facilitate the estimation of causal effects for a limited range of mediation models. The uptake of causal mediation analysis can also be enhanced through the expansion of current software packages and/or the development of new software packages that facilitate causal effect estimation for a wider range of more complicated mediation models, such as models with latent variables and multiple mediator models. To date, only M plus facilitates the estimation of causal effects for mediation models with latent variables and the causal effect estimation for multiple mediator models is only supported by the Mediation and Medflex packages in R [ 23 , 25 , 26 , 27 ]. Also, the causal effect estimation for multilevel and longitudinal mediation models is limitedly supported by the currently available software packages and warrants attention in future software development [ 27 ].
Strengths and limitations
This scoping review assessed the methodological characteristics of mediation analyses published based on observational data. Observational data is common in the field of epidemiology and mediation analysis is becoming an increasingly popular method to analyze observational data. Two previously published reviews also reported that traditional mediation analysis is the most frequently used mediation analysis method, but one of these reviews focused on the analysis of experimental data [ 29 ], and the other on mediation models with time-to-event outcomes [ 20 ]. This scoping review was not restricted to specific types of mediation models, providing insight in the use of mediation analysis methods across a range of model characteristics. Another strength of this review is that it covered a relatively wide range of publication years to gain insight into the uptake of causal mediation analysis in recent years. Based on the current practices observed in this scoping review, we provided recommendations for applied researchers who wish to apply mediation analysis to their data.
A limitation of this study is that the results might not be generalizable to all observational mediation analyses published between 2015 and 2019, as we only searched two databases and the search strategy was limited to the title, abstract and keywords of the papers. Therefore, it is likely that not all observational mediation analyses published between 2015 and 2019 were identified by our search. However, the goal of our paper was to provide insight into the methodological characteristics of mediation analysis methods used to analyze observational data. Even though this scoping review may not have included all observational mediation analyses published between 2015 and 2019, the results demonstrate large heterogeneity in the mediation analysis methods used to analyze observational data. Based on the findings in this scoping review, we were able to provide recommendations to improve the quality of future mediation analyses. Furthermore, compared to the previously published review by Vo and colleagues [ 29 ] who reviewed the methodological characteristics of mediation analysis methods applied in randomized controlled trials, we used a more extensive search term, a longer search period, and we searched both the MEDLINE and EMBASE databases. MEDLINE and EMBASE are two of the largest databases for epidemiological publications and with 174 included papers this is one of the largest reviews on mediation analysis methods so far [ 20 , 29 , 91 , 92 ].
Another limitation is that the studies included in this scoping review might not have been able to report all aspects of their mediation analyses due to journal requirements such as word limits. For example, although the no (unmeasured) confounding assumptions are of critical importance in mediation analysis, the studies in this review generally provided little information on the causal theory underlying the confounder selection. That is, information was generally lacking on the specific pathways that might be confounded by each of the confounders. Journal requirements might therefore partially explain the large heterogeneity in the reporting of mediation analyses observed in this scoping review and in previous reviews [ 20 , 29 , 91 , 93 ]. The transparency in the reporting of future mediation analyses will likely be enhanced by the guideline for the reporting of mediation analyses that was recently published [ 94 ].
Mediation analysis is becoming increasingly popular in the field of epidemiology, as it can be used to gain insight into mechanisms of disease development. Even though causal mediation analysis is the generally preferred method for mediation analysis, we showed that traditional mediation analysis is still frequently applied in practice. We recommend that researchers use causal mediation analysis and assess the plausibility of relevant causal assumptions to ensure the causal interpretation of the direct and indirect effect estimates. Furthermore, the uptake of causal mediation analysis could be enhanced through tutorial papers and the development of software packages that facilitate the estimation of causal effects for relatively complicated mediation models.
Availability of data and materials
The dataset supporting the conclusions of this article is included within the article and its additional files.
Abbreviations
body mass index
controlled direct effect
directed acyclic graph
pure natural indirect effect
pure natural direct effect
preferred reporting items for systematic reviews and meta-analyses
total effect
total natural indirect effect
total natural direct effect
Nguyen TQ, Schmid I, Stuart EA. Clarifying causal mediation analysis for the applied researcher: defining effects based on what we want to learn. Psychol Methods. 2020.
Alwin DF, Hauser RM. The decomposition of effects in path analysis. Am Sociol Rev. 1975:37–47.
MacKinnon DP. Introduction to statistical mediation analysis. New York: Erlbaum; 2008.
Google Scholar
Pearl J, editor Direct and indirect effects. Proceedings of the seventeenth conference on uncertainty in artifical intelligence; 2001: Morgan Kaufmann Publishers Inc.
Li Y, Zhang T, Han T, Li S, Bazzano L, He J, et al. Impact of cigarette smoking on the relationship between body mass index and insulin: longitudinal observation from the Bogalusa heart study. Diabetes Obes Metab. 2018;20(7):1578–84.
Article CAS PubMed PubMed Central Google Scholar
Pechey R, Monsivais P. Socioeconomic inequalities in the healthiness of food choices: exploring the contributions of food expenditures. Prev Med. 2016;88:203–9.
Article PubMed PubMed Central Google Scholar
Wright S. The relative importance of heredity and environment in determining the piebald pattern of guinea-pigs. Proc Natl Acad Sci U S A. 1920;6(6):320.
Wright S. Correlation and causation. J Agric Res. 1921;20:557–80.
Judd CM, Kenny DA. Process analysis - estimating mediation in treatment evaluations. Eval Rev. 1981;5(5):602–19.
Article Google Scholar
Baron RM, Kenny DA. The moderator mediator variable distinction in social psychological-research - conceptual, strategic, and statistical considerations. J Pers Soc Psychol. 1986;51(6):1173–82.
Article CAS PubMed Google Scholar
Hayes AF. Introduction to mediation, moderation, and conditional process analysis: a regression-based approach: Guilford publications; 2017.
MacKinnon DP, Dwyer JH. Estimating mediated effects in prevention studies. Eval Rev. 1993;17(2):144–58.
Mackinnon DP, Warsi G, Dwyer JH. A simulation study of mediated effect measures. Multivar Behav Res. 1995;30(1):41–62.
Holland PW. Causal inference, path analysis and recursive structural equations models. ETS Research Report Series. 1988;1988(1):i–50.
Robins JM, Greenland S. Identifiability and exchangeability for direct and indirect effects. Epidemiology. 1992;3(2):143–55.
MacKinnon DP, Valente MJ, Gonzalez O. The correspondence between causal and traditional mediation analysis: the link is the mediator by treatment interaction. Prev Sci. 2020;21(2):147–57.
Rijnhart JJM, Twisk JWR, Chinapaw MJM, de Boer MR, Heymans MW. Comparison of methods for the analysis of relatively simple mediation models. Contemporary Clinical Trials Communications. 2017;7:130–5.
Rijnhart JJM, Valente MJ, MacKinnon DP, Twisk JWR, Heymans MW. The use of traditional and causal estimators for mediation models with a binary outcome and exposure-mediator interaction. Struct Equ Model Multidiscip J. 2020:1–11.
VanderWeele TJ. Explanation in causal inference: methods for mediation and interaction: Oxford University press; 2015.
Lapointe-Shaw L, Bouck Z, Howell NA, Lange T, Orchanian-Cheff A, Austin PC, et al. Mediation analysis with a time-to-event outcome: a review of use and reporting in healthcare research. BMC Med Res Methodol. 2018;18(1):118.
Discacciati A, Bellavia A, Lee JJ, Mazumdar M, Valeri L. Med4way: a Stata command to investigate mediating and interactive mechanisms using the four-way effect decomposition. Int J Epidemiol. 2019;48(1):15–20.
Emsley R, Liu H. PARAMED: Stata module to perform causal mediation analysis using parametric regression models. 2013.
Muthén BO, Muthén LK, Asparouhov T. Regression and mediation analysis using Mplus. Los Angeles: Muthén & Muthén; 2017.
SAS Institute. User's guide the CAUSALMED procedure. Cary: SAS Institute Inc.; 2018.
Steen J, Loeys T, Moerkerke B, Vansteelandt S. medflex: An R Package for Flexible Mediation Analysis using Natural Effect Models. Journal of Statistical Software. 2017;76(11).
Tingley D, Yamamoto T, Hirose K, Keele L, Imai K. Mediation: R Package for Causal Mediation Analysis. J Stat Software. 2014;59(5).
Valente MJ, Rijnhart JJM, Smyth HL, Muniz FB, Mackinnon DP. Causal mediation programs in R, Mplus, SAS, SPSS, and Stata. Struct Equ Model Multidiscip J. 2020;27(6):975–84.
Valeri L, Vanderweele TJ. Mediation analysis allowing for exposure-mediator interactions and causal interpretation: theoretical assumptions and implementation with SAS and SPSS macros. Psychol Methods. 2013;18(2):137–50.
Vo T, Superchi C, Boutron I, Vansteelandt S. The conduct and reporting of mediation analysis in recently published randomized controlled trials: results from a methodological systematic review. J Clin Epidemiol. 2020;117:78–88.
Article PubMed Google Scholar
Munn Z, Peters MDJ, Stern C, Tufanaru C, McArthur A, Aromataris E. Systematic review or scoping review? Guidance for authors when choosing between a systematic or scoping review approach. BMC Med Res Methodol. 2018;18(1):1–7.
Sobel ME. Asymptotic confidence intervals for indirect effects in structural equation models. Sociol Methodol. 1982;13:290–312.
Sobel ME. Some new results on indirect effects and their standard errors in covariance structure models. Sociol Methodol. 1986;16:159–86.
Stone CA, Sobel ME. The robustness of estimates of total indirect effects in covariance structure models estimated by maximum likelihood. Psychometrika. 1990;55(2):337–52.
MacKinnon DP, Lockwood CM, Hoffman JM, West SG, Sheets V. A comparison of methods to test mediation and other intervening variable effects. Psychol Methods. 2002;7(1):83–104.
Hayes AF, Scharkow M. The relative trustworthiness of inferential tests of the indirect effect in statistical mediation analysis: does method really matter? Psychol Sci. 2013;24(10):1918–27.
Mackinnon DP, Lockwood CM, Williams J. Confidence limits for the indirect effect: distribution of the product and resampling methods. Multivar Behav Res. 2004;39(1):99–128.
Rudolph KE, Goin DE, Paksarian D, Crowder R, Merikangas KR, Stuart EA. Causal mediation analysis with observational data: considerations and illustration examining mechanisms linking neighborhood poverty to adolescent substance use. Am J Epidemiol. 2019;188(3):598–608.
Preacher KJ, Hayes AF. Asymptotic and resampling strategies for assessing and comparing indirect effects in multiple mediator models. Behavioral Research Methods. 2008;40(3):879–91.
Cole DA, Maxwell SE. Testing mediational models with longitudinal data: questions and tips in the use of structural equation modeling. J Abnorm Psychol. 2003;112(4):558–77.
Cohen J, Cohen P, West SG, Aiken LS. Applied multiple regression/correlation analysis for the behavioral sciences. 3rd ed. Mawah: Lawrence Erlbaum Associates, Inc.; 2003.
MacKinnon DP, Lockwood CM, Brown CH, Wang W, Hoffman JM. The intermediate endpoint effect in logistic and probit regression. Clinical Trials. 2007;4(5):499–513.
Rijnhart JJM, Twisk JWR, Eekhout I, Heymans MW. Comparison of logistic-regression based methods for simple mediation analysis with a dichotomous outcome variable. BMC Med Res Methodol. 2019;19(1):19.
Tein JY, MacKinnon DP. Estimating mediated effects with survival data. New developments in psychometrics: Springer; 2003. p. 405–412.
Jiang ZC, VanderWeele TJ. When is the difference method conservative for assessing mediation? Am J Epidemiol. 2015;182(2):105–8.
Pearl J. The causal mediation formula—a guide to the assessment of pathways and mechanisms. Prev Sci. 2012;13(4):426–36.
VanderWeele TJ, Vansteelandt S. Conceptual issues concerning mediation, interventions and composition. Statistics and its Interface. 2009;2(4):457–68.
Holland PW. Statistics and causal inference. J Am Stat Assoc. 1986;81(396):945–60.
Rubin DB. Estimating causal effects of treatments in randomized and nonrandomized studies. J Educ Psychol. 1974;66(5):688–701.
Robins JM. Semantics of causal DAG models and the identification of direct and indirect effects. Oxford Statistical Science Series. 2003:70–82.
Nguyen TQ, Webb-Vargas Y, Koning IM, Stuart EA. Causal mediation analysis with a binary outcome and multiple continuous or ordinal mediators: simulations and application to an alcohol intervention. Struct Equ Model Multidiscip J. 2016;23(3):368–83.
Andrews RM, Didelez V. Insights into the" cross-world" independence assumption of causal mediation analysis. arXiv preprint arXiv:200310341. 2020.
Pearl J, Mackenzie D. The book of why: the new science of cause and effect: basic books; 2018.
Imai K, Keele L, Tingley D. A general approach to causal mediation analysis. Psychol Methods. 2010;15(4):309–34.
Lange T, Vansteelandt S, Bekaert M. A simple unified approach for estimating natural direct and indirect effects. Am J Epidemiol. 2012;176(3):190–5.
Vansteelandt S, Bekaert M, Lange T. Imputation strategies for the estimation of natural direct and indirect effects. Epidemiologic Methods. 2012;1(1):131–58.
Vanderweele TJ, Vansteelandt S. Odds ratios for mediation analysis for a dichotomous outcome. Am J Epidemiol. 2010;172(12):1339–48.
Van der Weele TJ. Causal mediation analysis with survival data. Epidemiology (Cambridge, Mass). 2011;22(4):582.
VanderWeele TJ, Valeri L, Ananth CV. Counterpoint: mediation formulas with binary mediators and outcomes and the “rare outcome assumption”. Am J Epidemiol. 2019;188(7):1204–5.
Vansteelandt S. Commentary: understanding counterfactual-based mediation analysis approaches and their differences. Epidemiology. 2012;23(6):889–91.
Hong G, editor Ratio of mediator probability weighting for estimating natural direct and indirect effects. Proceedings of the American Statistical Association, Biometrics Section; 2010: American Statistical Association Alexandria, VA.
Lange T, Rasmussen M, Thygesen LC. Assessing natural direct and indirect effects through multiple pathways. Am J Epidemiol. 2014;179(4):513–8.
