
An official website of the United States government
The .gov means it’s official. Federal government websites often end in .gov or .mil. Before sharing sensitive information, make sure you’re on a federal government site.
The site is secure. The https:// ensures that you are connecting to the official website and that any information you provide is encrypted and transmitted securely.
- Publications
- Account settings
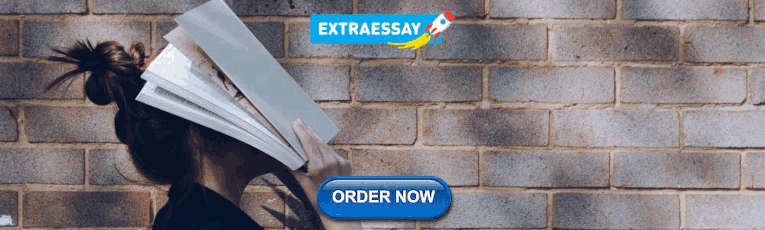
Trending Articles
- Depleting myeloid-biased haematopoietic stem cells rejuvenates aged immunity. Ross JB, et al. Nature. 2024. PMID: 38538791
- Global fertility in 204 countries and territories, 1950-2021, with forecasts to 2100: a comprehensive demographic analysis for the Global Burden of Disease Study 2021. GBD 2021 Fertility and Forecasting Collaborators. Lancet. 2024. PMID: 38521087
- Formation of memory assemblies through the DNA-sensing TLR9 pathway. Jovasevic V, et al. Nature. 2024. PMID: 38538785
- Single-cell dissection of the human motor and prefrontal cortices in ALS and FTLD. Pineda SS, et al. Cell. 2024. PMID: 38521060
- Tumor-secreted FGF21 acts as an immune suppressor by rewiring cholesterol metabolism of CD8 + T cells. Hu C, et al. Cell Metab. 2024. PMID: 38537635 No abstract available.
Latest Literature
- Ann Neurol (1)
- Arch Phys Med Rehabil (1)
- Gastroenterology (4)
- J Biol Chem (7)
- J Clin Endocrinol Metab (1)
- J Neurosci (2)
- Nat Commun (32)
NCBI Literature Resources
MeSH PMC Bookshelf Disclaimer
The PubMed wordmark and PubMed logo are registered trademarks of the U.S. Department of Health and Human Services (HHS). Unauthorized use of these marks is strictly prohibited.
- Search by keyword
- Search by citation
Page 1 of 492
Socioeconomic inequalities in type 2 diabetes mellitus: a study based on a population-based survey in Iran
Type 2 diabetes mellitus (T2DM) is the most prevalent form of Diabetes Mellitus (DM), with social and economic determinants significantly influencing its prevalence. This study aimed to analyze the socioeconom...
- View Full Text
Proximity to public green spaces and depressive symptoms among South African residents: a population-based study
Exposure to green spaces has been suggested to improve mental health and may reduce the risk of depression. However, there is generally limited evidence on the association between green spaces and depression o...
Influenza vaccine coverage and factors associated with non-vaccination among caregiving and care-receiving adults in the Canadian Longitudinal Study on Aging (CLSA)
Influenza vaccination is recommended for those at increased risk of influenza complications and their household contacts to help reduce influenza exposure. Adults who require care often experience health issue...
Adulterants present in the San Diego county fentanyl supply: a laboratory analysis of seized law enforcement samples
The opioid overdose crisis is one of the worst public health crises ever to face the US and emerging evidence suggests its effects are compounded by the presence of drug adulterants. Here we report our efforts...
Knowledge, attitude, and practice toward weight management among diabetic patients in Qidong City, Jiangsu Province
Weight management is an effective prevention and treatment strategy for diabetes mellitus. This study aimed to assess the knowledge, attitude, and practice (KAP) of diabetic patients towards weight management.
Who chooses “healthy” meals? An analysis of lunchtime meal quality in a workplace cafeteria
The workplace can play an important role in shaping the eating behaviors of U.S. adults. Unfortunately, foods obtained in the workplace tend to be low in nutritional quality. Questions remain about the best wa...
Study on the measurement of coupling and coordinated development level between China’s internet and elderly care services and its influencing factors
With the intensification of China’s aging population, the demand for elderly care services has become increasingly prominent. At the same time, rapid development of internet technology provides more convenienc...
Chronic diseases and determinants of community health services utilization among adult residents in southern China: a community-based cross-sectional study
The burden of chronic diseases has become a major public health concern, and high-efficiency use of community health services is essential in combating chronic diseases. This study described the status of chro...
Malaria infection and predictor factors among Chadian nomads’ children
In Chad, malaria remains a significant public health concern, particularly among nomadic populations. Geographical factors and the mobility of human populations have shown to be associated with the diversity of P...
Inspecting the “health poverty trap” mechanism: self-reinforcing effect and endogenous force
The term “health poverty trap” describes a vicious cycle in which developing countries or regions become trapped in low levels of health and poverty during the process of modernization. Although significant pr...
Dietary diversity and its determinants among women of reproductive age residing in the urban area of Nouakchott, Mauritania
The intake of nutrient-rich foods from diverse diets ensures adequate nutrition for women. This study aims to determine dietary diversity among women of reproductive age (WRA) using the MDD-W indicator and how...
How do people with long COVID utilize COVID-19 vaccination and rehabilitation services and what are their experiences with these services? results of a qualitative study with 48 participants from Germany
Studies estimate that at least 7.5% of adults are affected by long-term symptoms such as fatigue or cognitive impairment after the acute phase of COVID-19. COVID-19 vaccination may reduce the risk of long COVI...
One-year trajectories of nutritional status in perimenopausal women: a community-based multi-centered prospective study
Nutritional status is a modifiable factor associated with perimenopausal women’s health and quality of life. Assessing body composition indicators helps to comprehensively understand nutritional status compare...
Connecting families: a qualitative study examining the experiences of parenting young children under financial strain in Ontario, Canada
There is little research investigating the subjective experiences of parenting young children while living in poverty and experiencing financial strain using qualitative methodologies. Therefore, the objective...
Absorbent hygiene products disposal behaviour in informal settlements: identifying determinants and underlying mechanisms in Durban, South Africa
Within South Africa, many low-income communities lack reliable waste management services. Within these contexts, absorbent hygiene product (AHP) waste, including nappies (diapers), are not recycled, and are of...
Racial/ethnic differences in the association between transgender-related U.S. state policies and self-rated health of transgender women
Policy protections for transgender adults in the United States are consistently associated with positive health outcomes. However, studies over-represent non-Latinx White transgender people and obscure variati...
Coverage and determinants of HIV testing and counseling services among mothers attending antenatal care in sub-Saharan African countries: a multilevel analysis
HIV/AIDS is one of the top global public health threats that causes significant cases, deaths, and socioeconomic impact. Even though both HIV testing and counseling are identified as essential HIV intervention...
Menstruating while homeless: navigating access to products, spaces, and services
People experiencing homelessness (PEH) in the United States face substantial challenges related to menstruation, exacerbated by the COVID-19 pandemic. Limited access to period products, heightened stigma, and ...
Digital health literacy and associated factors among internet users from China: a cross-sectional study
As the internet develops and 5G technology becomes increasingly prominent, the internet has become a major source of health-related information. Increasingly, people use the internet to find health-related inf...
Job boredom as an antecedent of four states of mental health: life satisfaction, positive functioning, anxiety, and depression symptoms among young employees – a latent change score approach
Job boredom has been generally associated with poorer self-rated health but the evidence is mainly cross-sectional and there is a lack of a holistic mental health approach. We examined the temporal relationshi...
Psychometric analysis and linguistic adaptation of the Persian version of Contraceptive Self-Efficacy Scale (CSES-P)
This study was aimed to test adaptability of the Contraceptive Self-Efficacy Scale (CSES) for use on Persian-speaking women of reproductive age.
The individuals’ awareness and adoption of electronic health records in China: a questionnaire survey of 1,337 individuals
Electronic health records (EHRs) are digital records of individual health information. However, their adoption and utilization remain low. This study explores the factors influencing the implementation of EHRs...
Linkages between the Sustainable Development Goals and health in Somalia
The Sustainable Development Goals (SDGs) adopted in 2015 compromises 17 universal and indivisible goals for sustainable development, however the interactions between the SDGs in Somalia is not known which is v...
Food insecurity in urban American Indian and Alaska Native populations during the COVID-19 pandemic
Food insecurity is an important social determinant of health that was exacerbated by the COVID-19 pandemic. Both food insecurity and COVID-19 infection disproportionately affect racial and ethnic minority grou...
Cyberchondria severity and utilization of health services in Polish society: a cross-sectional study
It has been suggested that cyberchondria leads to increased utilization of healthcare services. Unfortunately, not many studies have analyzed this effect comprehensively. The aim of this study was to analyze t...
A novel correction method for modelling parameter-driven autocorrelated time series with count outcome
Count time series (e.g., daily deaths) are a very common type of data in environmental health research. The series is generally autocorrelated, while the widely used generalized linear model is based on the as...
Publisher Correction: Association of urinary chlorpyrifos, paraquat, and cyproconazole levels with the severity of fatty liver based on MRI
The original article was published in BMC Public Health 2024 24 :807
Knowledge, attitudes, and practices towards Kawasaki disease from caregivers of children with Kawasaki disease: a cross-sectional study
To examine the knowledge, attitudes, and practices (KAP) of caregivers of children with Kawasaki disease toward Kawasaki disease.
Cross-country variations in the caregiver role: evidence from the ENTWINE-iCohort study
Globally, economically developed countries face similar ageing demographics and the challenge of a ‘care gap’, yet they vary due to different care and formal support systems, and different cultural and societa...
ICT penetration and life expectancy in emerging market economies: panel evidence from asymmetric causality analysis
Life expectancy is a significant result indicator of public health and sustainable development. Therefore, one of the final objectives of all economic and social policies is to increase the life expectancy. In...
Differences in nutritional status and level of physical activity among adolescents living in urban and rural areas of Montenegro - national study
Nutritional status and physical activity are important factors for adolescent health. These factors may vary by the place of residence. This study aims to assess the nutritional status and physical activity le...
Changes in social mixing and attitudes and practices to precautionary measures in a maturing COVID-19 pandemic in six communities in Sudan: a qualitative study
With low COVID-19 vaccination coverage, non-pharmaceutical interventions were critical to mitigating the COVID-19 pandemic in Sudan. We explored changes in social contact patterns, risk perception, attitudes, ...
The relationship between psychological distress and weight maintenance in weight cycling: mediating role of eating behavior
Obesity is a global public health concern. The goal of this study was to see if eating habits could mediate the relationship between psychological distress and weight maintenance in a population with a history...
Barriers and enabling factors for utilizing physical rehabilitation services by Afghan immigrants and refugees with disabilities in Iran: a qualitative study
Individuals with a migrant background often underutilize physical rehabilitation services (PRS) compared to the host population. This disparity is attributed to various barriers, including limited access to in...
Design and usability evaluation of a mobile application for self-care among Iranian adolescents
Mobile phones can be an ideal platform to engage adolescents to maintain, improve, and promote self-care. Therefore, the current study aims to design and evaluate the usability of a mobile application for self...
Changes in disease burden and global inequalities in bladder, kidney and prostate cancers from 1990 to 2019: a comparative analysis based on the global burden of disease study 2019
Bladder, kidney and prostate cancers make significant contributors to cancer burdens. Exploring their cross-country inequalities may inform equitable strategies to meet the 17 sustainable development goals bef...
A great way to bring up health behaviour topics at playgroup: a qualitative evaluation of the Healthy Conversations @ Playgroup program
The early years is a critical stage to establish optimal nutrition and movement behaviours. Community playgroups are a relaxed environment for parents with a focus on social connection and supporting parents i...
Neighborhood-level factors associated with COVID-19 vaccination rates: a case study in Chicago
Chicago’s deeply-rooted racial and socioeconomic residential segregation is a pattern mirrored in other major cities, making it a prototype for studying the uptake of public health interventions across the US....
Food safety and dietary diversity in African urban cities: evidence from Ghana
Food safety is integral to food security and is increasingly becoming a significant concern in the urban areas of Africa, which are rapidly growing in population. In the case of Ghana, many urban households de...
Exploring the medical decision-making patterns and influencing factors among the general Chinese public: a binary logistic regression analysis
With the ongoing evolution of the healthcare system and shifts in cultural paradigms, there is a pressing need to delve into the medical decision-making behaviors of general Chinese public and understand their...
Pregnancy health in a multi-state U.S. population of systemically underserved patients and their children: PROMISE cohort design and baseline characteristics
Gestational weight gain (GWG) is a routinely monitored aspect of pregnancy health, yet critical gaps remain about optimal GWG in pregnant people from socially marginalized groups, or with pre-pregnancy body ma...