Steen J, Loeys T, Moerkerke B, Vansteelandt S. Flexible mediation analysis with multiple mediators. Am J Epidemiol. 2017;186(2):184–93.
Vansteelandt S, Daniel RM. Interventional effects for mediation analysis with multiple mediators. Epidemiology (Cambridge, Mass). 2017;28(2):258.
Article PubMed Central Google Scholar
Valeri L, VanderWeele TJ. SAS macro for causal mediation analysis with survival data. Epidemiology. 2015;26(2):E23–E4.
Moher D, Liberati A, Tetzlaff J, Altman DG, Group P. Preferred reporting items for systematic reviews and meta-analyses: the PRISMA statement. PLoS Med. 2009;6(7):e1000097.
Tricco AC, Lillie E, Zarin W, O'Brien KK, Colquhoun H, Levac D, et al. PRISMA extension for scoping reviews (PRISMA-ScR): checklist and explanation. Ann Intern Med. 2018;169(7):467–73.
Booth A, Clarke M, Dooley G, Ghersi D, Moher D, Petticrew M, et al. The nuts and bolts of PROSPERO: an international prospective register of systematic reviews. Systematic Reviews. 2012;1(1):2.
Bramer WM, Rethlefsen ML, Kleijnen J, Franco OH. Optimal database combinations for literature searches in systematic reviews: a prospective exploratory study. Systematic Reviews. 2017;6(1):1–12.
Ouzzani M, Hammady H, Fedorowicz Z, Elmagarmid A. Rayyan—a web and mobile app for systematic reviews. Systematic Reviews. 2016;5(1):210.
MacKinnon DP, Krull JL, Lockwood CM. Equivalence of the mediation, confounding and suppression effect. Prev Sci. 2000;1(4):173–81.
O'Rourke HP, MacKinnon DP. Reasons for testing mediation in the absence of an intervention effect: a research imperative in prevention and intervention research. J Stud Alcohol Drugs. 2018;79(2):171–81.
Mood C. Logistic regression: why we cannot do what we think we can do, and what we can do about it. Eur Sociol Rev. 2010;26(1):67–82.
Imai K, Keele L, Yamamoto T. Identification, inference and sensitivity analysis for causal mediation effects. Stat Sci. 2010:51–71.
De Stavola BL, Daniel RM, Ploubidis GB, Micali N. Mediation analysis with intermediate confounding: structural equation modeling viewed through the causal inference lens. Am J Epidemiol. 2015;181(1):64–80.
Mauro R. Understanding LOVE (left out variables error): a method for estimating the effects of omitted variables. Psychol Bull. 1990;108(2):314.
Valente MJ, Pelham WE III, Smyth H, MacKinnon DP. Confounding in statistical mediation analysis: what it is and how to address it. J Couns Psychol. 2017;64(6):659.
Van der Weele TJ. Bias formulas for sensitivity analysis for direct and indirect effects. Epidemiology (Cambridge, Mass). 2010;21(4):540–51.
Gelfand LA, MacKinnon DP, DeRubeis RJ, Baraldi AN. Mediation analysis with survival outcomes: accelerated failure time vs proportional hazards models Front Psychol. 2016;7:423.
PubMed Google Scholar
VanderWeele TJ. Mediation analysis: a practitioner's guide. Annu Rev Public Health. 2016;37:17–32.
Pearl J. Causality. New York: Oxford University Press; 2000.
Kenny DA. Enhancing validity in psychological research. Am Psychol. 2019;74(9):1018.
Bellavia A, Valeri L. Decomposition of the total effect in the presence of multiple mediators and interactions. Am J Epidemiol. 2018;187(6):1311–8.
Greenland S. Interpretation and choice of effect measures in epidemiologic analyses. Am J Epidemiol. 1987;125(5):761–8.
Bollen KA, Stine R. Direct and indirect effects: classical and bootstrap estimates of variability. Sociol Methodol. 1990:115–40.
Preacher KJ, Selig JP. Advantages of Monte Carlo confidence intervals for indirect effects. Commun Methods Meas. 2012;6(2):77–98.
Tofighi D, MacKinnon DP. RMediation: an R package for mediation analysis confidence intervals. Behav Res Methods. 2011;43(3):692–700.
Fritz MS, Mackinnon DP. Required sample size to detect the mediated effect. Psychol Sci. 2007;18(3):233–9.
Fritz MS, Taylor AB, MacKinnon DP. Explanation of two anomalous results in statistical mediation analysis Multivariate Behav Res. 2012;47(1):61–87.
Miočević M, O’Rourke HP, MacKinnon DP, Brown HC. Statistical properties of four effect-size measures for mediation models. Behav Res Methods. 2018;50(1):285–301.
Preacher KJ, Kelley K. Effect size measures for mediation models: quantitative strategies for communicating indirect effects. Psychol Methods. 2011;16(2):93.
Liu S-H, Ulbricht CM, Chrysanthopoulou SA, Lapane KL. Implementation and reporting of causal mediation analysis in 2015: a systematic review in epidemiological studies. BMC Res Notes. 2016;9(1):354.
Hertzog M. Trends in mediation analysis in nursing research: improving current practice. West J Nurs Res. 2018;40(6):907–30.
Cashin AG, Lee H, Lamb SE, Hopewell S, Mansell G, Williams CM, et al. An overview of systematic reviews found suboptimal reporting and methodological limitations of mediation studies investigating causal mechanisms. J Clin Epidemiol. 2019;111:60–8 e1.
Lee H, Cashin AG, Lamb SE, Hopewell S, Vansteelandt S, VanderWeele TJ, ... Henschke N. A Guideline for Reporting Mediation Analyses of Randomized Trials and Observational Studies: The AGReMA Statement. JAMA. 2021;326(11):1045–56.
Download references
Acknowledgements
Not applicable.
This work was supported by the National Institute on Drug Abuse (R37DA09757 to DPM).
Author information
Authors and affiliations.
Department of Epidemiology and Data Science, Amsterdam UMC, Location VU University Medical Center, Amsterdam Public Health Research Institute, PO Box 7057, 1007, MB, Amsterdam, The Netherlands
Judith J. M. Rijnhart, Jos W. R. Twisk & Martijn W. Heymans
Department of Psychology, Arizona State University, Tempe, AZ, USA
Sophia J. Lamp & David P. MacKinnon
Department of Psychology, Center for Children and Families, Florida International University, Miami, FL, USA
Matthew J. Valente
You can also search for this author in PubMed Google Scholar
Contributions
JJMR, JWRT, MWH, DPM, and MJV designed the study. JJMR directed the study implementation, including quality assurance and control. JJMR and MWH designed the study’s analytic strategy. JJMR, SJL, and MJV conducted the literature review. JJMR prepared the draft of the paper. JWRT, MWH, DPM, SJL and MJV helped critically revise the paper. All authors read and approved the final manuscript.
Corresponding author
Correspondence to Judith J. M. Rijnhart .
Ethics declarations
Ethics approval and consent to participate, consent for publication, competing interests.
MWH is an editorial board member of BMC Medical Research Methodology. The authors declare that they have no competing interests.
Additional information
Publisher’s note.
Springer Nature remains neutral with regard to jurisdictional claims in published maps and institutional affiliations.
Supplementary Information
Additional file 1: supplementary appendix 1..
PRISMA-ScR checklist.
Additional file 2: Supplementary appendix 2.
The PubMed and EMBASE search strategies.
Additional file 3: Supplementary appendix 3.
List of papers included in the scoping review.
Additional file 4: Supplementary appendix 4.
Dataset with extracted data.
Additional file 5: Supplementary appendix 5.
Overview of mediation analysis methods used to analyze repeated measurements in the included papers.
Rights and permissions
Open Access This article is licensed under a Creative Commons Attribution 4.0 International License, which permits use, sharing, adaptation, distribution and reproduction in any medium or format, as long as you give appropriate credit to the original author(s) and the source, provide a link to the Creative Commons licence, and indicate if changes were made. The images or other third party material in this article are included in the article's Creative Commons licence, unless indicated otherwise in a credit line to the material. If material is not included in the article's Creative Commons licence and your intended use is not permitted by statutory regulation or exceeds the permitted use, you will need to obtain permission directly from the copyright holder. To view a copy of this licence, visit http://creativecommons.org/licenses/by/4.0/ . The Creative Commons Public Domain Dedication waiver ( http://creativecommons.org/publicdomain/zero/1.0/ ) applies to the data made available in this article, unless otherwise stated in a credit line to the data.
Reprints and permissions
About this article
Cite this article.
Rijnhart, J.J.M., Lamp, S.J., Valente, M.J. et al. Mediation analysis methods used in observational research: a scoping review and recommendations. BMC Med Res Methodol 21 , 226 (2021). https://doi.org/10.1186/s12874-021-01426-3
Download citation
Received : 24 February 2021
Accepted : 21 September 2021
Published : 25 October 2021
DOI : https://doi.org/10.1186/s12874-021-01426-3
Share this article
Anyone you share the following link with will be able to read this content:
Sorry, a shareable link is not currently available for this article.
Provided by the Springer Nature SharedIt content-sharing initiative
- Mediation analysis
- Counterfactuals
- Potential outcomes
- Indirect effect
- Direct effect
- Observational data
BMC Medical Research Methodology
ISSN: 1471-2288
- General enquiries: [email protected]
- Open access
- Published: 08 April 2021
A moderated mediation analysis of the relationship between a high-stakes English test and test takers’ extracurricular English learning activities
- Jing Zhang ORCID: orcid.org/0000-0001-8694-2493 1
Language Testing in Asia volume 11 , Article number: 5 ( 2021 ) Cite this article
10k Accesses
3 Citations
1 Altmetric
Metrics details
This study investigated the relationship between a large-scale and high-stakes English test and test takers’ learning behavior. Specifically, it explored whether and how the National Matriculation English Test (NMET) influenced test takers’ extracurricular English learning activities under the Chinese Mainland educational context. Based on Bandura’s triadic reciprocal determinism theory, this study proposed a distal mediation model and employed covariance-based Structural Equation Modeling to test the model. The data were collected via a cross-sectional survey with 470 test takers. The results showed that test takers’ perceptions of the examination exerted direct and indirect effects on their extracurricular English learning activities, and that test takers’ perceived self-efficacy for self-regulated learning and academic achievement were two important factors mediating the relationship between their perceptions of the test and extracurricular learning. Furthermore, test takers’ perceptions of the exam-approaching have diverse moderating effects on different mediation effects. This study suggests that introducing the triadic reciprocal determinism theory helps understand how an examination influences learning. It also highlights the role of test takers’ perceptions of an examination and their perceived self-efficacy in predicting a test’s impact on learning.
Introduction
This study was conducted under the Chinese Mainland educational background, with a particular focus on Gaokao—the college entrance examination for the entire country. The competition of Gaokao is so fierce that the mass media usually compare the difficulty of taking Gaokao to “thousands of troops crossing one narrow bridge” (Shi & Jia, 2015 ). Additionally, the number of test takers has been increasing in recent years, which reached 10,710,000 in 2020, an increase of 400,000 over last year (Ministry of Education of the People’s Republic of China, 2020 ). Hence, Gaokao is undoubtedly a large-scale and high-stakes test for most test takers in the Chinese Mainland. The current study only focused on the English component of Gaokao—the National Matriculation English Test (NMET).
Despite the importance of the NMET, its impact on teaching and learning has not attracted enough attention (Dong, 2018 ; Zou & Dong, 2014 ). The NMET is designed to help universities select qualified students and to guide teaching and learning in senior high schools (Ministry of Education of the People’s Republic of China, 2017 ). Thus, in this context, the impact of the NMET on teaching and learning deserves scrutiny. In the Chinese Mainland, since the inception of the impact studies of the NMET in 1990, its impact on teaching has been the predominant focus (e.g., Dong, 2014 ; Dong, 2018 ; Li, 1990 ; Qi, 2004 ). However, the impact of the NMET on learning has been under-investigated (Zou & Dong, 2014 ). Test takers are the most important stakeholders of a test (Green, 2013 ; Rea-Dickins, 1997 ) and test takers’ perceptions of the test are of great importance because these exert influence on their learning behavior (Hughes, 1993 ). It is thus reasonable to infer that understanding the mechanism of test’s impact on learning might help improve test takers’ learning. Hence, this study aims to investigate the relationship between the NMET and test takers’ learning, particularly their extracurricular English learning.
Literature review
In the field of language testing, a wealth of studies investigating test impact on learning have reported that test takers engaged in extracurricular English learning activities during test preparation (e.g., Sato, 2019 ; Zhan & Andrews, 2014 ). However, most studies merely focused on traditional test preparation behavior, such as doing past papers (e.g., Xie & Andrews, 2012 ), while only a few studies highlighted the importance of test takers’ extracurricular English learning activities (TEELA) and the relationship between a large-scale and high-stakes examination and TEELA, and even fewer studies specifically addressed the issue of whether such a relationship changes with the exam time approaching.
TEELA is an important type of learning that deserves attention. It refers to the communicatively-oriented English learning activities test takers are engaged in outside the classroom, such as reading English novels or watching TED lectures. Compared with traditional learning activities that are typically assigned and supervised by teachers or schools, TEELA is usually autonomous and somewhat like amusements that might help students to relax from a mountain of schoolwork. TEELA is thus not a test preparation practice per se in a way that test takers work on past examination papers. Extracurricular learning activities are not only an important contributor to students’ academic achievement (e.g., Cooper, Valentine, Nye, & Lindsay, 1999 ) but also a facilitating factor for improving their language skills (e.g., Cao, 2015 ; Huang & Naerssen, 1987 ; Marefat & Barbari, 2009 ; Pan, 2014 ). In the Chinese Mainland, it is also believed that extracurricular English learning activities are instrumental in helping students achieve their long-term learning goals and improving their comprehensive language skills (Cao, 2015 ; Liang, 2011 ). Moreover, NMET test designers also regard developing students’ comprehensive language skills as their supreme goal (Ministry of Education of the People’s Republic of China, 2017 ). Hence, it is warranted to examine whether and how the NMET influences TEELA.
In terms of the relationship between a large-scale and high-stakes examination and TEELA, contradictory conclusions have been gained under various educational contexts. For example, Zhan and Andrews ( 2014 ) conducted a case study in the Chinese Mainland and concluded that undergraduate test takers engaged in TEELA at the early stage of test preparation, and they admitted that they did such activities due to the influence of the examination. On the contrary, Sato ( 2019 ) implemented an exploratory study in Japan and found that senior high school test takers engaged in TEELA due to their interest in English rather than test impact.