Key predictors of food security and nutrition in Africa: a spatio-temporal model-based study
There is voluminous literature on Food Security in Africa. This study explicitly considers the spatio-temporal factors in addition to the usual FAO-based metrics in modeling and understanding the dynamics of f...
Systematic review of empiric studies on lockdowns, workplace closures, and other non-pharmaceutical interventions in non-healthcare workplaces during the initial year of the COVID-19 pandemic: benefits and selected unintended consequences
We conducted a systematic review aimed to evaluate the effects of non-pharmaceutical interventions within non-healthcare workplaces and community-level workplace closures and lockdowns on COVID-19 morbidity an...
Racial discrimination is associated with food insecurity, stress, and worse physical health among college students
Students of color disproportionately experience racial discrimination and food insecurity, which both lead to poor academic and health outcomes. This study explores the extent to which the location of racial d...
Efficacy of health literacy interventions aimed to improve health gains of higher education students—a systematic review
Health literacy (HL) among higher education students is low, making them vulnerable about their health. To reverse this trend, higher education institutions promote HL interventions with various topics and met...
Covid-19 skepticism and public health norms during refugee assistance: does skepticism always lead to poor safety protocol adherence?
Skepticism about COVID-19’s existence or severity has spread as fast as the disease itself, and in some populations has been shown to undermine protective public health behaviors that can mitigate infection. F...
Correction to: Impact of COVID-19 on mental health of health care workers in Spain: a mix-methods study
The original article was published in BMC Public Health 2024 24 :463
Trends in psychosomatic symptoms among adolescents and the role of lifestyle factors
Adolescent mental health problems are on the rise globally, including in Sweden. One indicator of this trend is increased psychosomatic symptoms (PSS) over time. Lifestyle factors such as physical activity (PA...
Content and face validity of Workplace COVID-19 Knowledge & Stigma Scale (WoCKSS)
The COVID-19 pandemic has led to fear, rumours, and stigma, particularly against those infected with the virus. In Malaysia, the manufacturing industry is particularly vulnerable to COVID-19 clusters, making i...
Risk of diabetes and hypertension in a population with alcohol use disorders
A population-based follow-up study assessing the risk of developing hypertension and diabetes associated with alcohol use disorder (AUD) is crucial. We investigated this relationship by using insurance claims ...
Important information
Editorial board
For authors
For editorial board members
For reviewers
- Manuscript editing services
Annual Journal Metrics
2022 Citation Impact 4.5 - 2-year Impact Factor 4.7 - 5-year Impact Factor 1.661 - SNIP (Source Normalized Impact per Paper) 1.307 - SJR (SCImago Journal Rank)
2023 Speed 32 days submission to first editorial decision for all manuscripts (Median) 173 days submission to accept (Median)
2023 Usage 24,332,405 downloads 24,308 Altmetric mentions
- More about our metrics
Peer-review Terminology
The following summary describes the peer review process for this journal:
Identity transparency: Single anonymized
Reviewer interacts with: Editor
Review information published: Review reports. Reviewer Identities reviewer opt in. Author/reviewer communication
More information is available here
- Follow us on Twitter
BMC Public Health
ISSN: 1471-2458
- Submission enquiries: [email protected]
- General enquiries: [email protected]

An official website of the United States government
The .gov means it’s official. Federal government websites often end in .gov or .mil. Before sharing sensitive information, make sure you’re on a federal government site.
The site is secure. The https:// ensures that you are connecting to the official website and that any information you provide is encrypted and transmitted securely.
- Publications
- Account settings
Preview improvements coming to the PMC website in October 2024. Learn More or Try it out now .
- Advanced Search
- Journal List
- Future Healthc J
- v.8(2); 2021 Jul

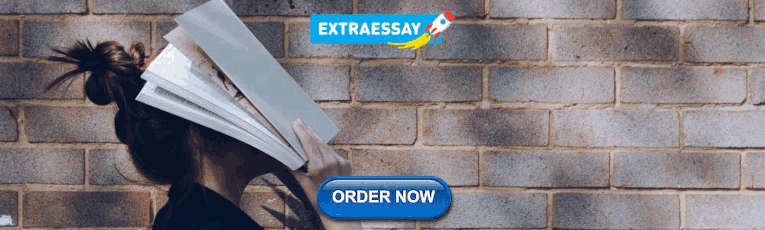
Artificial intelligence in healthcare: transforming the practice of medicine
Junaid bajwa.
A Microsoft Research, Cambridge, UK
Usman Munir
B Microsoft Research, Cambridge, UK
Aditya Nori
C Microsoft Research, Cambridge, UK
Bryan Williams
D University College London, London, UK and director, NIHR UCLH Biomedical Research Centre, London, UK
Artificial intelligence (AI) is a powerful and disruptive area of computer science, with the potential to fundamentally transform the practice of medicine and the delivery of healthcare. In this review article, we outline recent breakthroughs in the application of AI in healthcare, describe a roadmap to building effective, reliable and safe AI systems, and discuss the possible future direction of AI augmented healthcare systems.
Introduction
Healthcare systems around the world face significant challenges in achieving the ‘quadruple aim’ for healthcare: improve population health, improve the patient's experience of care, enhance caregiver experience and reduce the rising cost of care. 1–3 Ageing populations, growing burden of chronic diseases and rising costs of healthcare globally are challenging governments, payers, regulators and providers to innovate and transform models of healthcare delivery. Moreover, against a backdrop now catalysed by the global pandemic, healthcare systems find themselves challenged to ‘perform’ (deliver effective, high-quality care) and ‘transform’ care at scale by leveraging real-world data driven insights directly into patient care. The pandemic has also highlighted the shortages in healthcare workforce and inequities in the access to care, previously articulated by The King's Fund and the World Health Organization (Box (Box1 1 ). 4,5
Workforce challenges in the next decade
The application of technology and artificial intelligence (AI) in healthcare has the potential to address some of these supply-and-demand challenges. The increasing availability of multi-modal data (genomics, economic, demographic, clinical and phenotypic) coupled with technology innovations in mobile, internet of things (IoT), computing power and data security herald a moment of convergence between healthcare and technology to fundamentally transform models of healthcare delivery through AI-augmented healthcare systems.
In particular, cloud computing is enabling the transition of effective and safe AI systems into mainstream healthcare delivery. Cloud computing is providing the computing capacity for the analysis of considerably large amounts of data, at higher speeds and lower costs compared with historic ‘on premises’ infrastructure of healthcare organisations. Indeed, we observe that many technology providers are increasingly seeking to partner with healthcare organisations to drive AI-driven medical innovation enabled by cloud computing and technology-related transformation (Box (Box2 2 ). 6–8
Quotes from technology leaders
Here, we summarise recent breakthroughs in the application of AI in healthcare, describe a roadmap to building effective AI systems and discuss the possible future direction of AI augmented healthcare systems.
What is artificial intelligence?
Simply put, AI refers to the science and engineering of making intelligent machines, through algorithms or a set of rules, which the machine follows to mimic human cognitive functions, such as learning and problem solving. 9 AI systems have the potential to anticipate problems or deal with issues as they come up and, as such, operate in an intentional, intelligent and adaptive manner. 10 AI's strength is in its ability to learn and recognise patterns and relationships from large multidimensional and multimodal datasets; for example, AI systems could translate a patient's entire medical record into a single number that represents a likely diagnosis. 11,12 Moreover, AI systems are dynamic and autonomous, learning and adapting as more data become available. 13
AI is not one ubiquitous, universal technology, rather, it represents several subfields (such as machine learning and deep learning) that, individually or in combination, add intelligence to applications. Machine learning (ML) refers to the study of algorithms that allow computer programs to automatically improve through experience. 14 ML itself may be categorised as ‘supervised’, ‘unsupervised’ and ‘reinforcement learning’ (RL), and there is ongoing research in various sub-fields including ‘semi-supervised’, ‘self-supervised’ and ‘multi-instance’ ML.
- Supervised learning leverages labelled data (annotated information); for example, using labelled X-ray images of known tumours to detect tumours in new images. 15
- ‘Unsupervised learning’ attempts to extract information from data without labels; for example, categorising groups of patients with similar symptoms to identify a common cause. 16
- In RL, computational agents learn by trial and error, or by expert demonstration. The algorithm learns by developing a strategy to maximise rewards. Of note, major breakthroughs in AI in recent years have been based on RL.
- Deep learning (DL) is a class of algorithms that learns by using a large, many-layered collection of connected processes and exposing these processors to a vast set of examples. DL has emerged as the predominant method in AI today driving improvements in areas such as image and speech recognition. 17,18
How to build effective and trusted AI-augmented healthcare systems?
Despite more than a decade of significant focus, the use and adoption of AI in clinical practice remains limited, with many AI products for healthcare still at the design and develop stage. 19–22 While there are different ways to build AI systems for healthcare, far too often there are attempts to force square pegs into round holes ie find healthcare problems to apply AI solutions to without due consideration to local context (such as clinical workflows, user needs, trust, safety and ethical implications).
We hold the view that AI amplifies and augments, rather than replaces, human intelligence. Hence, when building AI systems in healthcare, it is key to not replace the important elements of the human interaction in medicine but to focus it, and improve the efficiency and effectiveness of that interaction. Moreover, AI innovations in healthcare will come through an in-depth, human-centred understanding of the complexity of patient journeys and care pathways.
In Fig Fig1, 1 , we describe a problem-driven, human-centred approach, adapted from frameworks by Wiens et al , Care and Sendak to building effective and reliable AI-augmented healthcare systems. 23–25

Multi-step, iterative approach to build effective and reliable AI-augmented systems in healthcare.
Design and develop
The first stage is to design and develop AI solutions for the right problems using a human-centred AI and experimentation approach and engaging appropriate stakeholders, especially the healthcare users themselves.
Stakeholder engagement and co-creation
Build a multidisciplinary team including computer and social scientists, operational and research leadership, and clinical stakeholders (physician, caregivers and patients) and subject experts (eg for biomedical scientists) that would include authorisers, motivators, financiers, conveners, connectors, implementers and champions. 26 A multi-stakeholder team brings the technical, strategic, operational expertise to define problems, goals, success metrics and intermediate milestones.
Human-centred AI
A human-centred AI approach combines an ethnographic understanding of health systems, with AI. Through user-designed research, first understand the key problems (we suggest using a qualitative study design to understand ‘what is the problem’, ‘why is it a problem’, ‘to whom does it matter’, ‘why has it not been addressed before’ and ‘why is it not getting attention’) including the needs, constraints and workflows in healthcare organisations, and the facilitators and barriers to the integration of AI within the clinical context. After defining key problems, the next step is to identify which problems are appropriate for AI to solve, whether there is availability of applicable datasets to build and later evaluate AI. By contextualising algorithms in an existing workflow, AI systems would operate within existing norms and practices to ensure adoption, providing appropriate solutions to existing problems for the end user.
Experimentation
The focus should be on piloting of new stepwise experiments to build AI tools, using tight feedback loops from stakeholders to facilitate rapid experiential learning and incremental changes. 27 The experiments would allow the trying out of new ideas simultaneously, exploring to see which one works, learn what works and what doesn't, and why. 28 Experimentation and feedback will help to elucidate the purpose and intended uses for the AI system: the likely end users and the potential harm and ethical implications of AI system to them (for instance, data privacy, security, equity and safety).
Evaluate and validate
Next, we must iteratively evaluate and validate the predictions made by the AI tool to test how well it is functioning. This is critical, and evaluation is based on three dimensions: statistical validity, clinical utility and economic utility.
- Statistical validity is understanding the performance of AI on metrics of accuracy, reliability, robustness, stability and calibration. High model performance on retrospective, in silico settings is not sufficient to demonstrate clinical utility or impact.
- To determine clinical utility, evaluate the algorithm in a real-time environment on a hold-out and temporal validation set (eg longitudinal and external geographic datasets) to demonstrate clinical effectiveness and generalisability. 25
- Economic utility quantifies the net benefit relative to the cost from the investment in the AI system.
Scale and diffuse
Many AI systems are initially designed to solve a problem at one healthcare system based on the patient population specific to that location and context. Scale up of AI systems requires special attention to deployment modalities, model updates, the regulatory system, variation between systems and reimbursement environment.
Monitor and maintain
Even after an AI system has been deployed clinically, it must be continually monitored and maintained to monitor for risks and adverse events using effective post-market surveillance. Healthcare organisations, regulatory bodies and AI developers should cooperate to collate and analyse the relevant datasets for AI performance, clinical and safety-related risks, and adverse events. 29
What are the current and future use cases of AI in healthcare?
AI can enable healthcare systems to achieve their ‘quadruple aim’ by democratising and standardising a future of connected and AI augmented care, precision diagnostics, precision therapeutics and, ultimately, precision medicine (Table (Table1 1 ). 30 Research in the application of AI healthcare continues to accelerate rapidly, with potential use cases being demonstrated across the healthcare sector (both physical and mental health) including drug discovery, virtual clinical consultation, disease diagnosis, prognosis, medication management and health monitoring.
Widescale adoption and application of artificial intelligence in healthcare
Timings are illustrative to widescale adoption of the proposed innovation taking into account challenges / regulatory environment / use at scale.
We describe a non-exhaustive suite of AI applications in healthcare in the near term, medium term and longer term, for the potential capabilities of AI to augment, automate and transform medicine.
AI today (and in the near future)
Currently, AI systems are not reasoning engines ie cannot reason the same way as human physicians, who can draw upon ‘common sense’ or ‘clinical intuition and experience’. 12 Instead, AI resembles a signal translator, translating patterns from datasets. AI systems today are beginning to be adopted by healthcare organisations to automate time consuming, high volume repetitive tasks. Moreover, there is considerable progress in demonstrating the use of AI in precision diagnostics (eg diabetic retinopathy and radiotherapy planning).
AI in the medium term (the next 5–10 years)
In the medium term, we propose that there will be significant progress in the development of powerful algorithms that are efficient (eg require less data to train), able to use unlabelled data, and can combine disparate structured and unstructured data including imaging, electronic health data, multi-omic, behavioural and pharmacological data. In addition, healthcare organisations and medical practices will evolve from being adopters of AI platforms, to becoming co-innovators with technology partners in the development of novel AI systems for precision therapeutics.
AI in the long term (>10 years)
In the long term, AI systems will become more intelligent , enabling AI healthcare systems achieve a state of precision medicine through AI-augmented healthcare and connected care. Healthcare will shift from the traditional one-size-fits-all form of medicine to a preventative, personalised, data-driven disease management model that achieves improved patient outcomes (improved patient and clinical experiences of care) in a more cost-effective delivery system.
Connected/augmented care
AI could significantly reduce inefficiency in healthcare, improve patient flow and experience, and enhance caregiver experience and patient safety through the care pathway; for example, AI could be applied to the remote monitoring of patients (eg intelligent telehealth through wearables/sensors) to identify and provide timely care of patients at risk of deterioration.
In the long term, we expect that healthcare clinics, hospitals, social care services, patients and caregivers to be all connected to a single, interoperable digital infrastructure using passive sensors in combination with ambient intelligence. 31 Following are two AI applications in connected care.
Virtual assistants and AI chatbots
AI chatbots (such as those used in Babylon ( www.babylonhealth.com ) and Ada ( https://ada.com )) are being used by patients to identify symptoms and recommend further actions in community and primary care settings. AI chatbots can be integrated with wearable devices such as smartwatches to provide insights to both patients and caregivers in improving their behaviour, sleep and general wellness.
Ambient and intelligent care
We also note the emergence of ambient sensing without the need for any peripherals.
- Emerald ( www.emeraldinno.com ): a wireless, touchless sensor and machine learning platform for remote monitoring of sleep, breathing and behaviour, founded by Massachusetts Institute of Technology faculty and researchers.
- Google nest: claiming to monitor sleep (including sleep disturbances like cough) using motion and sound sensors. 32
- A recently published article exploring the ability to use smart speakers to contactlessly monitor heart rhythms. 33
- Automation and ambient clinical intelligence: AI systems leveraging natural language processing (NLP) technology have the potential to automate administrative tasks such as documenting patient visits in electronic health records, optimising clinical workflow and enabling clinicians to focus more time on caring for patients (eg Nuance Dragon Ambient eXperience ( www.nuance.com/healthcare/ambient-clinical-intelligence.html )).
Precision diagnostics
Diagnostic imaging.
The automated classification of medical images is the leading AI application today. A recent review of AI/ML-based medical devices approved in the USA and Europe from 2015–2020 found that more than half (129 (58%) devices in the USA and 126 (53%) devices in Europe) were approved or CE marked for radiological use. 34 Studies have demonstrated AI's ability to meet or exceed the performance of human experts in image-based diagnoses from several medical specialties including pneumonia in radiology (a convolutional neural network trained with labelled frontal chest X-ray images outperformed radiologists in detecting pneumonia), dermatology (a convolutional neural network was trained with clinical images and was found to classify skin lesions accurately), pathology (one study trained AI algorithms with whole-slide pathology images to detect lymph node metastases of breast cancer and compared the results with those of pathologists) and cardiology (a deep learning algorithm diagnosed heart attack with a performance comparable with that of cardiologists). 35–38
We recognise that there are some exemplars in this area in the NHS (eg University of Leeds Virtual Pathology Project and the National Pathology Imaging Co-operative) and expect widescale adoption and scaleup of AI-based diagnostic imaging in the medium term. 39 We provide two use cases of such technologies.
Diabetic retinopathy screening
Key to reducing preventable, diabetes-related vision loss worldwide is screening individuals for detection and the prompt treatment of diabetic retinopathy. However, screening is costly given the substantial number of diabetes patients and limited manpower for eye care worldwide. 40 Research studies on automated AI algorithms for diabetic retinopathy in the USA, Singapore, Thailand and India have demonstrated robust diagnostic performance and cost effectiveness. 41–44 Moreover, Centers for Medicare & Medicaid Services approved Medicare reimbursement for the use of Food and Drug Administration approved AI algorithm ‘IDx-DR’, which demonstrated 87% sensitivity and 90% specificity for detecting more-than-mild diabetic retinopathy. 45
Improving the precision and reducing waiting timings for radiotherapy planning
An important AI application is to assist clinicians for image preparation and planning tasks for radiotherapy cancer treatment. Currently, segmentation of the images is time consuming and laborious task, performed manually by an oncologist using specially designed software to draw contours around the regions of interest. The AI-based InnerEye open-source technology can cut this preparation time for head and neck, and prostate cancer by up to 90%, meaning that waiting times for starting potentially life-saving radiotherapy treatment can be dramatically reduced (Fig (Fig2 2 ). 46,47

Potential applications for the InnerEye deep learning toolkit include quantitative radiology for monitoring tumour progression, planning for surgery and radiotherapy planning. 47
Precision therapeutics
To make progress towards precision therapeutics, we need to considerably improve our understanding of disease. Researchers globally are exploring the cellular and molecular basis of disease, collecting a range of multimodal datasets that can lead to digital and biological biomarkers for diagnosis, severity and progression. Two important future AI applications include immunomics / synthetic biology and drug discovery.
Immunomics and synthetic biology
Through the application of AI tools on multimodal datasets in the future, we may be able to better understand the cellular basis of disease and the clustering of diseases and patient populations to provide more targeted preventive strategies, for example, using immunomics to diagnose and better predict care and treatment options. This will be revolutionary for multiple standards of care, with particular impact in the cancer, neurological and rare disease space, personalising the experience of care for the individual.
AI-driven drug discovery
AI will drive significant improvement in clinical trial design and optimisation of drug manufacturing processes, and, in general, any combinatorial optimisation process in healthcare could be replaced by AI. We have already seen the beginnings of this with the recent announcements by DeepMind and AlphaFold, which now sets the stage for better understanding disease processes, predicting protein structures and developing more targeted therapeutics (for both rare and more common diseases; Fig Fig3 3 ). 48,49

An overview of the main neural network model architecture for AlphaFold. 49 MSA = multiple sequence alignment.
Precision medicine
New curative therapies.
Over the past decade, synthetic biology has produced developments like CRISPR gene editing and some personalised cancer therapies. However, the life cycle for developing such advanced therapies is still extremely inefficient and expensive.
In future, with better access to data (genomic, proteomic, glycomic, metabolomic and bioinformatic), AI will allow us to handle far more systematic complexity and, in turn, help us transform the way we understand, discover and affect biology. This will improve the efficiency of the drug discovery process by helping better predict early which agents are more likely to be effective and also better anticipate adverse drug effects, which have often thwarted the further development of otherwise effective drugs at a costly late stage in the development process. This, in turn will democratise access to novel advanced therapies at a lower cost.
AI empowered healthcare professionals
In the longer term, healthcare professionals will leverage AI in augmenting the care they provide, allowing them to provide safer, standardised and more effective care at the top of their licence; for example, clinicians could use an ‘AI digital consult’ to examine ‘digital twin’ models of their patients (a truly ‘digital and biomedical’ version of a patient), allowing them to ‘test’ the effectiveness, safety and experience of an intervention (such as a cancer drug) in the digital environment prior to delivering the intervention to the patient in the real world.
We recognise that there are significant challenges related to the wider adoption and deployment of AI into healthcare systems. These challenges include, but are not limited to, data quality and access, technical infrastructure, organisational capacity, and ethical and responsible practices in addition to aspects related to safety and regulation. Some of these issues have been covered, but others go beyond the scope of this current article.
Conclusion and key recommendations
Advances in AI have the potential to transform many aspects of healthcare, enabling a future that is more personalised, precise, predictive and portable. It is unclear if we will see an incremental adoption of new technologies or radical adoption of these technological innovations, but the impact of such technologies and the digital renaissance they bring requires health systems to consider how best they will adapt to the changing landscape. For the NHS, the application of such technologies truly has the potential to release time for care back to healthcare professionals, enabling them to focus on what matters to their patients and, in the future, leveraging a globally democratised set of data assets comprising the ‘highest levels of human knowledge’ to ‘work at the limits of science’ to deliver a common high standard of care, wherever and whenever it is delivered, and by whoever. 50 Globally, AI could become a key tool for improving health equity around the world.
As much as the last 10 years have been about the roll out of digitisation of health records for the purposes of efficiency (and in some healthcare systems, billing/reimbursement), the next 10 years will be about the insight and value society can gain from these digital assets, and how these can be translated into driving better clinical outcomes with the assistance of AI, and the subsequent creation of novel data assets and tools. It is clear that we are at an turning point as it relates to the convergence of the practice of medicine and the application of technology, and although there are multiple opportunities, there are formidable challenges that need to be overcome as it relates to the real world and the scale of implementation of such innovation. A key to delivering this vision will be an expansion of translational research in the field of healthcare applications of artificial intelligence. Alongside this, we need investment into the upskilling of a healthcare workforce and future leaders that are digitally enabled, and to understand and embrace, rather than being intimidated by, the potential of an AI-augmented healthcare system.
Healthcare leaders should consider (as a minimum) these issues when planning to leverage AI for health:
- processes for ethical and responsible access to data: healthcare data is highly sensitive, inconsistent, siloed and not optimised for the purposes of machine learning development, evaluation, implementation and adoption
- access to domain expertise / prior knowledge to make sense and create some of the rules which need to be applied to the datasets (to generate the necessary insight)
- access to sufficient computing power to generate decisions in real time, which is being transformed exponentially with the advent of cloud computing
- research into implementation: critically, we must consider, explore and research issues which arise when you take the algorithm and put it in the real world, building ‘trusted’ AI algorithms embedded into appropriate workflows.