Studies investigating whether the relationship between the test and TEELA changes as the exam time approaches are rare. Most research employed univariate techniques such as t tests to examine whether the exam time approaching affects TEELA. For example, Pan ( 2014 ) reported that the frequency of college students’ engaging in TEELA increased as the exam time approached. It appears that although researchers realized the exam time approaching might influence TEELA, its role in moderating the relationship between the test and TEELA has not aroused enough attention.
In the test impact literature, test takers’ perceptions have typically been used as predictors to represent a test. For example, Xie and Andrews ( 2012 ) employed test takers’ perceptions of test design and test use as the predicting variables to examine the relationship between the College English Test and test takers’ test preparation behavior. The present study follows this practice—using test takers’ perceptions of the NMET (TPN) as the predictor, which is defined as test takers’ perceptions of the positive influence that the NMET exerts on their English learning. This definition is inspired by the idea that a well-designed test might motivate test takers to be engaged in learning activities that are beneficial to their long-term learning goals (Green, 2013 ). For students, a well-designed test might mean a test that exerts a good effect on learning. Cheng, Andrews, and Yu ( 2010 ) have used a similar construct to investigate test takers’ perceptions of a newly-introduced test. Nevertheless, the construct was treated as an outcome variable in their research.
Another gap identified in impact studies regarding learning was that most research adopted qualitative methods (e.g., Sato, 2019 ; Zhan & Andrews, 2014 ), with a particular lack of confirmatory studies of mediating factors (Sato, 2019 ). The existing literature suggests that many mediating factors exist on the testing–learning path, and applying qualitative methods enables researchers to identify these factors (Watanabe, 2004 ; Xie, 2015 ). For example, Watanabe ( 2004 ) has summarized five types of mediating factors based on previous research, including test factors, prestige factors, personal factors, micro-context factors, and macro-context factors. However, these factors were under-explored (Xie, 2015 ), meaning that little has been investigated about their “relative importance” (Xie, 2015 , p. 58), their relationships (Sato, 2019 ), and their generalizability to diverse situations. Thus, researchers are encouraged to employ “more sophisticated data collection and analysis methods” (Tsagari & Cheng, 2017 , p. 368). Xie and Andrews ( 2012 ), for example, conducted a mediation analysis and showed that the expectation of success was a good mediator on the path from test taker perceptions of the examination to test preparation behavior. However, in their research, the construct of the expectation of success was measured by the self-efficacy scale, suggesting that self-efficacy might be a good factor mediating the relationship between a test and test takers' learning. The mediating effect of self-efficacy accounting for the impact of test taker perceptions on their learning behavior is thus worth further scrutiny. Additionally, estimation methods of mediation effects employed in the existing impact research, such as the products of coefficients approach, were lack of statistical power (see Data analysis). Consequently, it is necessary to find a new approach to analyzing mediation effects.
Theoretical framework
This study introduced Bandura’s triadic reciprocal determinism (TRD) theory (1986) to explain the process of the NMET’s impact on learning.
TRD theory attempts to explain humans’ learning behavior in the social environment. It proposes that environmental factors, personal factors, and behavior are independent of each other, but they interrelate with and determine each other (Bandura, 1986 ). Environmental factors refer to the external social events that greatly influence individuals, for example, the NMET is an influential environmental factor for test takers; personal factors, such as cognitive, emotional, and motivational factors, play a strong controlling and guiding role in human behavior (Guo & Jiang, 2008 ). The three elements do not always exert equivalent influence on each other, and their influences change due to different circumstances, individuals, and activities.
The TRD model involves three interactions: The interaction between the environment and the person describes that the environment interacts with human beliefs and cognitive competencies (Guo & Jiang, 2008 ). The interaction between the person and behavior refers to the interaction of human thoughts and actions. The interaction between the environment and behavior depicts that the environment influences human behavior, which in turn influences that environment. Thus, based on this model, the NMET, test takers’ factors, and their learning behavior interrelate with each other. Specifically, there are interactions between the NMET and test takers’ belief about the NMET, between test takers’ thoughts and actions, and between test takers’ learning behavior and certain aspects of the NMET. Besides, personal factors have been assumed to be mediating factors between a test and learning behavior (e.g., Watanabe, 2004 ); thus, it might be reasonable to infer that test takers’ perceptions of the NMET exert an impact on the personal factors, and in turn influence their learning behavior.
Within the framework of the TRD theory, Bandura further explored the personal factors. Particularly, he highlights the importance of self-efficacy, a cognitive self-concept of the capabilities that “one can successfully execute the behavior required to produce desired outcomes” (Bandura, 1977 , p. 193), because perceived self-efficacy is helpful in explaining a myriad of phenomena such as “changes in coping behavior produced by different modes of influence” (Bandura, 1982 , p. 122). According to Bandura ( 1982 ), people first form their perceptions of the environment. Based on these perceptions, individuals appraise their efficacy. High self-percept of efficacy may encourage people to deploy their efforts to deal with the demands of the environment and in turn enhance their performance, while low self-percept of efficacy may lead people to maximize the potential difficulties, which in turn jeopardize their performance. Therefore, there is strong reason to suspect that under the context of testing, test takers may first have their perceptions of the test, then evaluate their self-efficacy based on their perceptions, which may finally affect their learning behavior.
Self-efficacy is a multidimensional construct (Bandura, 1986 ), in which perceived self-efficacy for self-regulated learning (PSE-SRL) and academic achievement (PSE-AA) are two strong predictors for student academic learning and performance (Oliveira, Taveira, Porfeli, & Grace, 2018 ; Zimmerman, Bandura, & Martinez-Pons, 1992 ). PSE-SRL refers to the prediction of one’s capabilities to actively and systematically use self-regulatory process to gain the desired learning outcome (Lee, Lee, & Bong, 2014 ). Self-regulated learners display “a high sense of efficacy in their capabilities, which influence their commitment to fulfilling these challenges” (Zimmerman et al., 1992 , p. 664). PSE-AA is defined as the conviction that learners can successfully attain their desired academic achievement (Schunk, 1991 ). A high sense of PSE-AA motivates learners to deploy more efforts, persistence, and intrinsic interest in their learning and performance (Zimmerman et al., 1992 ). Additionally, PSE-SRL has been proved to predict PSE-AA (Lee et al., 2014 ; Zimmerman et al., 1992 ). However, the effects of these two kinds of self-efficacy in terms of improving students’ extracurricular English learning and their mediating effects between testing and learning behavior were under-investigated within the field of language testing. Only Xie and Andrews ( 2012 ) have explored the mediating effect of self-efficacy, but the self-efficacy measure used in their research focused more on motivated learning strategy. Therefore, little attention has been devoted to the mediating role of PSE-SRL and PSE-AA. Thus, this study conducted a mediation analysis to explore the effects of these two types of self-efficacy and their relationship.
Conceptual model and research questions
Based on the TRD theory and related literature, this study proposes that TPN influences test takers’ PSE-SRL and PSE-AA, which in turn affect their TEELA. This process is moderated by test takers’ perceptions of exam-approaching. Specifically, the following conceptual model (Fig. 1 ) depicts the proposed theory:
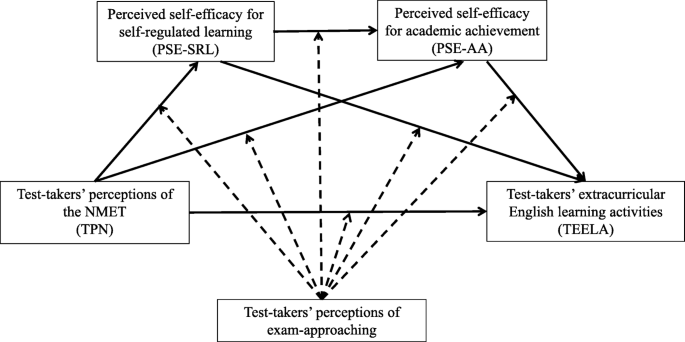
Conceptual model
Three research questions are included in this study:
Does TPN have a direct effect on TEELA?
If this direct effect exists, will it change with the exam time approaching?
On the path from TPN to TEELA,
Does PSE-SRL mediate the relationship between TPN and TEELA?
Does PSE-AA mediate the relationship between TPN and TEELA?
Does the TPN→PSE-SRL→PSE-AA→TEELA path exist?
Will test takers’ perceptions of exam-approaching
Moderate the indirect effect of TPN on TEELA through PSE-SRL?
Moderate the indirect effect of TPN on TEELA through PSE-AA?
Moderate the indirect effect of TPN on TEELA through PSE-SRL and PSE-AA?
Research context and participants
The NMET aims to examine test takers’ language knowledge and use (Ministry of Education of the People’s Republic of China, 2019 ). In terms of language knowledge, test takers are required to master and use English phonetics, vocabulary, grammar, function-notion, and topics that they have learned. In terms of language use, the NMET examines test takers’ ability from four perspectives: listening, reading, writing, and speaking. Table 1 describes the components of the NMET written test paper used in the province where the present study was conducted. All test takers are required to take the written test. On the contrary, the NMET spoken test is separate and optional. Typically, two types of students take this test: students wishing to apply for special majors such as foreign affairs and international law and students wishing to know their spoken English level. Test formats include reading a short passage aloud and answering the examiner’s questions.
This research was conducted in an Eastern province in the Chinese Mainland. From five ordinary senior high schools (Table 2 ) in the capital city of the province, 470 students were randomly selected for this study. Based on Hair Jr., Black, Babin, and Anderson’s ( 2019 ) suggestion, a sample size of 470 is large enough for this study. There is no wide disparity among these schools in terms of teaching quality, school facilities, the minimum score of high school admission, and philosophies of schooling. All five English teachers agreed to include several randomly selected classes in the present study. Besides, random selection within the classes was performed by the author. Table 3 shows the demographic characteristics of these participants.
Instrumentation
A questionnaire (see Appendix ), including four multi-item measures (31 items), was employed to assess the latent constructs in the conceptual model. All measures were revised from other researchers’ scales so that they were originally developed in English. Having been examined and discussed by three experts, all the items were translated into Chinese via the translation–back translation procedure (Brislin, 1970 ). Before the formal data collection, at the end of 2019, a pilot study of 89 senior high school students from one middle school in the same province with the formal survey, was conducted to evaluate the quality of the research design and questionnaire items. No problematic items were identified based on the results of the item analysis.
Test takers’ perceptions of the NMET
TPN was assessed by a nine-item scale adapted based on the “students’ perception subscale” developed by Cheng et al. ( 2010 ) and the NMET syllabus issued in 2019. High TPN score means that test takers believe the NMET can influence their English learning positively. The respondents were asked to choose from a seven-point Likert scale ranging from 1, “strongly disagree”, to 7, “strongly agree”. The Cronbach’s alpha (in the actual administration) for the TPN subscale was .928.
Test takers’ perceived self-efficacy
Two subscales from the Multidimensional Scales of Perceived Self-Efficacy (Bandura, 1989, as cited in Williams & Coombs, 1996 ) were selected and revised for use in the present study: PSE-SRL and PSE-AA. The PSE-SRL subscale was composed of 10 items, measuring test takers’ perceived ability to use diverse self-regulated learning strategies. The PSE-AA subscale consisted of six items assessing test takers’ perceived capability to gain success in six aspects: English vocabulary, grammar, reading, listening, speaking, and writing. Participants rated the strength of their belief on a 7-point scale ranging from 1, “not well at all”, to 7, “very well”. The Cronbach’s alphas of the PSE-SRL and PSE-AA subscales were .952 and .958, respectively.
- Test takers’ extracurricular English learning activities
TEELA was measured by a six-item subscale modified from the “test-related English activities outside school” subscale in the study of Cheng et al. ( 2010 ). Items in the TEELA scale measured test takers’ frequency of engaging in TEELA in the past year. The items were responded to on a 7-point Likert scale with values varying from 1, “never”, to 7, “every time”. The Cronbach’s alpha of this subscale was .940.
Test-takers’ perceptions of exam-approaching
This construct is represented by three grades in high school. The higher the grade, the stronger test takers’ perceptions or senses of the exam-approaching. Because the NMET is held at the end of senior three, the grade 3 students are the closest to the examination. As a consequence, compared with grade 1 and 2 students, grade 3 students face more pressure of Gaokao and spend more time and energy in test preparation (Cao, 2016 ), It is thus reasonable to infer that with the advance of grade, students’ perceptions of the time of testing become increasingly intense.
Data collection
This study involved a cross-sectional survey conducted in the spring of 2020. To guarantee the reliability of the responses and absolute confidentiality, the participants were assured of anonymity, and they were ensured that only the researcher would see their responses. The survey was created and implemented with a widely used tool—WENJUANXING ( http://www.wenjuanxing.com ). One advantage of using WENJUANXING is that no missing data will be generated due to its prior setting (if respondents forget to fill in one item, they will be reminded to complete it; otherwise, they cannot continue with the questionnaire). Students who completed and successfully submitted the questionnaire joined in an online lucky draw immediately after their submission, and several types of awards were provided as a token of gratitude from the author.
Data analysis
Analytic strategy.
This study employed the covariance-based Structural Equation Modeling (CB-SEM) technique to answer the research questions with Amos 24. CB-SEM is typically used to test process models developed by a theory (Hayes, 2009 ; Lei & Wu, 2007 ). When using CB-SEM, investigators do not find a model to fit the data (Kline, 2016 ), but test a theory via specifying a model depicting the relationships between the constructs that are described in that theory, with the constructs measured by valid observed variables (Hair Jr. et al., 2019 ). In doing so, researchers can “evaluate the validity of substantive theories with empirical data” (Lei & Wu, 2007 , p. 33), which in turn helps develop a theory (Anderson & Gerbing, 1988 ). Hence, the present study employed CB-SEM to reveal what happened in the process of the NMET exerting influence on learning.
The maximum likelihood estimation method was employed because it has been known to gain more robust parameter estimates compared with other estimators (e.g., generalized least squares) (Curran, West, & Finch, 1996 ), even when the observed variables were not on a multivariate normal distribution (Iacobucci, 2010 ).
To answer the research questions, this study administered three analyses. Firstly, confirmatory factor analysis (CFA) was performed to assess the measurement model. Secondly, mediation analysis was conducted employing bootstrapping (Hayes, 2009 ) to answer research questions 1 and 2. Finally, the subgroup method and bootstrapping were applied to conduct a moderated mediation analysis to answer research question 3. All the bootstrapping procedures were conducted with 5000 bootstrap samples (Hayes, 2009 ).
Effect sizes were also discussed. Hedges’ g was calculated to gauge how different groups of test takers varied (Ellis, 2010 ). Besides, Pearson product moment correlation coefficient ( r ) and coefficient of multiple determination ( R 2 ) were applied to measure the strength of the relationships between constructs (Ellis, 2010 ).
Mediation analysis
A distal mediation model (Fletcher, 2006 ) was developed in this study, as illustrated in Fig. 2 . a1 and a2 represent the path coefficients from TPN to PSE-SRL and PSE-SRL to TEELA , respectively. b1 and b2 represent the path coefficients from TPN to PSE-AA and PSE-AA to TEELA , respectively. c is the path coefficient from PSE-SRL to PSE-AA . d is the path coefficient from TPN to TEELA , representing the direct effect of TPN on TEELA . Three specific indirect effects (SIE) are included in this model: The product of a1 and a2 represents the mediation effect of TPN on TEELA through PSE-SRL (SIE 1) . The product of b1 and b2 represents the indirect effect of TPN on TEELA via PSE-AA (SIE 2) . The product of a1 , c , and b2 represents the distal mediation effect of TPN on TEELA through PSE-SRL and PSE-AA (SIE 3) . The total indirect effect is quantified as SIE 1 + SIE 2 + SIE 3, while the total effect is quantified as SIE 1 + SIE 2 + SIE 3 + d .
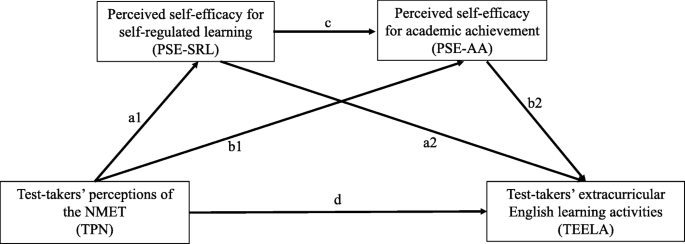
Distal mediation model
The assessment of such a process model is mediation analysis, which allows researchers to understand by what means a predicting variable exerts its influence on an outcome variable (Preacher, Rucker, & Hayes, 2007 ). The mediation effect or indirect effect deserves proper attention, otherwise, “the relationship between two variables of concern may not be fully considered” (Raykov & Marcoulides, 2006 , p. 7).
Diverse methods can be used to gauge the magnitude of indirect effects. Baron and Kenny’s ( 1986 ) causal steps approach has been the most widely used one (Hayes, 2009 ; MacKinnon, Lockwood, & Williams, 2004 ). However, it has been criticized for the lowest statistical power (Fritz & MacKinnon, 2007 ; Hayes, 2009 ), and it is only applicable to the simple mediation model (Preacher et al., 2007 ). As a consequence, investigators usually adopt the Sobel test as a “supplement” (Hayes, 2009 , p. 6) to the causal steps approach. Nevertheless, both of the causal steps approach and Sobel test are based on the premise that the product of a1 and a2 (or b1 and b2 ) is normally distributed, which is difficult to achieve (Bollen & Stine, 1990 ; Preacher et al., 2007 ; Stone & Sobel, 1990 ). Thus, the present study introduced a cutting-edge technique—bootstrapping (Bollen & Stine, 1990 ; Hair Jr. et al., 2019 ; Hayes, 2009 ; Preacher et al., 2007 ) to assess mediation effects, which does not require the assumption of normal distribution (Hayes, 2009 ; Preacher et al., 2007 ).
Two forms of bootstrapping were adopted in this study: naive bootstrapping (Yung & Bentler, 1996 ) and Bollen–Stine bootstrapping (Bollen & Stine, 1992 ). The former was used to conduct a mediation analysis (Hayes, 2009 ; Preacher et al., 2007 ), and the latter was applied to modify the enlarged χ 2 due to multivariate nonnormality (Enders, 2005 ).
Moderated mediation
When the effect of an independent variable on a dependent variable varies due to different levels of a third variable, this variable is called a moderator (Baron & Kenny, 1986 ; Edwards & Lambert, 2007 ; James & Brett, 1984 ). As mediation analysis has aroused considerable attention, many researchers show interest in the condition under which an indirect effect occurs, which is thus referred to as conditional indirect effects (Preacher et al., 2007 ) or moderated mediation (James & Brett, 1984 ).
The most widely used method to examine moderated mediation is to analyze the mediation effect separately at each level of the moderator (Fabrigar & Wegener, 2014 ), which is called the subgroup approach (Edwards & Lambert, 2007 ). Following Preacher et al.’s ( 2007 ) suggestion, within each subgroup (grades 1, 2, and 3), mediation effects were estimated with the bootstrapping procedure.
Data examination
To ensure the quality of CFA, outliers and distributional assumptions were examined first (Jackson, Gillaspy Jr., & Purc-Stephenson, 2009 ). Seven cases were judged to be outliers based on Mahalanobis d square values (Byrne, 2016 ) and were deleted from further analysis. Then multivariate normality was examined, which is the prerequisite of the maximum likelihood estimation (Byrne, 2016 ; Curran et al., 1996 ). Although all the observed variables exhibited univariate normality, the critical ratio of multivariate kurtosis value was above 5.00 (c.r. = 99.291), indicating that the data were multivariate nonnormal (Bentler, 2005 ), which may mislead the researcher to reject the correct model (Curran et al., 1996 ; Lei & Wu, 2007 ). Byrne ( 2016 ) thus recommended that researchers “correct the test statistic, rather than use a different mode of estimation” (p. 124). Hence, Bollen–Stine bootstrapping was applied to re-estimate chi-square and standard error (Bollen & Stine, 1992 ; Enders, 2005 ; Lei & Wu, 2007 ), which might help “gain insight into the behavior of the test statistic with nonnormal data” (Bollen & Stine, 1992 , p. 229).
Measurement model
Before analyzing the structural model, the measurement model should be carefully tested to guarantee that all the observed variables reflect the desired latent constructs (Anderson & Gerbing, 1988 ; Jackson et al., 2009 ) and to determine how well the theoretically specified factor structures fit the sample data (Hair Jr. et al., 2019 ).
Following Hair Jr. et al.’s suggestion ( 2019 ), before formally assessing the measurement model, the diagnostic information from a preliminary CFA was used to modify the model slightly and to improve the quality of the model. Five problematic indicators (see Appendix ) were identified. They exhibited the possibility of cross-loadings and error term correlations, which “would be inconsistent with the theoretical basis of CFA and SEM in general” (Hair Jr. et al., 2019 , p. 678). After carefully considering the face validity and discussing with experts many times, the author decided to delete the five indicators from further analysis. The following section reported the results of assessing measurement model validity, including fit and construct validity.
Firstly, the fit validity was examined. Following Hair Jr. et al.’s ( 2019 ) and Jackson et al.’s ( 2009 ) suggestions, this study reported the following fit indices: chi-square value, relative chi-square ( χ 2 /df), root mean square error of approximation (RMSEA), Tucker Lewis Index (TLI), and comparative fit index (CFI). A relative chi-square of 3.0 or less is considered good, RMSEA values of lower than .08 are associated with good fitting, and TLI and CFI values that approach 1.0 are considered good (Hair Jr. et al., 2019 ). The model with 26 measured variables (Fig. 3 ) yielded a Bollen–Stine χ 2 of 424.274 with 293 degrees of freedom, a relative chi-square of 1.45, an RMSEA of .03, a TLI of .99, and a CFI of .99, which were highly suggestive that the specified factor structure fit the sample data reasonably well.
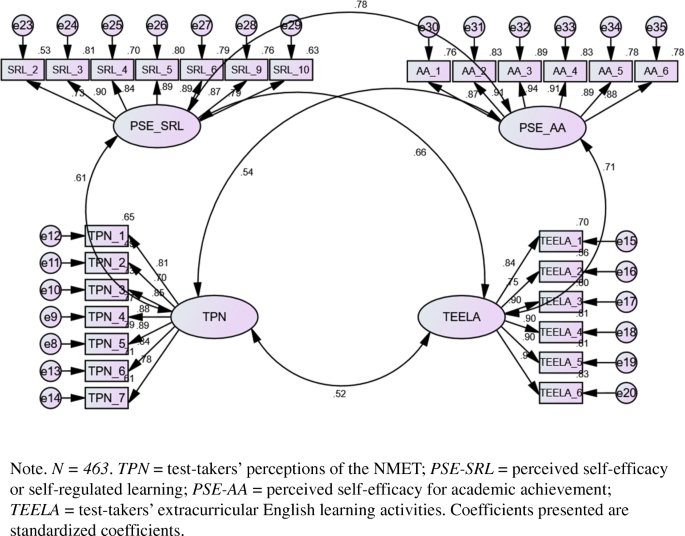
Then, the construct validity was evaluated (Table 4 ), which was the main target of CFA (Hair Jr. et al., 2019 ). All the standardized factor loadings were above .50 and significant ( p < .001), meaning that the items were ideally convergent on their corresponding latent construct (Hair Jr. et al., 2019 ). Besides, all the AVE values were above .50, which was suggestive of adequate convergence (Hair Jr. et al., 2019 ). Further, all the SMC values were above .36, indicating that all the items were reliable (Fornell & Larcker, 1981 ). The composite reliability of greater than .70 rendered enough evidence of good reliability, which suggested appropriate internal consistency within every construct (Hair Jr. et al., 2019 ). Table 5 contains the result of testing the discriminant validity. Following Hair Jr. et al.’s ( 2019 ) suggestion, the discriminant validity was assessed by comparing “the AVE values for any two constructs with the square of the correlation estimate between these two constructs” (p. 677). Thus, the square roots of AVEs were calculated and compared with correlation estimates. All square roots of AVEs were greater than the corresponding Pearson correlation coefficients, indicating that every construct was distinct from each other.
Overall, the results of the CFA showed that the specified measurement model fit well with the sample data, which provided a basic and vital premise for the subsequent structural model analysis (Hair Jr. et al., 2019 ).
Structural model
This section summarized the results of testing the proposed structural theory, which focused on examining the overall structural model fit and the hypothesized structural relationships between constructs. The structural model yielded a Bollen–Stine χ 2 of 424.274 with 293 degrees of freedom, a relative chi-square of 1.45, an RMSEA of .03, a TLI of .99, and a CFI of .99, indicating that the hypothesized structure adequately fit the observed covariance matrix.
Figure 4 illustrates the standardized path estimates and R 2 of the hypothesized model. All the path coefficients were statistically significant ( p < .05), indicating that all hypothesized relationships between constructs were supported. The R 2 for TEELA was .54, suggesting that the structural model explained 54% of the variance in TEELA. Table 6 summarizes the results of the mediation analysis. Five thousand bootstrapping with 95% confidence revealed that the direct path from TPN to TEELA was statistically significant ( B = .179; p < .01). Additionally, TPN had an indirect, statistically significant, positive effect on TEELA via PSE-SRL (SIE 1) ( B = .151; p < .01) or PSE-AA (SIE 2) ( B = .062; p < .05). Besides, TPN also had an indirect, statistically significant, positive relationship with TEELA via PSE-SRL and PSE-AA (SIE 3) ( B = .252; p < .001). All of the bootstrapping confidence interval ranges did not include zero, thus further proving that TPN had direct and indirect effects on TEELA, which also indicated the hypothesized model was a partial mediation model (Hair Jr. et al., 2019 ).
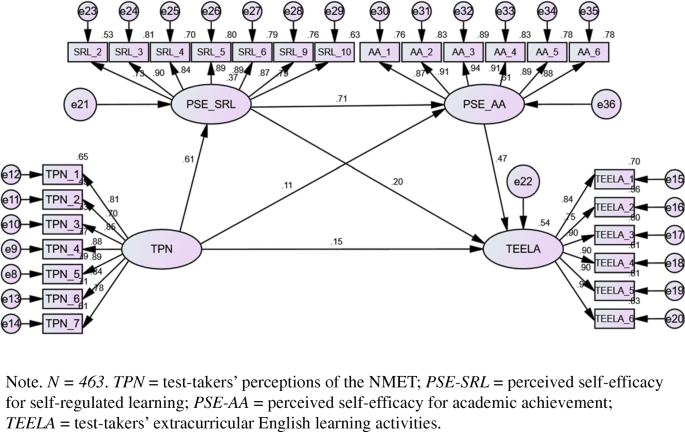
Structural Equation Modeling of the Hypothesized Model with Standardized Coefficients and R²
Finally, all possible pairwise comparisons among the three SIEs were examined to explore their relative importance, showing that only SIE 2 and SIE 3 was significantly different (SIE diff = − .191; p = .000), while there was no statistically significant difference between SIE 1 and 3 (SIE diff = − .102; p = .215), SIE 1 and 2 (SIE diff = − .089; p = .219).
Moderated mediation analysis
As shown in Table 7 , the moderated mediation analysis revealed that the total indirect effect and SIE 3 were statistically significant within each grade. However, neither SIE 1 nor SIE 2 was significant except for SIE 1 in grade 3 ( B = .335; p < .001). The SIE comparison within each grade showed that there was no significant difference between SIE 1 and SIE 2 in three grades. SIE 1 and SIE 3 differed significantly (SIE diff = − .345 and .205, respectively; p < .05) in grades 1 and 3. SIE 2 and SIE 3 differed significantly (SIE diff = .364; p < .001) in grade 1.
Table 8 summarizes the results of the comparison of the indirect and direct effects among three grades. Despite no significant difference existing among the three grades in terms of the direct effect and total indirect effect, grades 1 and 3 differed significantly in terms of SIE 1 and SIE 3 (SIE diff = − .307 and .242, respectively; p < .05). The effect sizes were medium for the difference in SIE 1 (Hedges’ g = .224) and small for that in SIE 3 (Hedges’ g = .129).