Research articles
Use of progestogens and the risk of intracranial meningioma, delirium and incident dementia in hospital patients, derivation and external validation of a simple risk score for predicting severe acute kidney injury after intravenous cisplatin, quality and safety of artificial intelligence generated health information, large language models and the generation of health disinformation, 25 year trends in cancer incidence and mortality among adults in the uk, cervical pessary versus vaginal progesterone in women with a singleton pregnancy, comparison of prior authorization across insurers, diagnostic accuracy of magnetically guided capsule endoscopy with a detachable string for detecting oesophagogastric varices in adults with cirrhosis, ultra-processed food exposure and adverse health outcomes, added benefit and revenues of oncology drugs approved by the ema, exposure to air pollution and hospital admission for cardiovascular diseases, short term exposure to low level ambient fine particulate matter and natural cause, cardiovascular, and respiratory morbidity, optimal timing of influenza vaccination in young children, effect of exercise for depression, association of non-alcoholic fatty liver disease with cardiovascular disease and all cause death in patients with type 2 diabetes, duration of cpr and outcomes for adults with in-hospital cardiac arrest, clinical effectiveness of an online physical and mental health rehabilitation programme for post-covid-19 condition, atypia detected during breast screening and subsequent development of cancer, publishers’ and journals’ instructions to authors on use of generative ai in academic and scientific publishing, effectiveness of glp-1 receptor agonists on glycaemic control, body weight, and lipid profile for type 2 diabetes, neurological development in children born moderately or late preterm, invasive breast cancer and breast cancer death after non-screen detected ductal carcinoma in situ, all cause and cause specific mortality in obsessive-compulsive disorder, acute rehabilitation following traumatic anterior shoulder dislocation, perinatal depression and risk of mortality, undisclosed financial conflicts of interest in dsm-5-tr, effect of risk mitigation guidance opioid and stimulant dispensations on mortality and acute care visits, update to living systematic review on sars-cov-2 positivity in offspring and timing of mother-to-child transmission, perinatal depression and its health impact, christmas 2023: common healthcare related instruments subjected to magnetic attraction study, using autoregressive integrated moving average models for time series analysis of observational data, demand for morning after pill following new year holiday, christmas 2023: christmas recipes from the great british bake off, effect of a doctor working during the festive period on population health: experiment using doctor who episodes, christmas 2023: analysis of barbie medical and science career dolls, christmas 2023: effect of chair placement on physicians’ behavior and patients’ satisfaction, management of chronic pain secondary to temporomandibular disorders, christmas 2023: projecting complete redaction of clinical trial protocols, christmas 2023: a drug target for erectile dysfunction to help improve fertility, sexual activity, and wellbeing, christmas 2023: efficacy of cola ingestion for oesophageal food bolus impaction, conservative management versus laparoscopic cholecystectomy in adults with gallstone disease, social media use and health risk behaviours in young people, untreated cervical intraepithelial neoplasia grade 2 and cervical cancer, air pollution deaths attributable to fossil fuels, implementation of a high sensitivity cardiac troponin i assay and risk of myocardial infarction or death at five years, covid-19 vaccine effectiveness against post-covid-19 condition, association between patient-surgeon gender concordance and mortality after surgery, intravascular imaging guided versus coronary angiography guided percutaneous coronary intervention, treatment of lower urinary tract symptoms in men in primary care using a conservative intervention, autism intervention meta-analysis of early childhood studies, effectiveness of the live zoster vaccine during the 10 years following vaccination, effects of a multimodal intervention in primary care to reduce second line antibiotic prescriptions for urinary tract infections in women, pyrotinib versus placebo in combination with trastuzumab and docetaxel in patients with her2 positive metastatic breast cancer, association of dcis size and margin status with risk of developing breast cancer post-treatment, racial differences in low value care among older patients in the us, pharmaceutical industry payments and delivery of low value cancer drugs, rosuvastatin versus atorvastatin in adults with coronary artery disease, clinical effectiveness of septoplasty versus medical management for nasal airways obstruction, ultrasound guided lavage with corticosteroid injection versus sham lavage with and without corticosteroid injection for calcific tendinopathy of shoulder, early versus delayed antihypertensive treatment in patients with acute ischaemic stroke, mortality risks associated with floods in 761 communities worldwide, interactive effects of ambient fine particulate matter and ozone on daily mortality in 372 cities, association between changes in carbohydrate intake and long term weight changes, future-case control crossover analysis for adjusting bias in case crossover studies, association between recently raised anticholinergic burden and risk of acute cardiovascular events, suboptimal gestational weight gain and neonatal outcomes in low and middle income countries: individual participant data meta-analysis, efficacy and safety of an inactivated virus-particle vaccine for sars-cov-2, effect of invitation letter in language of origin on screening attendance: randomised controlled trial in breastscreen norway, visits by nurse practitioners and physician assistants in the usa, non-erosive gastro-oesophageal reflux disease and oesophageal adenocarcinoma, venous thromboembolism with use of hormonal contraception and nsaids, food additive emulsifiers and risk of cardiovascular disease, balancing risks and benefits of cannabis use, promoting activity, independence, and stability in early dementia and mild cognitive impairment, effect of home cook interventions for salt reduction in china, cancer mortality after low dose exposure to ionising radiation, effect of a smartphone intervention among university students with unhealthy alcohol use, long term risk of death and readmission after hospital admission with covid-19 among older adults, mortality rates among patients successfully treated for hepatitis c, association between antenatal corticosteroids and risk of serious infection in children, the proportions of term or late preterm births after exposure to early antenatal corticosteroids, and outcomes, safety of ba.4-5 or ba.1 bivalent mrna booster vaccines, comparative effectiveness of booster vaccines among adults aged ≥50 years, third dose vaccine schedules against severe covid-19 during omicron predominance in nordic countries, private equity ownership and impacts on health outcomes, costs, and quality, healthcare disruption due to covid-19 and avoidable hospital admission, educational inequalities in mortality and their mediators among generations across four decades, prevalence and predictors of data and code sharing in the medical and health sciences, medicare eligibility and in-hospital treatment patterns and health outcomes for patients with trauma, therapeutic value of first versus supplemental indications of drugs in us and europe, hospital admissions linked to sars-cov-2 infection in children and adolescents, vitamin d supplementation and major cardiovascular events, menopausal hormone therapy and dementia, associations between modest reductions in kidney function and adverse outcomes in young adults, association between surgeon volume and patient outcomes after elective shoulder replacement surgery, risk prediction of covid-19 related death or hospital admission in adults testing positive for sars-cov-2, effectiveness of grace risk score in patients admitted to hospital with non-st elevation acute coronary syndrome, peer reviewers’ response to invitations by gender and geographical region, breast cancer mortality in women with early invasive breast cancer, follow us on, content links.
- Collections
- Health in South Asia
- Women’s, children’s & adolescents’ health
- News and views
- BMJ Opinion
- Rapid responses
- Editorial staff
- BMJ in the USA
- BMJ in South Asia
- Submit your paper
- BMA members
- Subscribers
- Advertisers and sponsors
Explore BMJ
- Our company
- BMJ Careers
- BMJ Learning
- BMJ Masterclasses
- BMJ Journals
- BMJ Student
- Academic edition of The BMJ
- BMJ Best Practice
- The BMJ Awards
- Email alerts
- Activate subscription
Information
Skip to Content
Google Health research publications
Publishing our work allows us to share ideas and work collaboratively to advance healthcare. This is a comprehensive view of our publications and associated blog posts.
by Ivor Horn
Google Keyword Blog | 2-Nov-2023
by Yossi Mattia Shravya Shetty
Google Keyword Blog | 31-Oct-2023
by Yossi Mattias
Google Keyword Blog | 23-Oct-2023
by Nira Goren
Google Keyword Blog | 18-Oct-2023
by Michaell Howell
Google Keyword Blog | 9-Oct-2023
by Karen DeSalvo
Google Keyword Blog | 3-Oct-2023
Google Keyword Blog | 18-Jul-2023
Blog Posts [more at Google Keyword Blog & Google AI Blog ]
by Susan Thomas
Google Keyword Blog | 5-Jul-2023
Google Keyword Blog | 13-Jun-2023
Google Keyword Blog | 23-May-2023
Google Keyword Blog | 22-May-2023
by Megan Jones Bell
Google Keyword Blog | 15-May-2023
Google Keyword Blog | 13-Apr-2023
Google Keyword Blog | 14-Mar-2023
by Greg Corrado & Yossi Matias
Google AI Blog | 23-Feb-2023
Google Keyword Blog | 26-Jan-2023
by Katie Malczyk
Google Keyword Blog | 17-Jan-2023
Google Keyword Blog | 5-Jan-2023
by Iz Conroy
Google Keyword Blog | 21-Dec-2022
by Hema Budaraju
Google Keyword Blog | 14-Dec-2022
Google Keyword Blog | 15-Nov-2022
by Aashima Gupta
Google Cloud Blog | 14-Nov-2022
by Jeff Dean
Google Keyword Blog | 2-Nov-2022
Google Keyword Blog | 27-Oct-2022
by Riva Sciuto
Google Keyword Blog | 19-Oct-2022
Google Keyword Blog | 12-Sep-2022
by Lauren Winer
Google Keyword Blog | 25-Aug-2022
by Anne Merritt
Google Keyword Blog | 20-Jul-2022
Google Keyword Blog | 17-May-2022
by Megan Jones Bell & Garth Graham
Google Keyword Blog | 10-May-2022
Google Keyword Blog | 2-Dec-2021
by Greg Corrado
Google AI Blog | 24-Mar-2022
Google Keyword Blog | 24-Mar-2022
by Katherine Chou & Sudhi Herle
Android Developers Blog | 24-Mar-2022
by Paul Muret
Google Keyword Blog | 15-Mar-2022
Google Keyword Blog | 8-Mar-2022
Google Research Blog | 11-Jan-2022
by Fred Hersch & Jing Tang
Google Keyword Blog | 8-Dec-2021
Google Keyword Blog | 17-Oct-2021
by Alicia Cormie
Google Keyword Blog | 6-May-2021
Google Keyword Blog | 16-Apr-2021
Google Keyword Blog | 23- Feb-2021
Google Research Blog | 12-Jan-2021
by David Feinberg
LinkedIn Blog | 8-Dec-2020
by Dave Greenwood
Google Keyword Blog | 2-Dec-2020
by Anna Lurchenko
Google Design Blog | 29-Jul-2020
by Daniel Gillison, Jr
Google Keyword Blog | 28-May-2020
Google Research Blog | 9-Jan-2020
by Yun Liu & Po-Hsuan Cameron Chen
Google AI Blog | 10-Dec-2019
Google Keyword Blog | 20-Nov-2019
by Ruth Porat
Google Keyword Blog | 21-Oct-2019
by Dominic King
Google Keyword Blog | 18-Sep-2019
Google Research Blog | 15-Jan-2019
Google Keyword Blog | 17-Jun-2019
by Kent Walter
Google Keyword Blog | 13-Dec-2018
Google Research Blog | 12-Jan-2018
by Paula Schnurr & Teri Brister
Google Keyword Blog | 5-Dec-2017
by Mary Giliberti
Google Keyword Blog | 23-Aug-2017
by Katherine Chou
Google AI Blog | 17-May-2017
Google Research Blog | 12-Jan-2017
COVID-19 Blog Posts
Google Keyword Blog | 16-Jun-2022
COVID-19 Blog Posts [more at Google Keyword Blog ]
by Lauren Gallagher
Google Keyword Blog | 11-Feb-2022
by Tomer Shekel
Google Keyword Blog | 9-Jun-2021
by the COVID Response team, Google India
Google India Blog | 10-May-2021
Google Keyword Blog | 15-Apr-2021 [Spanish version]
by Stephen Ratcliffe
Google Keyword Blog | 24-Feb-2021
by Sundar Pichai
Google Keyword Blog | 25-Jan-2021
by Steph Hannon
Google Keyword Blog | 11-Dec-2020
by Karen DeSalvo & Kristie Canegallo
Google Keyword Blog | 10-Dec-2020
Google Keyword Blog | 24-Nov-2020 [Spanish version]
Google Keyword Blog
10-Nov-2020
Google Keyword Blog | 27-Oct-2020
Google Keyword Blog | 17-Sept-2020
by Mollie Javerbaum & Meghan Houghton
Google Keyword Blog | 10-Sep-2020
by Evgeniy Gabrilovich
Google Keyword Blog | 2-Sep-2020
by Dave Burke
Google Keyword Blog | 31-Jul-2020
by Apple & Google
Google Keyword Blog | 20-May-2020
by Megan Washam
Google Keyword Blog | 13-May-2020
Google Keyword Blog | 8-May-2020
Google Africa Blog
Google Africa Blog | 23-Apr-2020
Google Keyword Blog | 10-Apr-2020
by Julie Black
Google Keyword Blog | 6-Apr-2020
by Jen Fitzpatrick & Karen DeSalvo
Google Keyword Blog | 3-Apr-2020
by Emily Moxley
Google Keyword Blog | 21-Mar-2020
Google Keyword Blog | 15-Mar-2020
Google Keyword Blog | 6-Mar-2020
Lehmann, L. S., Natarajan, V. & Peng, L. Chapter 39
(ed. Krittanawong, C.) Artificial Intelligence in Clinical Practice. 341–344 (Academic Press, 2024).
Deng, C.-Y., Mitani, A., Chen, C. W., Peng, L. H., Hammel, N. & Liu, Y
(eds. Yogesan, K., Goldschmidt, L., Cuadros, J. & Ricur, G.) 199–218. Springer International Publishing, 2023.
Serghiou, S. & Rough, K.
Am. J. Epidemiol. (2023). doi:10.1093/aje/kwad107
DeSalvo Karen B. & Howell Michael D.
NEJM Catalyst non-issue commentary (2023).
DeSalvo, K., Plough, A. L., Castrucci, B., Christopher, G. C. & Palacio, H.
Popul. Health Manag. (2023).
DeSalvo, K. B., Kadakia, K. T. & Chokshi, D. A.
JAMA Health Forum 2, e214051–e214051 (2021).
Kadakia, K. T., Howell, M. D. & DeSalvo, K. B.
JAMA 326, 385–386 (2021).
DeSalvo, K. B. & Kadakia, K. T.
Am. J. Public Health 111, S179–S181 (2021).
Sounderajah, V., Ashrafian, H., Rose, S., Shah, N. H., Ghassemi, M., Golub, R., Kahn, C. E., Jr, Esteva, A., Karthikesalingam, A., Mateen, B., Webster, D., Milea, D., Ting, D., Treanor, D., Cushnan, D., King, D., McPherson, D., Glocker, B., Greaves, F., Harling, L., Ordish, J., Cohen, J. F., Deeks, J., Leeflang, M., Diamond, M., McInnes, M. D. F., McCradden, M., Abràmoff, M. D., Normahani, P., Markar, S. R., Chang, S., Liu, X., Mallett, S., Shetty, S., Denniston, A., Collins, G. S., Moher, D., Whiting, P., Bossuyt, P. M. & Darzi, A.
Nat. Med. (2021).
Chen, P.-H. C., Mermel, C. H. & Liu, Y.
The Lancet Digital Health (2021). doi:10.1016/S2589-7500(21)00216-8
Kelly, C. J., Brown, A. P. Y. & Taylor, J. A.
(eds. Lidströmer, N. & Ashrafian, H.) 1–18 (Springer International Publishing, 2021).
Poplin, R., Zook, J. M. & DePristo, M.
JAMA 326, 268–269 (2021).
Mitani, A., Hammel, N. & Liu, Y.
Nature Biomedical Engineering 1–3 (2021). [readcube]
Esteva, A., Chou, K., Yeung, S., Naik, N., Madani, A., Mottaghi, A., Liu, Y., Topol, E., Dean, J. & Socher, R.
npj Digital Medicine 4, 5 (2021).
Steiner, D. F., Chen, P.-H. C. & Mermel, C. H.
Biochim. Biophys. Acta Rev. Cancer 1875, 188452 (2021).
Liu, Y., Yang, L., Phene, S. & Peng, L.
Artificial Intelligence in Medicine 247–264 (2021).
Warnert, E. A. H., Kasper, L., Meltzer, C. C., Lightfoote, J. B., Bucknor, M. D., Haroon, H., Duggan, G., Gowland, P., Wald, L., Miller, K. L., Morris, E. A. & Anazodo, U. C.
J. Magn. Reson. Imaging (2020). doi:10.1002/jmri.27476 [readcube]
Rakha, E. A., Toss, M., Shiino, S., Gamble, P., Jaroensri, R., Mermel, C. H. & Chen, P.-H. C.
J. Clin. Pathol. (2020). doi:10.1136/jclinpath-2020-206908
Sayres, R., Hammel, N. & Liu, Y.
Annals of Eye Science 5, 18–18 (2020).
Ibrahim, A., Gamble, P., Jaroensri, R., Abdelsamea, M. M., Mermel, C. H., Chen, P.-H. C. & Rakha, E. A.
Breast 49, 267–273 (2020).
Liu, Y., Chen, P.-H. C., Krause, J. & Peng, L.
JAMA 322, 1806–1816 (2019). [readcube]
Kelly, C. J., Karthikesalingam, A., Suleyman, M., Corrado, G., & King, D.
BMC Med. 17, 195 (2019).
Rajkomar, A., Hardt, M., Howell, M. D., Corrado, G., & Chin, M. H.
Ann. Intern. Med. 169(12):866-872 (2018).
Curiel-Lewandrowski, C., Novoa, R. A., Berry, E., Celebi, M. E., Codella, N., Giuste, F., Gutman, D., Halpern, A., Leachman, S., Liu, Y., Liu, Y., Reiter, O. & Tschandl, P.
599–628. Springer New York (2019).
Chen, C. P.-H., Liu, Y., & Peng, L.
Nat. Mater. 18, 410–414 (2019). [readcube]
Rajkomar, A., Dean, J., & Kohane I.
N. Engl. J. Med. 380:1347-1358 (2019).
Esteva, A., Robicquet, A., Ramsundar, B., Kuleshov, V., DePristo, M., Chou, K., Cui, C., Corrado, G., Thrun, S. & Dean, J.
Nat. Med. 25, 24–29 (2019). [readcube]
Rough K, Thompson J.
Ophthalmology. 125(8):1136-1138 (2018).
Wachter, R. M., Howell, M. D.
JAMA 320(1):25-26 (2018).
Cross-Specialty Applied AI
by Krishnamurthy (Dj) Dvijotham & Taylan Cemgil
Google Deepmind | 17-Jul-2023
by Shekoofeh Azizi & Laura Culp
Google AI Blog | 26-Apr-2023
by Alex D’Amour & Katherine Heller
Google AI Blog | 18-Oct-2021
by Shekoofeh Azizi
Google AI Blog | 13-Oct-2021
Publications
Brown, A., Tomasev, N., Freyberg, J., Liu, Y., Karthikesalingam, A. & Schrouff, J.
Nat. Commun. 14, 4314 (2023).
Dvijotham, K., Winkens, J., Barsbey, M., Ghaisas, S., Stanforth, R., Pawlowski, N., Strachan, P., Ahmed, Z., Azizi, S., Bachrach, Y., Culp, L., Daswani, M., Freyberg, J., Kelly, C., Kiraly, A., Kohlberger, T., McKinney, S., Mustafa, B., Natarajan, V., Geras, K., Witowski, J., Qin, Z. Z., Creswell, J., Shetty, S., Sieniek, M., Spitz, T., Corrado, G., Kohli, P., Cemgil, T. & Karthikesalingam, A.
Nat. Med. 1–7 (2023).
Azizi, S., Culp, L., Freyberg, J., Mustafa, B., Baur, S., Kornblith, S., Chen, T., Tomasev, N., Mitrović, J., Strachan, P., Mahdavi, S. S., Wulczyn, E., Babenko, B., Walker, M., Loh, A., Chen, P.-H. C., Liu, Y., Bavishi, P., McKinney, S. M., Winkens, J., Roy, A. G., Beaver, Z., Ryan, F., Krogue, J., Etemadi, M., Telang, U., Liu, Y., Peng, L., Corrado, G. S., Webster, D. R., Fleet, D., Hinton, G., Houlsby, N., Karthikesalingam, A., Norouzi, M. & Natarajan, V.
Nature Biomedical Engineering 1–24 (2023). [readcube]
Schrouff, J., Harris, N., Koyejo, O. O., Alabdulmohsin, I., Schnider, E., Opsahl-Ong, K., Brown, A., Roy, S., Mincu, D., Chen, C., Dieng, A., Liu, Y., Natarajan, V., Karthikesalingam, A., Heller, K. A., Chiappa, S. & D’Amour, A.
NeurIPS (2022).
McKinney, S. M.
medRxiv (2022).
Freeman, B., Hammel, N., Phene, S., Huang, A., Ackermann, R., Kanzheleva, O., Hutson, M., Taggart, C., Duong, Q. & Sayres, R.
HCOMP 9, 60–71 (2021).
Azizi, S., Mustafa, B., Ryan, F., Beaver, Z., Freyberg, J., Deaton, J., Loh, A., Karthikesalingam, A., Kornblith, S., Chen, T., Natarajan, V. & Norouzi, M.
Proceedings of the IEEE/CVF International Conference on Computer Vision (ICCV) 3478–3488 (2021).
Sadilek, A., Liu, L., Nguyen, D., Kamruzzaman, M., Serghiou, S., Rader, B., Ingerman, A., Mellem, S., Kairouz, P., Nsoesie, E. O., MacFarlane, J., Vullikanti, A., Marathe, M., Eastham, P., Brownstein, J. S., Arcas, B. A. Y., Howell, M. D. & Hernandez, J.
NPJ Digit Med 4, 132 (2021).
Mustafa, B., Loh, A., Freyberg, J., MacWilliams, P., Karthikesalingam, A., Houlsby, N. & Natarajan, V.
arXiv [cs.CV] (2021).
arXiv [eess.IV] (2021).
D’Amour, A., Heller, K., Moldovan, D., Adlam, B., Alipanahi, B., Beutel, A., Chen, C., Deaton, J., Eisenstein, J., Hoffman, M. D., Hormozdiari, F., Houlsby, N., Hou, S., Jerfel, G., Karthikesalingam, A., Lucic, M., Ma, Y., McLean, C., Mincu, D., Mitani, A., Montanari, A., Nado, Z., Natarajan, V., Nielson, C., Osborne, T. F., Raman, R., Ramasamy, K., Sayres, R., Schrouff, J., Seneviratne, M., Sequeira, S., Suresh, H., Veitch, V., Vladymyrov, M., Wang, X., Webster, K., Yadlowsky, S., Yun, T., Zhai, X. & Sculley, D.
arXiv [cs.LG] (2020).
Winkens, J., Bunel, R., Roy, A. G., Stanforth, R., Natarajan, V., Ledsam, J. R., MacWilliams, P., Kohli, P., Karthikesalingam, A., Kohl, S., Cemgil, T., Ali Eslami, S. M. & Ronneberger, O.
Hartman, T., Howell, M., Dean, J., Hoory, S., Slyper, R., Laish, I., Gilon, O, Vainstein, D., Corrado, G., Chou, K., Po, M., Williams, J., Ellis, S., Bee, G., Hassidim, A., Amira, R., Beryozkin, G., Szpektor, I., & Matias, Y.
BMC (2020).
Dermatology
by Lou Wang
Google Keyword Blog | 14-Jun-2023
Google Keyword Blog | 08-Feb-2022
by Abhijit Guha Roy & Jie Ren
Google AI Blog | 27-Jan-2022
by Miles Hutson & Aaron Loh
TensorFlow Blog | 11-Oct-2021
by Peggy Bui & Yuan Liu
Google Keyword Blog | 18-May-2021
by Ayush Jain & Peggy Bui
Google Keyword Blog | 28-Apr-2021
by Timo Kohlberger & Yuan Liu
Google AI Blog | 19-Feb-2020
by Yuan Liu & Peggy Bui
Google AI Blog | 12-Sep-2019
Alabdulmohsin, I.M., Schrouff, J., Koyejo, S.
35. NeurIPS (2022).
Vemulapalli, R., Morningstar, W. R., Mansfield, P. A., Eichner, H., Singhal, K., Afkanpour, A. & Green, B.
arXiv [cs.LG] (2022).
Huang, S. J., Liu, Y., Kanada, K., Corrado, G. S., Webster, D. R., Peng, L., Bui, P. & Liu, Y.
Skin Health and Disease (2021).
Guha Roy, A., Ren, J., Azizi, S., Loh, A., Natarajan, V., Mustafa, B., Pawlowski, N., Freyberg, J., Liu, Y., Beaver, Z., Vo, N., Bui, P., Winter, S., MacWilliams, P., Corrado, G. S., Telang, U., Liu, Y., Cemgil, T., Karthikesalingam, A., Lakshminarayanan, B. & Winkens, J.
Med. Image Analysis. 75, 102274 (2021). [ reading link ]
Weng, W.-H., Deaton, J., Natarajan, V., Elsayed, G. F. & Liu, Y.
JAMA Netw Open 4, e217249–e217249 (2021).
Machine Learning for Health NeurIPS Workshop (ML4H), PMLR 136:415-429 (2020).
Singh, N., Lee, K., Coz, D., Angermueller, C., Huang, S., Loh, A. & Liu, Y.
in 2020 IEEE/CVF Conference on Computer Vision and Pattern Recognition Workshops (CVPRW) 3172–3181 (2020).
Liu, Y., Jain, A., Eng, C., Way, D. H., Lee, K., Bui, P., Kanada, K., de Oliveira Marinho, G., Gallegos, J., Gabriele, S., Gupta, V., Singh, N., Natarajan, V., Hofmann-Wellenhof, R., Corrado, G. S., Peng, L. H., Webster, D. R., Ai, D., Huang, S., Liu, Y., Carter Dunn, R. & Coz, D.
Nat. Med. (2020). [readcube]
Ghorbani, A., Natarajan, V., Coz, D. & Liu, Y.
Machine Learning for Health NeurIPS Workshop (ML4H), PMLR 116:155-170 (2020).
Eng, C., Liu, Y. & Bhatnagar, R.
Br. J. Dermatol. (2019). readcube
by Yossi Matias & Ehud Rivlin
Google AI Blog | 5-Aug-2021
by Robin Suchan
Verily Press | 5-Aug-2021
by Daniel Freedman & Ehud Rivlin
Google AI Blog | 28-Aug-2020
Golany, T., Aides, A., Freedman, D., Rabani, N., Liu, Y., Rivlin, E., Corrado, G. S., Matias, Y., Khoury, W., Kashtan, H. & Reissman, P.
Surg. Endosc. (2022).
Livovsky, D. M., Veikherman, D., Golany, T., Aides, A., Dashinsky, V., Rabani, N., Ben Shimol, D., Blau, Y., Katzir, L., Shimshoni, I., Liu, Y., Segol, O., Goldin, E., Corrado, G., Lachter, J., Matias, Y., Rivlin, E. & Freedman, D.
Gastrointest. Endosc. (2021).
Freedman, D., Blau, Y., Katzir, L., Aides, A., Shimshoni, I., Veikherman, D., Golany, T., Gordon, A., Corrado, G., Matias, Y. & Rivlin, E.
IEEE Trans. Med. Imaging 1–1 (2020).