Research question 1 asks: “Does TPN have a direct effect on TEELA?” This study shows that TPN has a direct and positive effect on TEELA, suggesting that test takers who believe that the more positive impact the NMET has on their English learning, the more frequently they participate in extracurricular English learning activities. This is consistent with Cheng et al.’s ( 2010 ) finding that students who believed that the test had positive effects on their learning tended to engage in extracurricular English learning activities more frequently than those who held the opposite belief. Besides, this finding also partially coincides with Zhan and Andrews’ ( 2014 ) conclusion that the College English Test drove test takers to engage in out-of-class English learning activities. Based on Cohen’s ( 1988 ) benchmark, TPN is closely related to TEELA ( r = .521, large effect size), but the path coefficient from TPN to TEELA is small ( β = .145; p < .01), indicating that there exist mediating factors between the two constructs, which also suggests that educators should attach great importance to test takers’ perceptions of a test due to its potential in predicting and facilitating their extracurricular learning behavior. Specifically, test designers should communicate with test takers effectively and regularly. In doing so, they can understand test takers’ ideas and accordingly provide helpful suggestions with students to guide their extracurricular learning, which may ultimately facilitate their academic achievement and language skills.
Research question 1 also asks: “If this direct effect exists, will it change with the exam time approaching?” Results show that the direct effect of TPN on TEELA does not change as the exam time approaches (Table 8 ). On the other hand, the indirect effect of TPN on TEELA via PSE-SRL and PSE-AA (SIE 3) decreases as the exam time is imminent (Table 7 ). This finding is consistent with Zhan and Andrews’s ( 2014 ) conclusion that the frequency of college students participating in TEELA dropped as the exam time approached. However interestingly, the indirect effect of TPN on TEELA via PSE-SRL (SIE 1) increases with the exam time approaching. These findings indicate that test takers’ perceptions of exam-approaching plays a complex moderating role in the relationship between the test and extracurricular learning. Specifically, the exam time approaching exerts different influences on the direct and indirect effects of TPN on TEELA. Further investigations are thus needed to explore the moderating role of the exam time approaching.
Research question 2 is about how TPN exerts influence on TEELA. In this study, all the three mediation effects are statistically significant, indicating that PSE-SRL and PSE-AA might be useful and important mediators to explain how TPN affects TEELA, which is helpful in understanding the mechanisms of the test impact process. However, the standardized effect size of TPN→PSE-AA→TEELA (SIE 2) path is very small ( β = .050; p < .05), and this path is not significant in three grades (Table 7 ), indicating that PSE-AA might not serve as an independent mediator to account for the TPN–TEELA relationship.
The SIE comparison shows that there is a significant difference between SIE 2 and SIE 3, suggesting that the SIE 3 path might be more important than the SIE 2 path when explaining how TPN affects TEELA. Specifically, test takers believing an examination influences their learning positively tend to have a high sense of self-regulated learning efficacy, driving them to take diverse self-regulated learning strategies, which in turn motivates them to be more confident about their capabilities to gain academic success and finally engage in out-of-class English learning activities frequently. On the SIE 3 path, the PSE-SRL is predictive of PSE-AA ( β = .713; p < .001) and the effect size of the strength of their relationship is large ( r = .778). Namely, learners with higher PSE-SRL tend to have higher PSE-AA, which suggests that educators should pay great attention to the importance of student PSE-SRL. This finding is consistent with Zimmerman et al.’s ( 1992 ) conclusion that PSE-SRL was predictive of PSE-AA ( β = .512; p < .05).
The specified model explains 54% of the variance in TEELA, representing a large effect size, which shows that the selected factors make a significant contribution to TEELA. Besides, the hypothesized model is a partial mediation one, indicating that there might be other mediators on the path from TPN to TEELA, which coincides with Xie and Andrews’s ( 2012 ) conclusion that there were other mediating factors on the path from testing to learning. Further research is thus needed to explore other mediators (e.g., learner interest or test takers’ anxiety) explaining how an examination affects extracurricular learning.
Research question 3 is concerned with the moderating effect of test takers’ perceptions of exam-approaching. According to the moderated mediation analysis, although there is no significant difference in SIE 2 among three grades, grades 1 and 3 exhibit significant differences in the SIE 1 and SIE 3 (Table 8 ), suggesting that with the advance of grade, SIE 1 and SIE 3 change (Table 7 ), in which SIE 1 increases moderately (Hedges’ g = .224, medium effect size) and SIE 3 decreases slightly (Hedges’ g = .129, small effect size). Specifically, as exam time approaches, test takers who believe the NMET exerts a positive impact on their English learning are more confident about their ability to self-regulate learning strategically, which in turn motivates them to engage in extracurricular English learning activities more frequently. This is partially consistent with Zimmerman and Martinez-Pons’ ( 1990 ) finding that learners with higher PSE-SRL used learning strategies much greater than those with lower PSE-SRL. Additionally, the TPN→PSE-SRL→TEELA path is significant only in grade 3, suggesting that learners gradually become self-regulated with the exam approaching, which in turn motivates them to adopt diverse learning strategies. Further investigations, particularly longitudinal studies, are thus recommended to explore the mediating role of the PSE-SRL on the path from a test to test takers’ learning behavior.
On the other hand, the TPN→PSE-SRL→PSE-AA→TEELA path is always statistically significant across the three grades, suggesting that this path might be the most effective one when explaining how TPN influences TEELA. As mentioned earlier, the effect of this path decreases with exam time approaching, which may be because students invest more and more energy in traditional test preparation activities as the exam time is imminent. More studies are still needed to explore why the strength of the relationship between TPN and TEELA via PSE-SRL and PSE-AA became weaker as the exam time approached.
This study was the initial effort to address the issue of whether, how, and when the NMET affects TEELA. The proposed model fit the obtained data reasonably well and explained a large proportion of the variance in TEELA, indicating that introducing the TRD theory provides enlightenment for understanding the mechanism of test’s impact on learning. Additionally, this study provides empirical evidence for the hypothesis that many mediating factors might exist on the testing–learning path. The mediation effects of these mediators might diversify with the exam time approaching, which confirms that the mechanism of test impact is a highly complex process (Tsagari & Cheng, 2017 ) that calls for further investigation.
There were several limitations in this study. Firstly, this was a cross-sectional research under the educational context of the Chinese Mainland, and all the participants were from ordinary high schools. Thus, it should be cautious when generalizing the results to different educational settings. Secondly, this study gauged the effect sizes via Cohen’s ( 1988 ) benchmarks, which should be the last choice when discussing effect sizes (Ellis, 2010 ). Durlak ( 2009 ) once pointed that rather than applying Cohen’s benchmarking effect sizes as iron-clad criteria, researchers should examine the effect sizes obtained in prior relevant studies. However, in the test’s impact literature, there is not enough previous related research to refer to when discussing effect sizes. Conducting more quantitative studies concerning test’s impact on learning is thus warranted to help other investigators better understand the practical importance of the factors of concern. Finally, all data were from a self-reported questionnaire. It might be better to triangulate the findings with various techniques, which may further enrich the findings.
Availability of data and materials
The datasets analyzed during the current study are available from the corresponding author on reasonable request.
Abbreviations
Average variance extracted
Covariance-based structural equation modeling
Confirmatory factor analysis
Comparative fit index
National Matriculation English Test
Perceived self-efficacy for academic achievement
Perceived self-efficacy for self-regulated learning
Root mean square error of approximation
Standard error
Specific indirect effect
Squared multiple correlations
Standardized
Tucker Lewis Index
Triadic reciprocal determinism theory (Bandura, 1986)
Unstandardized
Anderson, J. C., & Gerbing, D. W. (1988). Structural equation modeling in practice: a review and recommended two-step approach. Psychological Bulletin , 103 (3), 411–422.
Article Google Scholar
Bandura, A. (1977). Self-efficacy: Toward a unifying theory of behavioral change. Psychological Review , 84 , 191–215.
Bandura, A. (1982). Self-efficacy mechanism in human agency. American Psychologist , 37 (2), 122–147.
Bandura, A. (1986). Social foundations of thought and action: a social cognition theory . Englewood Cliffs, NJ: Prentice-Hall.
Google Scholar
Baron, R. M., & Kenny, D. A. (1986). The moderator-mediator variable distinction in social psychological research: Conceptual, strategic, and statistical considerations. Journal of Personality and Social Psychology , 51 , 1173–1182.
Bentler, P. M. (2005). EQS 6 Structural Equations Program Manual . Encino, CA: Multivariate Software.
Bollen, K. A., & Stine, R. (1990). Direct and indirect effects: Classical and bootstrap estimates of variability. Sociological Methodology , 20 , 115–140.
Bollen, K. A., & Stine, R. A. (1992). Bootstrapping goodness-of-fit measures in structural equation models. Sociological Methods & Research , 21 (2), 205–229. https://doi.org/10.1177/0049124192021002004 .
Brislin, R. W. (1970). Back-translation for cross-cultural research. Journal of Cross Cultural Psychology , 1 (3), 185–216.
Byrne, B. (2016). Structural equation modeling with AMOS: Basic concepts, applications, and programming , (3rd ed., ). New York, NY: Routledge.
Book Google Scholar
Cao, D. (2016). A reflection on senior high school student extracurricular English learning activities. New Education Era Electronic Journal , 22 , 60–60.
Cao, W. (2015). A preliminary discussion concerning senior high school student extracurricular English learning activities. Middle School Curriculum Guidance , 9 , 117–118.
Cheng, L., Andrews, S., & Yu, Y. (2010). Impact and consequences of school-based assessment (SBA): Students’ and parents views of SBA in Hong Kong. Language Testing , 28 (2), 221–249.
Cohen, J. (1988). Statistical power analysis for the behavioral sciences , (2nd ed., ). Hillsdale, NJ: Lawrence Erlbaum.
Cooper, H., Valentine, J. C., Nye, B., & Lindsay, J. J. (1999). Relationships between five after-school activities and academic achievement. Journal of Educational Psychology , 91 (2), 369–378.
Curran, P. J., West, S. G., & Finch, J. F. (1996). The robustness of test statistics to nonnormality and specification error in confirmatory factor analysis. Psychological Methods , 1 (1), 16–29.
Dong, L. (2014). A study of the washback effect of the NMET in Beijing on English language teaching and learning in the senior middle school (Doctoral dissertation). Retrieved from CNKI.
Dong, M. (2018). NMET washback on high school English classroom teaching. Basic Foreign Language Education , 20 , 25–32.
Durlak, J. (2009). How to select, calculate, and interpret effect sizes. Journal of Pediatric Psychology , 34 (9), 917–928.
Edwards, J. R., & Lambert, L. S. (2007). Methods for integrating moderation and mediation: A general analytical framework using moderated path analysis. Psychological Methods , 12 , 1–22.
Ellis, P. (2010). The essential guide to effect sizes: Statistical power, meta-analysis, and the interpretation of research results . Cambridge: Cambridge University Press.
Enders, C. K. (2005). An SAS Macro for implementing the modified Bollen-Stine bootstrap for missing data: Implementing the bootstrap using existing structural equation modeling software. Structural Equation Modeling , 12 (4), 620–641.
Fabrigar, L. R., & Wegener, D. T. (2014). Exploring causal and noncausal hypotheses in nonexperimental data. In H. T. Reis, & C. M. Judd (Eds.), Handbook of research methods in social and personality psychology , (2nd ed., pp. 936–990). Cambridge: Cambridge University Press.
Fletcher, T. (2006). Methods and approaches to assessing distal mediation [Paper presentation]. In 66th annual meeting of the Academy of Management . Atlanta, GA: United States.
Fornell, C., & Larcker, D. F. (1981). Evaluating structural equation models with unobservable variables and measurement error. Journal of Marketing Research , 18 (1), 39–50. https://doi.org/10.2307/3151312 .
Fritz, M. S., & MacKinnon, D. P. (2007). Required sample size to detect the mediated effect. Psychological Science , 18 , 233–239.
Green, A. (2013). Washback in language assessment. International Journal of English Studies , 13 (2), 39–51.
Guo, B., & Jiang, F. (2008). Self-efficacy theory and it’s application . Shanghai: Shanghai Educational Publishing House.
Hair Jr., J. F., Black, W. C., Babin, B. J., & Anderson, R. E. (2019). Multivariate data analysis , (8th ed., ). UK: Cengage Learning.
Hayes, A. F. (2009). Beyond Baron and Kenny: Statistical mediation analysis in the New Millennium. Communication Monographs , 76 (4), 408–420.
Huang, X. H., & Naerssen, M. V. (1987). Learning strategies for oral communication. Applied Linguistics , 8 (3), 287–307.
Hughes, A. (1993). Backwash and TOEFL 2000 . Unpublished manuscript. Reading, U.K.: University of Reading.
Iacobucci, D. (2010). Structural equations modeling: Fit indices, ample size, and advanced topics. Journal of Consumer Psychology , 20 , 90–98. https://doi.org/10.1016/j.jcps.2009.09.003 .
Jackson, D. L., Gillaspy Jr., J. A., & Purc-Stephenson, R. (2009). Reporting practices in confirmatory factor analysis: An overview and some recommendations. Psychological methods , 14 (1), 6–23. https://doi.org/10.1037/a0014694 .
James, L. R., & Brett, J. M. (1984). Mediators, moderators, and tests for mediation. Journal of Applied Psychology , 69 , 307–321.
Kline, R. B. (2016). Principles and practice of structural equation modeling , (4th ed., ). New York: The Guilford Press.
Lee, W., Lee, M.-J., & Bong, M. (2014). Testing interest and self-efficacy as predictors of academic self-regulation and achievement. Contemporary Educational Psychology , 39 , 86–99.
Lei, P., & Wu, Q. (2007). Introduction to structural equation modeling: Issues and practical considerations. Educational Measurement: Issues and Practice, fall , (pp. 33–43).
Li, X. (1990). How powerful can a language test be? The MET in China. Journal of Multilingual and Multicultural Development , 11 , 393–404.
Liang, G. (2011). A study on effectively promoting student extracurricular English learning activities. Chinese and Foreign Education Research , 3 , 25–26.
MacKinnon, D. P., Lockwood, C. M., & Williams, J. (2004). Confidence limits for the indirect effect: Distribution of the product and resampling methods. Multivariate Behavioral Research , 39 (1), 99–128.
Marefat, F., & Barbari, F. (2009). The relationship between out-of-class language learning strategy use and reading comprehension ability. Porta Linguarum , 12 , 91–106.
Ministry of Education of the People’s Republic of China. (2017). The reply to the NO. 5574 proposal submitted in the fifth session of the 12th National People’s Congress. http://www.moe.gov.cn/jyb_xxgk/xxgk_jyta/jyta_jijiaosi/201712/t20171219_321937.html . Accessed 12 Feb 2020.