Epidemiological Forecasting
by Joel Shor & Sercan Arik
by Tomas Pfister
Google Cloud Blog | 15-Nov-2020
by Dario Sava
Google Cloud Blog | 3-Aug-2020
Tsai, T. C., Arik, S., Jacobson, B. H., Yoon, J., Yoder, N., Sava, D., Mitchell, M., Graham, G. & Pfister, T.
NPJ Digit Med 5, 59 (2022).
Cramer, E. Y., Ray, E. L., Lopez, V. K., Bracher, J., Brennen, A., Castro Rivadeneira, A. J., Gerding, A., Gneiting, T., House, K. H., Huang, Y., Jayawardena, D., Kanji, A. H., Khandelwal, A., Le, K., Mühlemann, A., Niemi, J., Shah, A., Stark, A., Wang, Y., Wattanachit, N., Zorn, M. W., Gu, Y., Jain, S., Bannur, N., Deva, A., Kulkarni, M., Merugu, S., Raval, A., Shingi, S., Tiwari, A., White, J., Abernethy, N. F., Woody, S., Dahan, M., Fox, S., Gaither, K., Lachmann, M., Meyers, L. A., Scott, J. G., Tec, M., Srivastava, A., George, G. E., Cegan, J. C., Dettwiller, I. D., England, W. P., Farthing, M. W., Hunter, R. H., Lafferty, B., Linkov, I., Mayo, M. L., Parno, M. D., Rowland, M. A., Trump, B. D., Zhang-James, Y., Chen, S., Faraone, S. V., Hess, J., Morley, C. P., Salekin, A., Wang, D., Corsetti, S. M., Baer, T. M., Eisenberg, M. C., Falb, K., Huang, Y., Martin, E. T., McCauley, E., Myers, R. L., Schwarz, T., Sheldon, D., Gibson, G. C., Yu, R., Gao, L., Ma, Y., Wu, D., Yan, X., Jin, X., Wang, Y.-X., Chen, Y., Guo, L., Zhao, Y., Gu, Q., Chen, J., Wang, L., Xu, P., Zhang, W., Zou, D., Biegel, H., Lega, J., McConnell, S., Nagraj, V. P., Guertin, S. L., Hulme-Lowe, C., Turner, S. D., Shi, Y., Ban, X., Walraven, R., Hong, Q.-J., Kong, S., van de Walle, A., Turtle, J. A., Ben-Nun, M., Riley, S., Riley, P., Koyluoglu, U., DesRoches, D., Forli, P., Hamory, B., Kyriakides, C., Leis, H., Milliken, J., Moloney, M., Morgan, J., Nirgudkar, N., Ozcan, G., Piwonka, N., Ravi, M., Schrader, C., Shakhnovich, E., Siegel, D., Spatz, R., Stiefeling, C., Wilkinson, B., Wong, A., Cavany, S., España, G., Moore, S., Oidtman, R., Perkins, A., Kraus, D., Kraus, A., Gao, Z., Bian, J., Cao, W., Lavista Ferres, J., Li, C., Liu, T.-Y., Xie, X., Zhang, S., Zheng, S., Vespignani, A., Chinazzi, M., Davis, J. T., Mu, K., Pastore Y Piontti, A., Xiong, X., Zheng, A., Baek, J., Farias, V., Georgescu, A., Levi, R., Sinha, D., Wilde, J., Perakis, G., Bennouna, M. A., Nze-Ndong, D., Singhvi, D., Spantidakis, I., Thayaparan, L., Tsiourvas, A., Sarker, A., Jadbabaie, A., Shah, D., Della Penna, N., Celi, L. A., Sundar, S., Wolfinger, R., Osthus, D., Castro, L., Fairchild, G., Michaud, I., Karlen, D., Kinsey, M., Mullany, L. C., Rainwater-Lovett, K., Shin, L., Tallaksen, K., Wilson, S., Lee, E. C., Dent, J., Grantz, K. H., Hill, A. L., Kaminsky, J., Kaminsky, K., Keegan, L. T., Lauer, S. A., Lemaitre, J. C., Lessler, J., Meredith, H. R., Perez-Saez, J., Shah, S., Smith, C. P., Truelove, S. A., Wills, J., Marshall, M., Gardner, L., Nixon, K., Burant, J. C., Wang, L., Gao, L., Gu, Z., Kim, M., Li, X., Wang, G., Wang, Y., Yu, S., Reiner, R. C., Barber, R., Gakidou, E., Hay, S. I., Lim, S., Murray, C., Pigott, D., Gurung, H. L., Baccam, P., Stage, S. A., Suchoski, B. T., Prakash, B. A., Adhikari, B., Cui, J., Rodríguez, A., Tabassum, A., Xie, J., Keskinocak, P., Asplund, J., Baxter, A., Oruc, B. E., Serban, N., Arik, S. O., Dusenberry, M., Epshteyn, A., Kanal, E., Le, L. T., Li, C.-L., Pfister, T., Sava, D., Sinha, R., Tsai, T., Yoder, N., Yoon, J., Zhang, L., Abbott, S., Bosse, N. I., Funk, S., Hellewell, J., Meakin, S. R., Sherratt, K., Zhou, M., Kalantari, R., Yamana, T. K., Pei, S., Shaman, J., Li, M. L., Bertsimas, D., Skali Lami, O., Soni, S., Tazi Bouardi, H., Ayer, T., Adee, M., Chhatwal, J., Dalgic, O. O., Ladd, M. A., Linas, B. P., Mueller, P., Xiao, J., Wang, Y., Wang, Q., Xie, S., Zeng, D., Green, A., Bien, J., Brooks, L., Hu, A. J., Jahja, M., McDonald, D., Narasimhan, B., Politsch, C., Rajanala, S., Rumack, A., Simon, N., Tibshirani, R. J., Tibshirani, R., Ventura, V., Wasserman, L., O’Dea, E. B., Drake, J. M., Pagano, R., Tran, Q. T., Ho, L. S. T., Huynh, H., Walker, J. W., Slayton, R. B., Johansson, M. A., Biggerstaff, M. & Reich, N. G.
Proc. Natl. Acad. Sci. U. S. A. 119, e2113561119 (2022).
Arık, S. Ö., Shor, J., Sinha, R., Yoon, J., Ledsam, J. R., Le, L. T., Dusenberry, M. W., Yoder, N. C., Popendorf, K., Epshteyn, A., Euphrosine, J., Kanal, E., Jones, I., Li, C.-L., Luan, B., Mckenna, J., Menon, V., Singh, S., Sun, M., Ravi, A. S., Zhang, L., Sava, D., Cunningham, K., Kayama, H., Tsai, T., Yoneoka, D., Nomura, S., Miyata, H. & Pfister, T.
NPJ Digit Med 4, 146 (2021).
Kapoor, A., Ben, X., Liu, L., Perozzi, B., Barnes, M., Blais, M. & O’Banion, S.
Arik, Li, Yoon, Sinha, Epshteyn, Le, Menon, Singh, Zhang, Nikoltchev, Sonthalia, Nakhost, Kanal & Pfister.
Adv. Neural Inf. Process. Syst. 2020.
Eye Diseases
by Kasumi Widner
Google Keyword Blog | 30-May-2023
by Angus Turner
Google Australia Blog | 7-Mar-2023
by Emma Beede
Google Keyword Blog | 25-Apr-2020
by Kasumi Widner & Sunny Virmani
Google Keyword Blog | 25-Feb-2019
Verily Blog | 25-Feb-2019
by Rory Sayres & Jonathan Krause
Google AI Blog | 13-Dec-2018
by Mustafa Suleyman
DeepMind Blog | 13-Aug-2018
by Lily Peng
Google Keyword Blog | 29-Nov-2016
by Lily Peng & Varun Gulshan
Google AI Blog | 29-Nov-2016
Widner, K., Virmani, S., Krause, J., Nayar, J., Tiwari, R., Pedersen, E. R., Jeji, D., Hammel, N., Matias, Y., Corrado, G. S., Liu, Y., Peng, L. & Webster, D. R.
Nat. Med. 1–3 (2023). [readcube]
Srisubat, A., Kittrongsiri, K., Sangroongruangsri, S., Khemvaranan, C., Shreibati, J. B., Ching, J., Hernandez, J., Tiwari, R., Hersch, F., Liu, Y., Hanutsaha, P., Ruamviboonsuk, V., Turongkaravee, S., Raman, R. & Ruamviboonsuk, P.
Ophthalmol Ther (2023).
Chia, M. A., Hersch, F., Sayres, R., Bavishi, P., Tiwari, R., Keane, P. A. & Turner, A. W.
Br. J. Ophthalmol. (2023).
Ruamviboonsuk, P., Tiwari, R., Sayres, R., Nganthavee, V., Hemarat, K., Kongprayoon, A., Raman, R., Levinstein, B., Liu, Y., Schaekermann, M., Lee, R., Virmani, S., Widner, K., Chambers, J., Hersch, F., Peng, L. & Webster, D. R.
The Lancet Digital Health (2022).
Pedersen Elin Rønby, Cuadros Jorge, Khan Mahbuba, Fleischmann Sybille, Wolff Gregory, Hammel Naama, Liu Yun & Leung Geoffrey.
NEJM Catalyst (2021).
Wilson, M., Chopra, R., Wilson, M. Z., Cooper, C., MacWilliams, P., Liu, Y., Wulczyn, E., Florea, D., Hughes, C. O., Karthikesalingam, A., Khalid, H., Vermeirsch, S., Nicholson, L., Keane, P. A., Balaskas, K. & Kelly, C. J.
JAMA Ophthalmol. (2021).
Limwattanayingyong, J., Nganthavee, V., Seresirikachorn, K., Singalavanija, T., Soonthornworasiri, N., Ruamviboonsuk, V., Rao, C., Raman, R., Grzybowski, A., Schaekermann, M., Peng, L. H., Webster, D. R., Semturs, C., Krause, J., Sayres, R., Hersch, F., Tiwari, R., Liu, Y. & Ruamviboonsuk, P.
Journal of Diabetes Research, 1–8 (2020).
Hsu J, Phene S, Mitani A, Luo J, Hammel N, Krause J, Sayres R.
in ACM-CHIL [arXiv] (2020)
Bresnick, G., Cuadros, J. A., Khan, M., Fleischmann, S., Wolff, G., Limon, A., Chang, J., Jiang, L., Cuadros, P. & Pedersen, E. R.
BMJ Open Diabetes Research and Care 8, e001154 (2020).
Beede, E., Baylor, E., Hersch, F., Iurchenko, A., Wilcox, L., Ruamviboonsuk, P. & Vardoulakis, L. M.
Proceedings of the 2020 CHI Conference on Human Factors in Computing Systems 1–12. Association for Computing Machinery (2020).
Schaekermann, M., Cai, C. J., Huang, A. E. & Sayres, R.
Proceedings of the 2020 CHI Conference on Human Factors in Computing Systems 1–13. Association for Computing Machinery (2020).
Phene, S., Dunn, R. C., Hammel, N., Liu, Y., Krause, J., Kitade, N., Schaekermann, M., Sayres, R., Wu, D. J., Bora, A., Semturs, C., Misra, A., Huang, A. E., Spitze, A., Medeiros, F. A., Maa, A. Y., Gandhi, M., Corrado, G. S., Peng, L. & Webster, D. R.
Ophthalmology 126, 1627–1639 (2019).
Schaekermann, M., Hammel, N., Terry, M., Ali, T. K., Liu, Y., Basham, B., Campana, B., Chen, W., Ji, X., Krause, J., Corrado, G. S., Peng, L., Webster, D. R., Law, E. & Sayres, R.
Transl. Vis. Sci. Technol. 8, 40 (2019).
Gulshan, V., Rajan, R. P., Widner, K., Wu, D., Wubbels, P., Rhodes, T., Whitehouse, K., Coram, M., Corrado, G., Ramasamy, K., Raman, R., Peng, L. & Webster, D. R.
JAMA Ophthalmol. (2019).
Ruamviboonsuk, P., Krause, J., Chotcomwongse, P., Sayres, R., Raman, R., Widner, K., Campana, B. J. L., Phene, S., Hemarat, K., Tadarati, M., Silpa-Archa, S., Limwattanayingyong, J., Rao, C., Kuruvilla, O., Jung, J., Tan, J., Orprayoon, S., Kangwanwongpaisan, C., Sukumalpaiboon, R., Luengchaichawang, C., Fuangkaew, J., Kongsap, P., Chualinpha, L., Saree, S., Kawinpanitan, S., Mitvongsa, K., Lawanasakol, S., Thepchatri, C., Wongpichedchai, L., Corrado, G. S., Peng, L. & Webster, D. R.
npj Digit Med 2, 25 (2019).
Sayres, R., Taly, A., Rahimy, E., Blumer, K., Coz, D., Hammel, N., Krause, J., Narayanaswamy, A., Rastegar, Z., Wu, D., Xu, S., Barb, S., Joseph, A., Shumski, M., Smith, J., Sood, A. B., Corrado, G. S., Peng, L. & Webster, D. R.
Ophthalmology 126, 552–564 (2019).
Fauw, J., Ledsam, J.R., Romera-Paredes, B., Nikolov, S., Tomasev, N., Blackwell, S., Askham, H., Glorot, X., O’Donoghue, B., Visentin, D., van den Driessche, G., Lakshminarayanan, B., Meyer, C., Mackinder, F., Bouton, S., Ayoub, K., Chopra, R., King, D., Karthikesalingam, A., Hughes, C.O., Raine, R., Hughes, J., Sim, D. A., Egan, C., Tufail, A., Montgomery, H., Hassabis, D., Rees, G., Back, T., Khaw, P.T., Suleyman, M., Cornebise, J., Keane, P.A., & Ronneberger, O.
Nat. Med. 24, 1342–1350 (2018). [readcube]
Krause, J., Gulshan, V., Rahimy, E., Karth, P., Widner, K., Corrado, G. S., Peng, L., & Webster, D.R.
Ophthalmology 125, 1264–1272 (2018). [arXiv]
Bouskill, K., Smith-Morris, C., Bresnick, G., Cuadros, J. & Pedersen, E. R.
BMC Health Services Research 18, (2018).
Smith-Morris, C., Bresnick, G. H., Cuadros, J., Bouskill, K. E. & Pedersen, E. R.
Med. Anthropol. 39, 109–122 (2018).
Guan, M., Gulshan, V., Dai, A, Hinton, G.
AAAI Conference on Artificial Intelligence (2018).
Gulshan, V., Peng, L., Coram, M., Stumpe, M. C., Wu, D., Narayanaswamy, A., Venugopalan, S., Widner, K., Madams, T., Cuadros, J., Ramasamy, K., Nelson, P., Mega, J., & Webster, D.
JAMA 316, 2402–2410 (2016).
Blog Posts [more at Fitbit Blog ]
by Amy McDonough
Google Keyword Blog | 26-Oct-2023
by Molly McHugh-Johnson
Google Keyword Blog | 19-Oct-2023
by Sandeep Waraich
Google Keyword Blog | 4-Oct-2023
by TJ Varghese
Google Keyword Blog | 28-Sep-2023
by Maggie Stanphill & Bhanu Narasimhan
Google Keyword Blog | 1-Aug-2023
by Elena Perez & Samy Abdel-Ghaffer
Google Keyword Blog | 2-Jun-2023
by Elena Perez
Google Keyword Blog | 4-May-2023
Google Keyword Blog | 16-Mar-2023
by Zahra Barnes
Google Keyword Blog | 20-Dec-2022
Google Keyword Blog | 6-Oct-2022
Google Keyword Blog | 29-Sep-2022
by The Fitbit Team
Google Keyword Blog | 24-Aug-2022
Google Keyword Blog | 22-Jun-2022
Google Keyword Blog | 11-Apr-2022
Speed, C., Arneil, T., Harle, R., Wilson, A., Karthikesalingam, A., McConnell, M. & Phillips, J.
PLOS Digit Health 2, e0000236 (2023).
Lubitz, S. A., Faranesh, A. Z., Selvaggi, C., Atlas, S. J., McManus, D. D., Singer, D. E., Pagoto, S., McConnell, M. V., Pantelopoulos, A. & Foulkes, A. S.
Circulation (2022).
Natarajan, A., Su, H.-W. & Heneghan, C.
Front. Physiol. 13, 898251 (2022).
Natarajan, A., Su, H.-W., Heneghan, C., Blunt, L., O’Connor, C. & Niehaus, L.
NPJ Digit Med 4, 136 (2021).
Blog Posts [more at DeepVariant Blog ]
by Andrew Carroll
Google Keyword Blog | 10-May-2023
by Andrew Caroll & Kishwar Shafin
Google AI Blog | 10-May-2023
Babak Behsaz & Andrew Carroll
Google AI Blog | 27-Apr-2023
by Andrew Caroll
Google Keyword Blog | 26-Oct-2022
by Andrew Carroll & Pi-Chuan Chang
Google Keyword Blog | 13-Jan-2022
by Farhad Hormozdiari & Andrew Carroll
Google Open Source Blog | 11-Jan-2022
by Andrew Carroll & Cory McLean
Google AI Blog | 23-Jun-2021
Google AI Blog | 18-Sep-2020
by Pi-Chuan Chang & Lizzie Dorfman
Google AI Blog | 19-Apr-2018
by Mark DePristo & Ryan Poplin
Google AI Blog | 4-Dec-2017
by Phing Lee
Google Keyword Blog | 17-Nov-2017
Rhie, A., Nurk, S., Cechova, M., Hoyt, S. J., Taylor, D. J., Altemose, N., Hook, P. W., Koren, S., Rautiainen, M., Alexandrov, I. A., Allen, J., Asri, M., Bzikadze, A. V., Chen, N.-C., Chin, C.-S., Diekhans, M., Flicek, P., Formenti, G., Fungtammasan, A., Garcia Giron, C., Garrison, E., Gershman, A., Gerton, J. L., Grady, P. G. S., Guarracino, A., Haggerty, L., Halabian, R., Hansen, N. F., Harris, R., Hartley, G. A., Harvey, W. T., Haukness, M., Heinz, J., Hourlier, T., Hubley, R. M., Hunt, S. E., Hwang, S., Jain, M., Kesharwani, R. K., Lewis, A. P., Li, H., Logsdon, G. A., Lucas, J. K., Makalowski, W., Markovic, C., Martin, F. J., Mc Cartney, A. M., McCoy, R. C., McDaniel, J., McNulty, B. M., Medvedev, P., Mikheenko, A., Munson, K. M., Murphy, T. D., Olsen, H. E., Olson, N. D., Paulin, L. F., Porubsky, D., Potapova, T., Ryabov, F., Salzberg, S. L., Sauria, M. E. G., Sedlazeck, F. J., Shafin, K., Shepelev, V. A., Shumate, A., Storer, J. M., Surapaneni, L., Taravella Oill, A. M., Thibaud-Nissen, F., Timp, W., Tomaszkiewicz, M., Vollger, M. R., Walenz, B. P., Watwood, A. C., Weissensteiner, M. H., Wenger, A. M., Wilson, M. A., Zarate, S., Zhu, Y., Zook, J. M., Eichler, E. E., O’Neill, R. J., Schatz, M. C., Miga, K. H., Makova, K. D. & Phillippy, A. M.
Nature (2023).
Liao, W.-W., Asri, M., Ebler, J., Doerr, D., Haukness, M., Hickey, G., Lu, S., Lucas, J. K., Monlong, J., Abel, H. J., Buonaiuto, S., Chang, X. H., Cheng, H., Chu, J., Colonna, V., Eizenga, J. M., Feng, X., Fischer, C., Fulton, R. S., Garg, S., Groza, C., Guarracino, A., Harvey, W. T., Heumos, S., Howe, K., Jain, M., Lu, T.-Y., Markello, C., Martin, F. J., Mitchell, M. W., Munson, K. M., Mwaniki, M. N., Novak, A. M., Olsen, H. E., Pesout, T., Porubsky, D., Prins, P., Sibbesen, J. A., Sirén, J., Tomlinson, C., Villani, F., Vollger, M. R., Antonacci-Fulton, L. L., Baid, G., Baker, C. A., Belyaeva, A., Billis, K., Carroll, A., Chang, P.-C., Cody, S., Cook, D. E., Cook-Deegan, R. M., Cornejo, O. E., Diekhans, M., Ebert, P., Fairley, S., Fedrigo, O., Felsenfeld, A. L., Formenti, G., Frankish, A., Gao, Y., Garrison, N. A., Giron, C. G., Green, R. E., Haggerty, L., Hoekzema, K., Hourlier, T., Ji, H. P., Kenny, E. E., Koenig, B. A., Kolesnikov, A., Korbel, J. O., Kordosky, J., Koren, S., Lee, H., Lewis, A. P., Magalhães, H., Marco-Sola, S., Marijon, P., McCartney, A., McDaniel, J., Mountcastle, J., Nattestad, M., Nurk, S., Olson, N. D., Popejoy, A. B., Puiu, D., Rautiainen, M., Regier, A. A., Rhie, A., Sacco, S., Sanders, A. D., Schneider, V. A., Schultz, B. I., Shafin, K., Smith, M. W., Sofia, H. J., Abou Tayoun, A. N., Thibaud-Nissen, F., Tricomi, F. F., Wagner, J., Walenz, B., Wood, J. M. D., Zimin, A. V., Bourque, G., Chaisson, M. J. P., Flicek, P., Phillippy, A. M., Zook, J. M., Eichler, E. E., Haussler, D., Wang, T., Jarvis, E. D., Miga, K. H., Garrison, E., Marschall, T., Hall, I. M., Li, H. & Paten, B.
Nature 617, 312–324 (2023).
Cosentino, J., Behsaz, B., Alipanahi, B., McCaw, Z. R., Hill, D., Schwantes-An, T.-H., Lai, D., Carroll, A., Hobbs, B. D., Cho, M. H., McLean, C. Y. & Hormozdiari, F.
Nat. Genet. (2023).
Belyaeva, A., Shor, J., Cook, D. E., Shafin, K., Liu, D., Töpfer, A., Wenger, A. M., Rowell, W. J., Yang, H., Kolesnikov, A., McLean, C. Y., Nattestad, M., Carroll, A. & Chang, P.-C.
Yuan, B., McLean, C. Y., Hormozdiari, F. I. & Cosentino, J.
Baid, G., Cook, D. E., Shafin, K., Yun, T., Llinares-López, F., Berthet, Q., Belyaeva, A., Töpfer, A., Wenger, A. M., Rowell, W. J., Yang, H., Kolesnikov, A., Ammar, W., Vert, J.-P., Vaswani, A., McLean, C. Y., Nattestad, M., Chang, P.-C. & Carroll, A.
Nat. Biotechnol. (2022).
Wagner, J., Olson, N. D., Harris, L., Khan, Z., Farek, J., Mahmoud, M., Stankovic, A., Kovacevic, V., Yoo, B., Miller, N., Rosenfeld, J. A., Ni, B., Zarate, S., Kirsche, M., Aganezov, S., Schatz, M. C., Narzisi, G., Byrska-Bishop, M., Clarke, W., Evani, U. S., Markello, C., Shafin, K., Zhou, X., Sidow, A., Bansal, V., Ebert, P., Marschall, T., Lansdorp, P., Hanlon, V., Mattsson, C.-A., Barrio, A. M., Fiddes, I. T., Xiao, C., Fungtammasan, A., Chin, C.-S., Wenger, A. M., Rowell, W. J., Sedlazeck, F. J., Carroll, A., Salit, M. & Zook, J. M.
Cell Genom 2, (2022).
Markello, C., Huang, C., Rodriguez, A., Carroll, A., Chang, P.-C., Eizenga, J., Markello, T., Haussler, D. & Paten, B.
Genome Res. 32, 893–903 (2022).
Goenka, S. D., Gorzynski, J. E., Shafin, K., Fisk, D. G., Pesout, T., Jensen, T. D., Monlong, J., Chang, P.-C., Baid, G., Bernstein, J. A., Christle, J. W., Dalton, K. P., Garalde, D. R., Grove, M. E., Guillory, J., Kolesnikov, A., Nattestad, M., Ruzhnikov, M. R. Z., Samadi, M., Sethia, A., Spiteri, E., Wright, C. J., Xiong, K., Zhu, T., Jain, M., Sedlazeck, F. J., Carroll, A., Paten, B. & Ashley, E. A.
Gorzynski, J. E., Goenka, S. D., Shafin, K., Jensen, T. D., Fisk, D. G., Grove, M. E., Spiteri, E., Pesout, T., Monlong, J., Baid, G., Bernstein, J. A., Ceresnak, S., Chang, P.-C., Christle, J. W., Chubb, H., Dalton, K. P., Dunn, K., Garalde, D. R., Guillory, J., Knowles, J. W., Kolesnikov, A., Ma, M., Moscarello, T., Nattestad, M., Perez, M., Ruzhnikov, M. R. Z., Samadi, M., Setia, A., Wright, C., Wusthoff, C. J., Xiong, K., Zhu, T., Jain, M., Sedlazeck, F. J., Carroll, A., Paten, B. & Ashley, E. A.
N. Engl. J. Med. (2022).
Gorzynski, J. E., Goenka, S. D., Shafin, K., Jensen, T. D., Fisk, D. G., Grove, M. E., Spiteri, E., Pesout, T., Monlong, J., Bernstein, J. A., Ceresnak, S., Chang, P.-C., Christle, J. W., Chubb, H., Dunn, K., Garalde, D. R., Guillory, J., Ruzhnikov, M. R. Z., Wright, C., Wusthoff, C. J., Xiong, K., Hollander, S. A., Berry, G. J., Jain, M., Sedlazeck, F. J., Carroll, A., Paten, B. & Ashley, E. A.