Ministry of Education of the People’s Republic of China. (2019). The national unified syllabus of Gaokao in 2019. http://gaokao.neea.edu.cn/html1/report/19012/5951-1.htm . Accessed 24 Jan 2021.
Ministry of Education of the People’s Republic of China. (2020). Making the best preparation for the 2020 GaoKao with the highest standard and the most stringent measures. http://www.gov.cn/xinwen/2020-07/02/content_5523462.html . Accessed 11 July 2020.
Oliveira, I. M., Taveira, M. C., Porfeli, E. J., & Grace, R. C. (2018). Confirmatory study of the Multidimensional Scales of Perceived Self-Efficacy with children. Universitas Psychologica , 17 (1), 1–12. https://doi.org/10.11144/Javeriana.upsy17-4.csms .
Pan, Y. C. (2014). Learner washback variability in standardized exit tests. The Electronic Journal for English as a Second Language , 18 (2), 1–30.
Preacher, K. J., Rucker, D. D., & Hayes, A. F. (2007). Addressing moderated mediation hypotheses: Theory, methods and prescriptions. Multivariate Behavioral Research , 42 , 185–227.
Qi, L. (2004). Has a high-stakes test produced the intended changes? In L. Cheng, Y. Watanabe, & A. Curtis (Eds.), Washback in Language Testing: Research Context and Methods , (pp. 171–190). New Jersey: Lawrence Erlbaum Associates.
Raykov, T., & Marcoulides, G. A. (2006). A first course in structural equation modeling , (2nd ed., ). New Jersey: Lawrence Erlbaum Associates.
Rea-Dickins, P. (1997). So, why do we need relationships with stakeholders in language testing? A view from the UK. Language Testing , 14 (3), 304–314.
Sato, T. (2019). An investigation of factors involved in Japanese students’ English learning behavior during test preparation. Language Testing and Assessment , 8 (1), 69–95.
Schunk, D. H. (1991). Self-efficacy and academic motivation. Educational Psychologist , 26 , 207–231.
Shi, Y., & Jia, D. (2015). Gao kao [A documentary]. In Zhongshi Media Corporation . Beijing Zhongshi Beijing Film and Television Production: Company.
Stone, C. A., & Sobel, M. E. (1990). The robustness of total indirect effects in covariance structure models estimated with maximum likelihood. Psychometrika , 55 , 337–352.
Tsagari, D., & Cheng, L. (2017). Washback, impact, and consequences revisited. In E. Shohamy, I. G. Or, & S. May (Eds.), Language Testing and Assessment , (3rd ed., pp. 359–372). Cham, Switzerland: Springer International Publishing AG.
Chapter Google Scholar
Watanabe, Y. (2004). Methodology in washback studies. In L. Cheng, Y. Watanabe, & A. Curtis (Eds.), Washback in Language Testing: Research Context and Methods , (pp. 19–36). New Jersey: Lawrence Erlbaum Associates.
Williams, J. E., & Coombs, W. T. (1996). An analysis of the reliability and validity of Bandura’s multidimensional scales of perceived self-efficacy [Paper presentation]. Annual Meeting of the American Educational Research Association . New York: NY, United States.
Xie, Q. (2015). Do component weighting and testing method affect time management and approaches to test preparation? A study on the washback mechanism. System , 50 , 56–68.
Xie, Q., & Andrews, F. (2012). Do test design and uses influence test preparation? Testing a model of washback with Structural Equation Modeling. Language Testing , 30 (1), 49–70.
Yung, Y.-F., & Bentler, P. M. (1996). Bootstrapping techniques in analysis of mean and covariance structures. In G. A. Marcoulides, & R. E. Schumacker (Eds.), Advanced structural equation modeling: Issues and techniques , (pp. 195–226). New Jersey: Lawrence Erlbaum Associates.
Zhan, Y., & Andrews, S. (2014). Washback effects from a high-stakes examination on out-of-class English learning: Insights from possible self theories. Assessment in Education: Principles, Policy & Practice , 21 (1), 71–89. https://doi.org/10.1080/0969594X.2012.757546 .
Zimmerman, B. J., Bandura, A., & Martinez-Pons, M. (1992). Self-motivation for academic attainment: The role of self-efficacy beliefs and personal goal setting. American Educational Research Journal , 29 , 663–676.
Zimmerman, B. J., & Martinez-Pons, M. (1990). Student differences in self-regulated learning: Relating grade, sex, and giftedness to self-efficacy and strategy use. Journal of Educational Psychology , 82 , 51–59.
Zou, S., & Dong, M. (2014). Washback research of the recent two decades in China: Current situation and thought. China Foreign Language , 4 , 4–14.
Download references
Acknowledgements
I would like to thank my supervisor Professor Yoshinori Watanabe, my peer PhD students Ms. Makiko Kato and Ms. Makiko Habu. Also, I would like to thank my families. Finally, I would like to thank all the respondents who filled in the questionnaire.
Not applicable
Author information
Authors and affiliations.
Department of Linguistics, Sophia University, Yotsuya Campus, 7-1 Kioi-cho, Chiyoda-Ku, Tokyo, 102-8554, Japan
You can also search for this author in PubMed Google Scholar
Contributions
Jing Zhang performed the research and wrote this manuscript independently. The author(s) read and approved the final manuscript.
Corresponding author
Correspondence to Jing Zhang .
Ethics declarations
Competing interests.
The author declares that she has no competing interests.
Additional information
Publisher’s note.
Springer Nature remains neutral with regard to jurisdictional claims in published maps and institutional affiliations.
Rights and permissions
Open Access This article is licensed under a Creative Commons Attribution 4.0 International License, which permits use, sharing, adaptation, distribution and reproduction in any medium or format, as long as you give appropriate credit to the original author(s) and the source, provide a link to the Creative Commons licence, and indicate if changes were made. The images or other third party material in this article are included in the article's Creative Commons licence, unless indicated otherwise in a credit line to the material. If material is not included in the article's Creative Commons licence and your intended use is not permitted by statutory regulation or exceeds the permitted use, you will need to obtain permission directly from the copyright holder. To view a copy of this licence, visit http://creativecommons.org/licenses/by/4.0/ .
Reprints and permissions
About this article
Cite this article.
Zhang, J. A moderated mediation analysis of the relationship between a high-stakes English test and test takers’ extracurricular English learning activities. Lang Test Asia 11 , 5 (2021). https://doi.org/10.1186/s40468-021-00120-x
Download citation
Received : 02 November 2020
Accepted : 28 February 2021
Published : 08 April 2021
DOI : https://doi.org/10.1186/s40468-021-00120-x
Share this article
Anyone you share the following link with will be able to read this content:
Sorry, a shareable link is not currently available for this article.
Provided by the Springer Nature SharedIt content-sharing initiative
- Self-efficacy for self-regulated learning
- Self-efficacy for academic achievement
- Covariance-based Structural Equation Modeling
- Bootstrapping
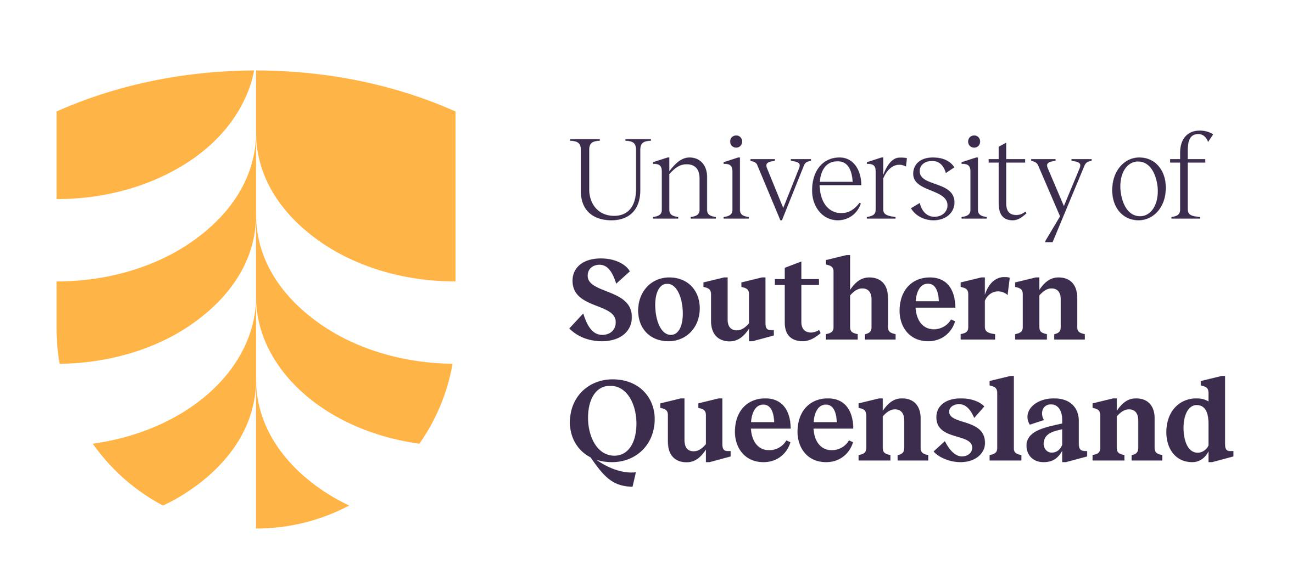
Want to create or adapt books like this? Learn more about how Pressbooks supports open publishing practices.
Section 7.2: Mediation Assumptions, The PROCESS Macro, Interpretation, and Write Up
Learning Objectives
At the end of this section you should be able to answer the following questions:
- Explain the assumptions that should be met before performing a mediation analysis.
- Explain the PROCESS Macro.
- What are the main ideas to focus on in mediation interpretation?
Mediation models focus on two effects – the direct effect and the indirect effect – and these can be combined into a measure of the model’s total effect.
Effects in a Simple Mediation Model
Using the prior example of the effects of conscientiousness and physical health, the indirect effect is the product of a and b = ab, from the previous figure. This is the indirect effect of the pathway from X to M, and M to Y. The total model effect is the combined direct effect and the indirect effect. The total effect quantifies how much two cases that differ by one unit on X are estimated to differ on Y.
Mediation Assumptions
There are a number of assumptions that should be met before performing a mediation analysis.
- The dependent, independent, and mediator variables (the variables of interest) need to be using a continuous scale.
- The variables of interest (the dependent variable and the independent and mediator variables) should have a linear relationship, which you can check with a scatterplot
- The data must not show multicollinearity (see Multiple Regression).
- There should be no spurious outliers, and the distribution of the variables should be approximately normal.
The MedMod Macro
The advent of affordable personal computers with statistical software has prompted researchers to develop new tools for analyses. Jamovi provides a number of free modules for more advanced analyses, including the MedMod Macro for meditation and moderation. Another tool for mediation analyses is the PROCESS Macro, which is available as a free extension for SPSS.
PowerPoint: Hayes PROCESS Macro
The following slide provides information on MedMod by illustrating where it appears in the Jamovi menu, and by showing menu option:
- Chapter Seven – MedMod Macro
Mediation Interpretation
PowerPoint: Mediation Menu and Results
The linked slides provide an example of mediation output:
- Chapter Seven – Mediation Menu and Results
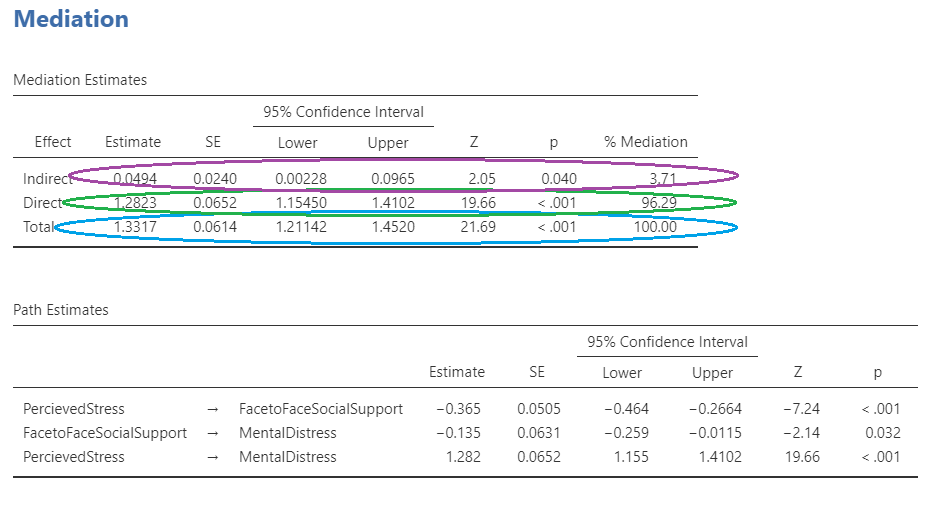
The total effect of the model can been seen in blue, with the direct effect (i.e. X and Y) in green. The indirect effect can be seen in purple, with the p value for the indirect effect can be found in orange. Now the interpretation of many of these statistics (p values etc) has been explained in previous lessons, but the main thing to focus on is the direct and indirect effects. If the direct effect is significant, then X does effect Y, and if there is a significant indirect effect then M does indeed mediate the relationship between X and Y.
Mediation Write Up
This mediation output results can be written up as follows:
A mediation analysiswas conducted to examine the mediating effect of social support on perceived stress and mental distress. The total effect of the model was found to be significant, b =1.33, z=21.69, BCa CI [1.21, 1.45], p <.001. It was found that there was a statistically significant direct effect, b =1.28, z=19.66, BCa CI [1.15, 1.41], p <.001. A statistically significant indirect effect was also found, b =0.05, z = 2.05, p= .040. These results suggest that social support partially mediated the relationship between perceived stress and mental distress
Statistics for Research Students Copyright © 2022 by University of Southern Queensland is licensed under a Creative Commons Attribution 4.0 International License , except where otherwise noted.
Share This Book

SPSS Mediation Analysis – The Complete Guide
How to examine mediation effects, spss regression dialogs, spss mediation analysis output, apa reporting mediation analysis, next steps - the sobel test, next steps - index of mediation.
A scientist wants to know which factors affect general well-being among people suffering illnesses. In order to find out, she collects some data on a sample of N = 421 cancer patients. These data -partly shown below- are in wellbeing.sav .
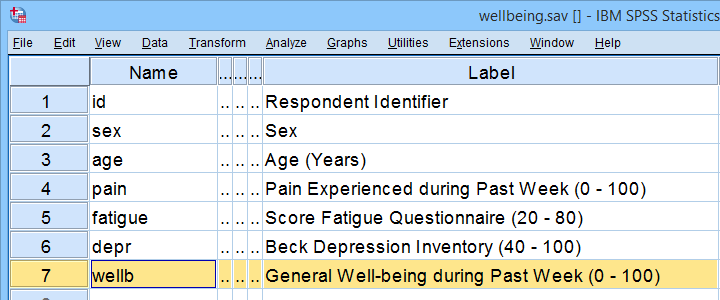
Now, our scientist believes that well-being is affected by pain as well as fatigue. On top of that, she believes that fatigue itself is also affected by pain. In short: pain partly affects well-being through fatigue. That is, fatigue mediates the effect from pain onto well-being as illustrated below.
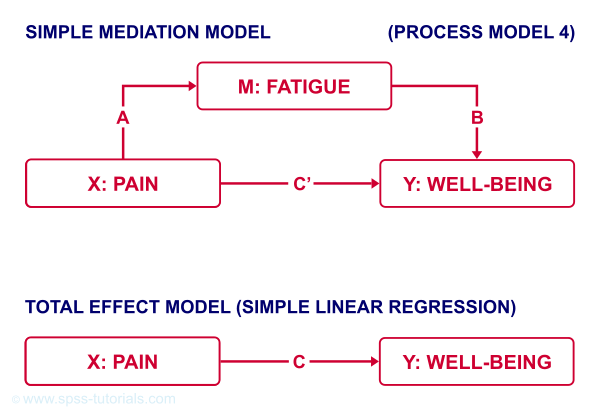
The lower half illustrates a model in which fatigue would (erroneously) be left out. This is known as the “total effect model” and is often compared with the mediation model above it.
Now, let's suppose for a second that all expectations from our scientist are exactly correct. If so, then what should we see in our data? The classical approach to mediation (see Kenny & Baron, 1986) says that
- \(a\) (from pain to fatigue) should be significant;
- \(b\) (from fatigue to well-being) should be significant;
- \(c\) (from pain to well-being) should be significant;
- \(c\,'\) ( direct effect) should be closer to zero than \(c\) ( total effect).
So how to find out if our data is in line with these statements? Well, all paths are technically just b-coefficients . We'll therefore run 3 (separate) regression analyses:
- regression from pain onto fatigue tells us if \(a\) is significant;
- multiple linear regression from pain and fatigue onto well-being tells us if \(b\) and \(c\,'\) are significant;
- regression from pain onto well-being tells if \(c\) is significant and/or different from \(c\,'\).
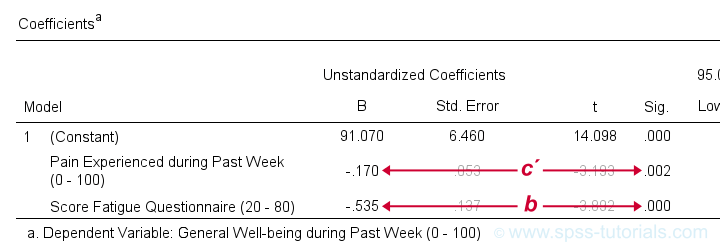
For a fairly basic analysis, we'll fill out these dialogs as shown below.
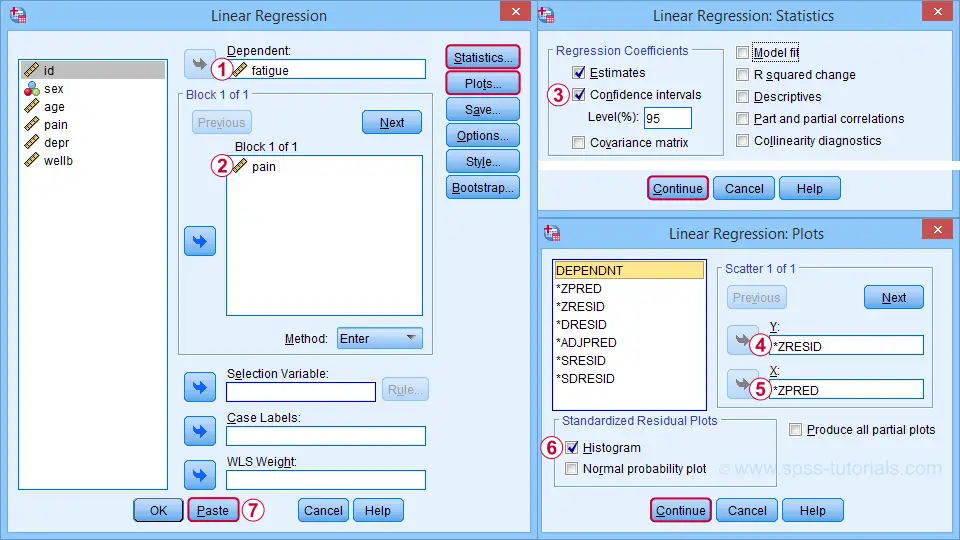
Completing these steps results in the SPSS syntax below. I suggest you shorten the pasted version a bit.
A second regression analysis estimates effects \(b\) and \(c\,'\). The easiest way to run it is to copy, paste and edit the first syntax as shown below.
We'll use the syntax below for the third (and final) regression which estimates \(c\), the total effect.
For our mediation analysis, we really only need the 3 coefficients tables. I copy-pasted them into this Googlesheet (read-only, partly shown below).
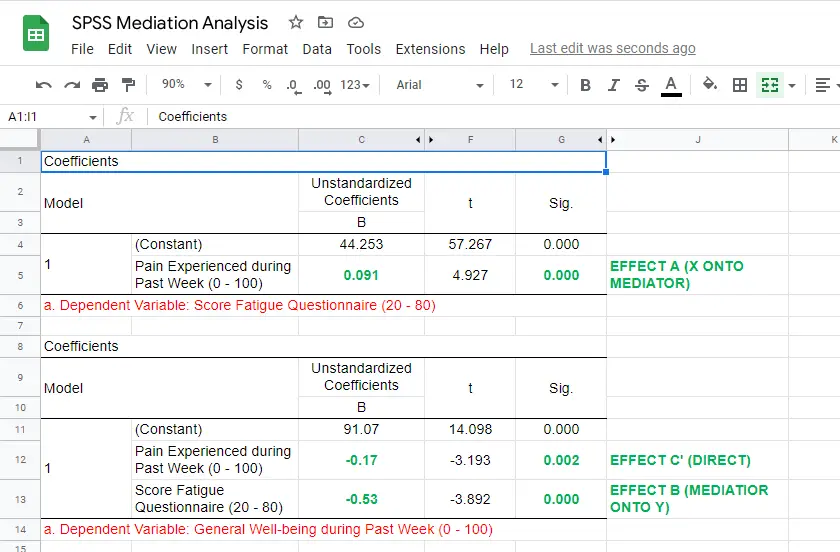
So what do we conclude? So what do we conclude? --> Well, all requirements for mediation are met by our results:
- effects \(a\), \(b\) and \(c\) are all statistically significant . This is because their “Sig.” or p < .05;
- the direct effect \(c\,'\) = -0.17 and thus closer to zero than the total effect \(c\) = -0.22.
- if \(c\) is significant but \(c\,'\)
The diagram below summarizes these results.
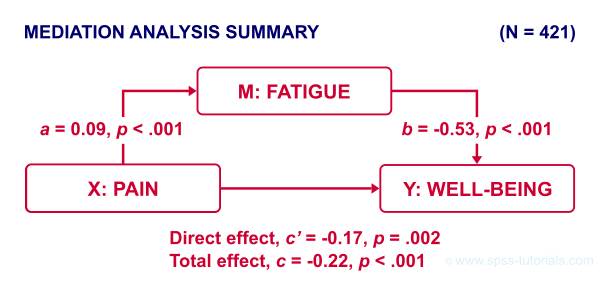
Note that both \(c\) and \(c\,'\) are significant. This is often called partial mediation : fatigue partially mediates the effect from pain onto well-being: adding it decreases the effect but doesn't nullify it altogether.
Besides partial mediation, we sometimes find full mediation . This means that \(c\) is significant but \(c\,'\) isn't: the effect is fully mediated and thus disappears when the mediator is added to the regression model.
Mediation analysis is often reported as separate regression analyses as in “the first step of our analysis showed that the effect of pain on fatigue was significant, b = 0.09, p < .001...” Some authors also include t-values and degrees of freedom (df) for b-coefficients. For some very dumb reason, SPSS does not report degrees of freedom but you can compute them as
$$df = N - k - 1$$
- \(N\) denotes the total sample size (N = 421 in our example) and
- \(k\) denotes the number of predictors in the model (1 or 2 in our example).
Like so, we could report “the second step of our analysis showed that the effect of fatigue on well-being was also significant, b = -0.53, t (419) = -3.89, p < .001...”
In our analysis, the indirect effect of pain via fatigue onto well-being consists of two separate effects, \(a\) (pain onto fatigue) and \(b\) fatigue onto well-being. Now, the entire indirect effect \(ab\) is simply computed as
$$\text{indirect effect} \;ab = a \cdot b$$
This makes perfect sense: if wage \(a\) is $30 per hour and tax \(b\) is $0.20 per dollar income, then I'll pay $30 · $0.20 = $6.00 tax per hour, right?
For our example, \(ab\) = 0.09 · -0.53 = -0.049: for every unit increase in pain, well-being decreases by an average 0.049 units via fatigue. But how do we obtain the p-value and confidence interval for this indirect effect? There's 2 basic options:
- the modern literature favors bootstrapping as implemented in the PROCESS macro which we'll discuss later;
- the Sobel test (also known as “normal theory” approach).
The second approach assumes \(ab\) is normally distributed with
$$se_{ab} = \sqrt{a^2se^2_b + b^2se^2_a + se^2_a se^2_b}$$
\(se_{ab}\) denotes the standard error of \(ab\) and so on.
For the actual calculations, I suggest you try our Sobel Test Calculator.xlsx , partly shown below.
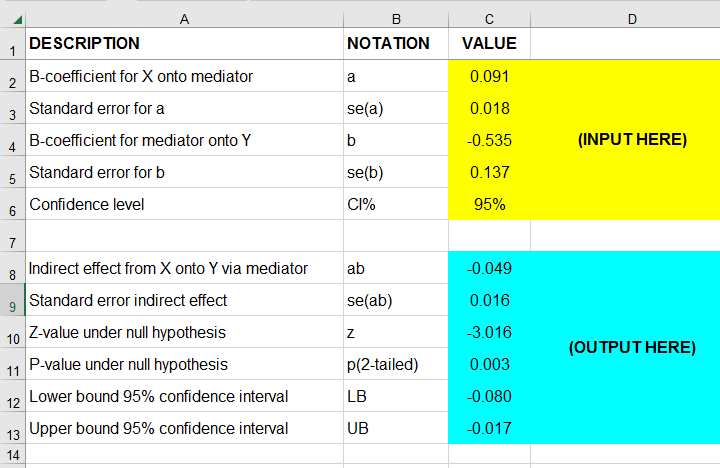
So what does this tell us? Well, our indirect effect is significant, B = -0.049, p = .002, 95% CI [-0.08, -0.02].
Our research variables (such as pain & fatigue) were measured on different scales without clear units of measurement. This renders it impossible to compare their effects. The solution is to report standardized coefficients known as β (Greek letter “beta”).
Our SPSS output already includes beta for most effects but not for \(ab\). However, we can easily compute it as
$$\beta_{ab} = \frac{ab \cdot SD_x}{SD_y}$$
\(SD_x\) is the sample-standard-deviation of our X variable and so on.
This standardized indirect effect is known as the index of mediation . For computing it, we may run something like DESCRIPTIVES pain wellb. in SPSS. After copy-pasting the resulting table into this Googlesheet , we'll compute \(\beta_{ab}\) with a quick formula as shown below.
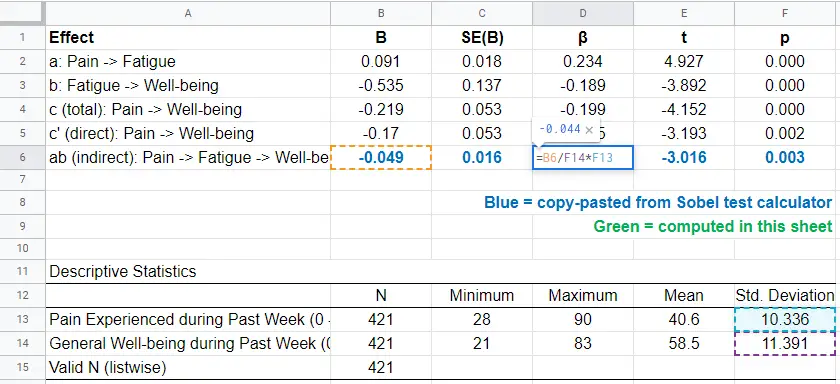
Adding the output from our Sobel test calculator to this sheet results in a very complete and clear summary table for our mediation analysis.
Final Notes
Mediation analysis in SPSS can be done with or without the PROCESS macro. Some reasons for not using PROCESS are that
- many people find PROCESS difficult to use and dislike its output format;
- PROCESS can't create regression residuals and the associated plots for checking regression assumptions such as linearity, homoscedasticity and normality;
- the PROCESS output does not include adjusted r-squared;
- PROCESS does not offer pairwise exclusion of missing values .
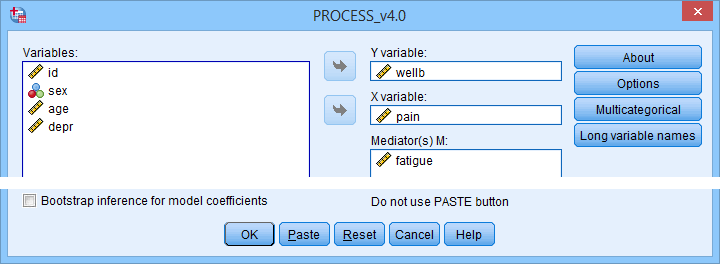
So why does anybody use PROCESS? Some reasons may be that
- PROCESS uses bootstrapping rather than the Sobel test. This is said to result in higher power and more accurate confidence intervals. Sadly, bootstrapping does not yield a p-value for the indirect effect whereas the Sobel test does;
- using PROCESS may save a lot of work for more complex models (parallel, serial and moderated mediation);
- if needed, PROCESS handles dummy coding for the X variable and moderators (if any);
- PROCESS doesn't require the additional calculations that we implemented in our Googlesheet: it calculates everything you need in one go.
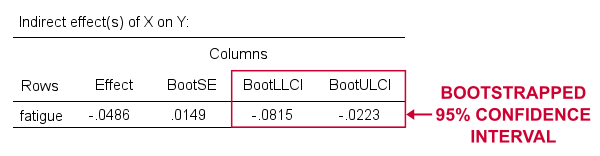
Right. I hope this tutorial has been helpful for running, reporting and understanding mediation analysis in SPSS. This is perhaps not the easiest topic but remember that practice makes perfect.
Thanks for reading!
Tell us what you think!
Privacy overview.
- FanNation FanNation FanNation
- SI.COM SI.COM SI.COM
- SI Swimsuit SI Swimsuit SI Swimsuit
- SI Sportsbook SI Sportsbook SI Sportsbook
- SI Tickets SI Tickets SI Tickets
- SI Showcase SI Showcase SI Showcase
- SI Resorts SI Resorts SI Resorts
Report: Glen Taylor, A-Rod and Lore set for mediation over Timberwolves ownership dispute
The two sides will reportedly meet for mediation on May 1.
- Author: Joe Nelson
In this story:
Who will be the majority owner of the Minnesota Timberwolves and Lynx when the public dispute between Glen Taylor and hopeful owners Marc Lore and Alex Rodriguez is settled?
Taylor has repeated that he plans to continue running the show while Lore and Rodriguez have gone on record saying they did nothing to breach the contract that would've seen them become majority owners of Minnesota's NBA and WNBA franchises. Stuck in a stalemate, a mediation session has been scheduled for May 1, according to ESPN's Adrian Wojnarowski.
ESPN Sources: A mediation session has been set for May 1 in Minneapolis in the Timberwolves’ ownership dispute between Glen Taylor and the Marc Lore/Alex Rodriguez group. Taylor ended ownership transition when he said Lore/ARod failed to meet deadlines on sales conditions. pic.twitter.com/qbsBCo5LZ1 — Adrian Wojnarowski (@wojespn) April 23, 2024
Taylor, 83, announced March 28 that the teams were no longer for sale, claiming Lore and Rodriguez didn't meet contractual obligations laid out in the purchase agreement the two sides signed in 2021.
"What I anticipate doing — if I can work everything out the way I'm planning on it — is I'll just continue to keep the ownership," Taylor told Twin Cities TV station Fox 9 last week. "I'll have the controlling ownership and just keep on running. We have a plan that upon my death we have a plan of what will happen, but they'll stay in Minnesota and they'll be controlled by my family."
Lore and Rodriguez have maintained that they didn't miss a deadline and believe Taylor has a case of "seller's remorse."
“We’re going to be the owners of the Minnesota Timberwolves,” Lore told Sportico after Taylor's surprising decision to take the teams off the market. “It’s just a matter of time, and how much pain Glen wants to put the fans, the players, the town and community through. It’s his choice. It didn’t have to be this way.”
Rodriguez, an interview on the Dane Moore NBA Podcast, said he and Lore were waiting on NBA approval for funding when Taylor dropped a "nuclear bomb" on their plans.
"The only reason we’re here is because we’ve been attacked, and this is a childhood dream for Marc and I," Rodriguez said March 29. "And our lawyers tell us that we have an ironclad agreement, and we’ll never relent.”
It's unclear who is hosting the mediation and if it will result in the needle moving in favor of Taylor or Lore and Rodriguez.
Latest Timberwolves News
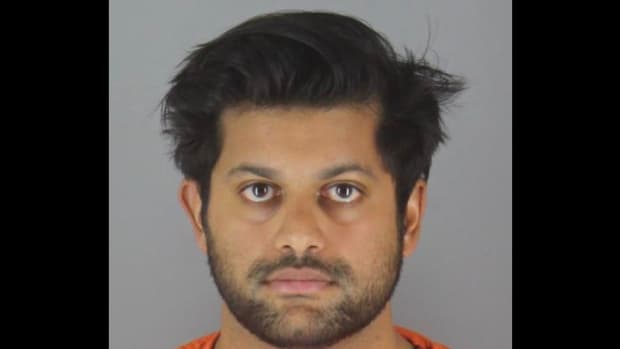
Fired Wolves employee charged with stealing 'strategic NBA information' from team exec
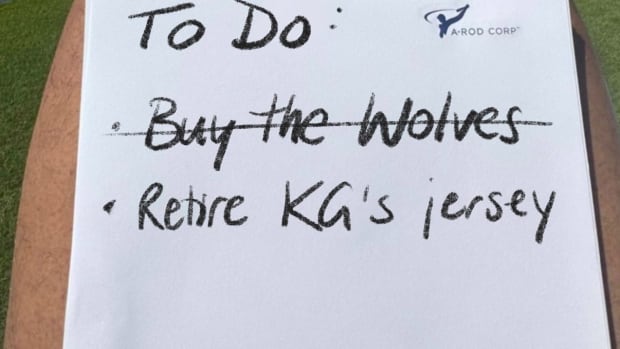
A-Rod hints that retiring KG's jersey will follow takeover
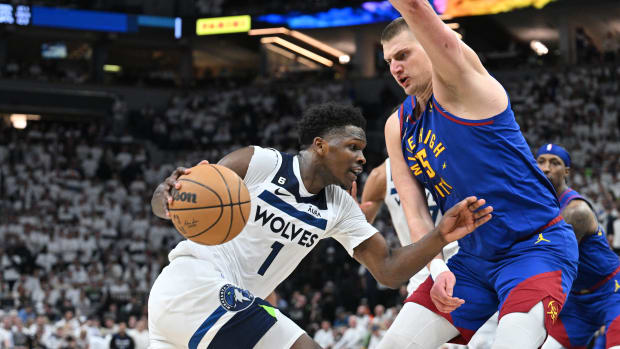
Nikola Jokic's Strong Statement on Anthony Edwards
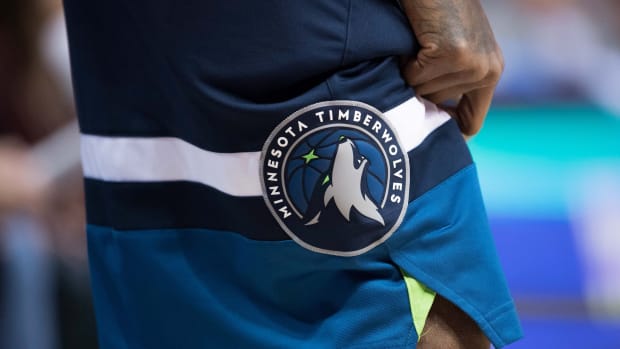
Police Charge Ex-T-Wolves Employee With Theft of Exec’s Hard Drive
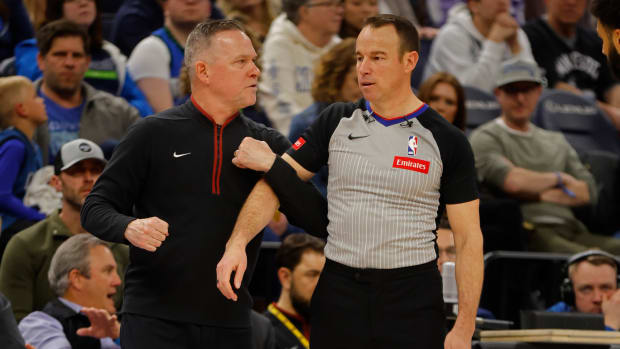
NBA Admits Missed Calls in Nuggets vs. Timberwolves
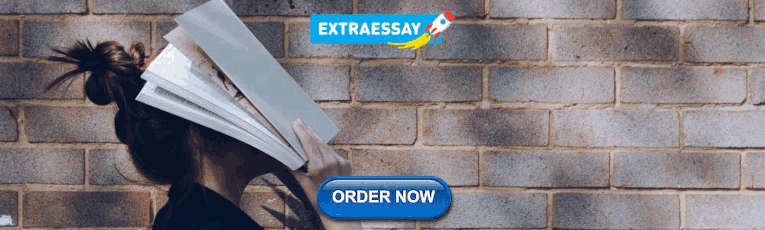
COMMENTS
Mediation analysis was developed to assess this "black box," and psychologists and social scientists have utilized this framework particularly frequently. Mediation analysis can explore and evaluate biological or social mechanisms, thereby elucidating unknown biological pathways and/or aiding in policy-making . However, because of advances ...
The model performance criteria utilized in the study were R 2 , Q 2 and the effect size (f 2 ), and the path analysis was estimated with the path value, t score and significance level [30].
Background Mediation analysis investigates whether a variable (i.e., mediator) changes in regard to an independent variable, in turn, affecting a dependent variable. Moderation analysis, on the other hand, investigates whether the statistical interaction between independent variables predict a dependent variable. Although this difference between these two types of analysis is explicit in ...
%PDF-1.7 %äãÏÒ 152 0 obj /Linearized 1.0 /O 154 /H [ 682 766 ] /L 329019 /E 26564 /N 26 /T 325861 >> endobj xref 152 15 0000000015 00000 n 0000000632 00000 n 0000001448 00000 n 0000001595 00000 n 0000001645 00000 n 0000006243 00000 n 0000007069 00000 n 0000007786 00000 n 0000016874 00000 n 0000017680 00000 n 0000018205 00000 n 0000026385 00000 n 0000026476 00000 n 0000000682 00000 n ...
Additional assumptions in mediation analysis refer to the correct timing and level of the mediated effect (MacKinnon 2008).Specifically, conclusions based on a single (as compared to repeated) assessment of X, M, or Y assume that the variables and relationships of interest do not change over time. In addition, inferring mediation without taking into account possible nesting of the data (e.g ...
On the Interpretation and Use of Mediation: Multiple Perspectives on Mediation Analysis. Robert Agler 1,2 * Paul De Boeck 1,3. 1 Department of Psychology, Ohio State University, Columbus, OH, United States. 2 Division of Epidemiology, College of Public Health, Ohio State University, Columbus, OH, United States.
Mediation analysis (MA) is a very common type of statistical analysis in psychology, sociology, epidemiology, and medicine. This type of analysis aims to discover pathways and mechanisms by which an exposure may affect an outcome. This review describes how MA was conducted and reported in recent randomized controlled trials.
(MacKinnon, 2008). Mediation analysis of an intervention trial's outcomes provides a way of evaluating the hypotheses that link an intervention to a mediator and a mediator to an outcome. In this thesis, we demonstrate a novel approach to mediation analysis that enables the evaluation of hypotheses about individual intervention components.
extending mediation analysis into a setting with time-varying and multiple mediators. An interventional approach has been used to define and identify the direct and indirect effects as well as path specific effects based in a causal inference framework, propose a parametric approach to estimate these effects, and provide an algorithm as well as ...
Mediation Analysis of the Efficacy of a Training and Technical Assistance Implementation Strategy on Intention to Implement a Couple-based HIV/STI Prevention Intervention Timothy Hunt The purpose of this study was to examine the effectiveness and exposure of an implementation
Key Points. Question What information should be reported in studies that include mediation analyses of randomized trials and observational studies?. Findings An international Delphi and consensus process (using the Enhancing Quality and Transparency of Health Research methodological framework) generated a 25-item reporting guideline for primary reports of mediation analyses and a 9-item short ...
Mediation analysis is increasingly being applied in many research fields [], including the field of epidemiology.Mediation analysis decomposes the total exposure-outcome effect into a direct effect and an indirect effect through a mediator variable [2,3,4].For example, mediation analysis can be used to investigate BMI as a mediator of the relation between smoking and insulin levels [], or to ...
Firstly, confirmatory factor analysis (CFA) was performed to assess the measurement model. Secondly, mediation analysis was conducted employing bootstrapping (Hayes, 2009) to answer research questions 1 and 2. Finally, the subgroup method and bootstrapping were applied to conduct a moderated mediation analysis to answer research question 3.
mediation analysis and provide up-to-date guidelines for researchers to make informed decisions and conduct the analysis appropriately. Keywords: Mediation analysis, Baron and Kenny, Preacher and Hayes, Malaysia, MySEM INTRODUCTION Mediational designs are at the heart of social science and business research, often referred to as
positive, yet complex, relationship between spirituality and physical health. Of even greater interest to psychologists is the link between spirituality and mental health, and in fact, a large. body of research exists examining this relationship. Spirituality and mental health and well-being.
Published on March 1, 2021 by Pritha Bhandari . Revised on June 22, 2023. A mediating variable (or mediator) explains the process through which two variables are related, while a moderating variable (or moderator) affects the strength and direction of that relationship. Including mediators and moderators in your research helps you go beyond ...
compliance. The mediation analysis showed that there was no mediating effect of amount of processing on the relationship between type of influence technique and compliance or on the other investigated relationships in this study. Furthermore, positive mood moderated the relationship between type of influence technique and compliance.
DigitalCommons@URI | University of Rhode Island Research
Mediation Write Up. This mediation output results can be written up as follows: A mediation analysiswas conducted to examine the mediating effect of social support on perceived stress and mental distress. The total effect of the model was found to be significant, b=1.33, z=21.69, BCa CI [1.21, 1.45], p<.001.
For a fairly basic analysis, we'll fill out these dialogs as shown below. Completing these steps results in the SPSS syntax below. I suggest you shorten the pasted version a bit. *EFFECT A (X ONTO MEDIATOR). REGRESSION. /MISSING LISTWISE. /STATISTICS COEFF OUTS CI (95) R ANOVA. /CRITERIA=PIN (.05) POUT (.10) /NOORIGIN.
Thesis. Stanley Black & Decker, Inc. ( NYSE: SWK) performance has suffered since 2022. In my opinion, management is the key to superior returns. Management needs to execute and provide investors ...
As growth has increased, so has the stock's valuation. The stock has an average price-to-earnings ratio of 41.0 since 2019 and 34.1 since 2014. According to Seeking Alpha, analysts expect that ...
Taylor, 83, announced March 28 that the teams were no longer for sale, claiming Lore and Rodriguez didn't meet contractual obligations laid out in the purchase agreement the two sides signed in 2021.