Circ Genom Precis Med 15, e003591 (2022).
Sirén, J., Monlong, J., Chang, X., Novak, A. M., Eizenga, J. M., Markello, C., Sibbesen, J. A., Hickey, G., Chang, P.-C., Carroll, A., Gupta, N., Gabriel, S., Blackwell, T. W., Ratan, A., Taylor, K. D., Rich, S. S., Rotter, J. I., Haussler, D., Garrison, E. & Paten, B.
Science 374 (2021).
McCaw, Z. R., Colthurst, T., Yun, T., Furlotte, N. A., Carroll, A., Alipanahi, B., McLean, C. Y. & Hormozdiari, F.
Nat. Commun. 13, 241 (2022).
O’Connell, J., Yun, T., Moreno, M., Li, H., Litterman, N., Kolesnikov, A., Noblin, E., Chang, P.-C.,Shastri, A., Dorfman, E. H., Shringarpure, S., Auton, A., Carroll, A. & McLean, C. Y.
Communications Biology 4, 1–9 (2021).
Shafin, K., Pesout, T., Chang, P.-C., Nattestad, M., Kolesnikov, A., Goel, S., Baid, G., Kolmogorov, M., Eizenga, J. M., Miga, K. H., Carnevali, P., Jain, M., Carroll, A. & Paten, B.
Nat. Methods 18, 1322–1332 (2021). [readcube]
Baid, G., Cook, D. E., Shafin, K., Yun, T., Llinares-López, F., Berthet, Q., Wenger, A. M., Rowell, W. J., Nattestad, M., Yang, H., Kolesnikov, A., Töpfer, A., Ammar, W., Vert, J.-P., Vaswani, A., McLean, C. Y., Chang, P.-C. & Carroll, A.
bioRxiv 2021.08.31.458403 (2021).
Alipanahi, B., Hormozdiari, F., Behsaz, B., Cosentino, J., McCaw, Z. R., Schorsch, E., Sculley, D., Dorfman, E. H., Foster, P. J., Peng, L. H., Phene, S., Hammel, N., Carroll, A., Khawaja, A. P. & McLean, C. Y.
Am. J. Hum. Genet. (2021).
Yun, T., Li, H., Chang, P-C., Lin, M., Carroll, A., & McLean, C. Y.
Bioinformatics 36, 5582-5589 (2021).
Yadlowsky, S., Yun, T., McLean, C. & D’Amour, A.
arXiv [stat.ML] (2021).
Alipanahi, B., Hormozdiari, F., Behsaz, B., Cosentino, J., McCaw, Z. R., Schorsch, E., Sculley, D., Dorfman, E. H., Phene, S., Hammel, N., Carroll, A., Khawaja, A. P. & McLean, C. Y.
arXiv [q-bio.GN] (2020).
McLean, C. Y., Hwang, Y., Poplin, R. & DePristo, M. A.
Bioinformatics 35, 4389–4391 (2019).
Wenger, A. M., Peluso, P., Rowell, W. J., Chang, P.-C., Hall, R. J., Concepcion, G. T., Ebler, J., Fungtammasan, A., Kolesnikov, A., Olson, N. D., Töpfer, A., Alonge, M., Mahmoud, M., Qian, Y., Chin, C.-S., Phillippy, A. M., Schatz, M. C., Myers, G., DePristo, M. A., Ruan, J., Marschall, T., Sedlazeck, F. J., Zook, J. M., Li, H., Koren, S., Carroll, A., Rank, D. R. & Hunkapiller, M. W.
Nat. Biotechnol. 37, 1155–1162 (2019). [readcube]
Poplin, R., Chang, P.-C., Alexander, D., Schwartz, S., Colthurst, T., Ku, A., Newburger, D., Dijamco, J., Nguyen, N., Afshar, P. T., Gross, S. S., Dorfman, L., McLean, C. Y. & DePristo, M. A.
Nat. Biotechnol. 36, 983–987 (2018). [readcube]
Telenti, A., Lippert, C., Chang, P.-C. & DePristo, M.
Hum. Mol. Genet. 27, R63–R71 (2018).
Kelley, D. R., Reshef, Y. A., Bileschi, M., Belanger, D., McLean, C. Y. & Snoek, J.
Genome Res. 28, 739–750 (2018).
Google Health Studies
by Nicholas Allen
Google Keyword Blog | 23-May-2022
by Jon Morgan & Paul Eastham
Google Keyword Blog | 9-Dec-2020
Health Sensors and Signals
by Xiaoran "Van" Fan & Trausti Thormundsson
Google AI Blog | 27-Oct-2023
by Michael Dixon & Reena Singhal Lee
Google AI Blog | 9-Nov-2021
by Ashton Udall
Google Keyword Blog | 16-Mar-2021
Google AI Blog | 16-Mar-2021
by Shwetak Patel
Google Keyword Blog | 4-Feb-2021
Fan, X., Pearl, D., Howard, R., Shangguan, L. & Thormundsson, T. APG.
Proceedings of the 29th Annual International Conference on Mobile Computing and Networking 1–15. Association for Computing Machinery (2023).
Yang, Y., Liu, X., Wu, J., Borac, S., Katabi, D., Poh, M.-Z. & McDuff, D.
arXiv [cs.LG] (ICLR 2023).
Bae, S., Borac, S., Emre, Y., Wang, J., Wu, J., Kashyap, M., Kang, S.-H., Chen, L., Moran, M., Cannon, J., Teasley, E. S., Chai, A., Liu, Y., Wadhwa, N., Krainin, M., Rubinstein, M., Maciel, A., McConnell, M. V., Patel, S., Corrado, G. S., Taylor, J. A., Zhan, J. & Po, M. J.
bioRxiv (2021).
Dixon, M., Schneider, L. D., Yu, J., Hsu, J., Pathak, A., Shin, D., Lee, R. S., Malhotra, M., Mixter, K., McConnell, M. V., Taylor, J. A., Patel, S. N.,
Google Whitepaper (2021).
Large Language Models
Blog Posts [more at Med-PaLM site ]
by Michael Howell
by Aashima Gupta & Greg Corrado
Google Cloud Blog | 29-Aug-2023
by Greg Corrado and Yossi Matias
Google AI Blog | 3-Aug-2023
by James Manyika & Jeff Dean
Google Keyword Blog | 25-May-2023
by Aashima Gupta & Amy Waldron
Google Cloud Blog | 13-April-2023
Galatzer-Levy, I. R., McDuff, D., Natarajan, V., Karthikesalingam, A. & Malgaroli, M.
arXiv [cs.CL] (2023).
Xu, S., Yang, L., Kelly, C., Sieniek, M., Kohlberger, T., Ma, M., Weng, W.-H., Kiraly, A., Kazemzadeh, S., Melamed, Z., Park, J., Strachan, P., Liu, Y., Lau, C., Singh, P., Chen, C., Etemadi, M., Kalidindi, S. R., Matias, Y., Chou, K., Corrado, G. S., Shetty, S., Tse, D., Prabhakara, S., Golden, D., Pilgrim, R., Eswaran, K. & Sellergren, A. ELIXR:
arXiv [cs.CV] (2023).
Tu, T., Azizi, S., Driess, D., Schaekermann, M., Amin, M., Chang, P.-C., Carroll, A., Lau, C., Tanno, R., Ktena, I., Mustafa, B., Chowdhery, A., Liu, Y., Kornblith, S., Fleet, D., Mansfield, P., Prakash, S., Wong, R., Virmani, S., Semturs, C., Sara Mahdavi, S., Green, B., Dominowska, E., Aguera y Arcas, B., Barral, J., Webster, D., Corrado, G. S., Matias, Y., Singhal, K., Florence, P., Karthikesalingam, A. & Natarajan, V.
Belyaeva, A., Cosentino, J., Hormozdiari, F., Eswaran, K., Shetty, S., Corrado, G., Carroll, A., McLean, C. Y. & Furlotte, N. A.
arXiv [q-bio.QM] (2023).
Liu, X., McDuff, D., Kovacs, G., Galatzer-Levy, I., Sunshine, J., Zhan, J., Poh, M.-Z., Liao, S., Di Achille, P. & Patel, S.
Singhal, K., Tu, T., Gottweis, J., Sayres, R., Wulczyn, E., Hou, L., Clark, K., Pfohl, S., Cole-Lewis, H., Neal, D., Schaekermann, M., Wang, A., Amin, M., Lachgar, S., Mansfield, P., Prakash, S., Green, B., Dominowska, E., Aguera y Arcas, B., Tomasev, N., Liu, Y., Wong, R., Semturs, C., Sara Mahdavi, S., Barral, J., Webster, D., Corrado, G. S., Matias, Y., Azizi, S., Karthikesalingam, A. & Natarajan, V.
Singhal, K., Azizi, S., Tu, T., Sara Mahdavi, S., Wei, J., Chung, H. W., Scales, N., Tanwani, A., Cole-Lewis, H., Pfohl, S., Payne, P., Seneviratne, M., Gamble, P., Kelly, C., Scharli, N., Chowdhery, A., Mansfield, P., Aguera y Arcas, B., Webster, D., Corrado, G. S., Matias, Y., Chou, K., Gottweis, J., Tomasev, N., Liu, Y., Rajkomar, A., Barral, J., Semturs, C., Karthikesalingam, A. & Natarajan, V.
Medical Audio
by Laurent El Shafey and Izhak Shafran
16-Aug-2019
by Julie Cattiau
Google Keyword Blog | 7-May-2019
by Katherine Chou and Chung-Cheng Chiu
Google AI Blog | 21-Nov-2017
Shafran, I., Du, N., Tran, L., Perry, A., Keyes, L., Knichel, M., Domin, A., Huang, L., Chen, Y., Li, G., Wang, M., El Shafey, L., Soltau, H. & Paul, J. S.
Proceedings of the Language Resources and Evaluation Conference. arXiv [cs.CL] (2020).
Du, N., Chen, K., Kannan, A., Tran, L., Chen, Y. & Shafran, I.
Proceedings of the Annual Meeting of the Association of Computational Linguistics. arXiv [cs.LG] (2019).
Rajkomar, A., Kannan, A., Chen, K., Vardoulakis, L., Chou, K., Cui, C., & Dean, J.
JAMA Intern. Med. 179, 836–838 (2019).
El Shafey, L., Soltau, H. & Shafran, I.
Proceedings of Interspeech. arXiv [cs.CL] (2019).
Du, N., Wang, M., Tran, L., Li, G. & Shafran, I.
Proc. Empirical Methods in Natural Language Processing. arXiv [cs.CL] (2019).
Chiu, C.-C., Tripathi, A., Chou, K., Co, C., Jaitly, N., Jaunzeikare, D., Kannan, A., Nguyen, P., Sak, H., Sankar, A., Tansuwan, J., Wan, N., Wu, Y., & Zhang X.
arXiv [cs.CL] (2017).
Medical Records
by Alvin Rajkoma and Eric Loreaux
Google AI Blog | 24-Jan-2023
by Jinsung Yoon and Sercan O. Arik
Google AI Blog | 21-Dec-2022
by Subhrajit Roy & Diana Mincu
Google AI Blog | 22-Jul-2021
by Kathryn Rough & Alvin Rajkomar
Google AI Blog | 2-Apr-2020
by Alvin Rajkomar & Eyal Oren
Google AI Blog | 22-Jan-2019
by Demis Hassabis & Mustafa Suleyman & Dominic King
DeepMind Blog | 13-Nov-2018
Google AI Blog | 8-May-2018
by Patrik Sundberg & Eyal Oren
Google AI Blog | 2-Mar-2018
Seneviratne, M. G., Li, R. C., Schreier, M., Lopez-Martinez, D., Patel, B. S., Yakubovich, A., Kemp, J. B., Loreaux, E., Gamble, P., El-Khoury, K., Vardoulakis, L., Wong, D., Desai, J., Chen, J. H., Morse, K. E., Downing, N. L., Finger, L. T., Chen, M.-J. & Shah, N.
BMJ Health Care Inform 29, (2022).
Loreaux, E., Yu, K., Kemp, J., Seneviratne, M., Chen, C., Roy, S., Protsyuk, I., Harris, N., D’Amour, A., Yadlowsky, S. & Chen, M.-J.
Rajkomar, A., Loreaux, E., Liu, Y., Kemp, J., Li, B., Chen, M.-J., Zhang, Y., Mohiuddin, A. & Gottweis, J.
Nat. Commun. 13, 7456 (2022).
Roy, S., Mincu, D., Loreaux, E., Mottram, A., Protsyuk, I., Harris, N., Xue, Y., Schrouff, J., Montgomery, H., Connell, A., Tomasev, N., Karthikesalingam, A. & Seneviratne, M.
J. Am. Med. Inform. Assoc. (2021).
Tomašev, N., Harris, N., Baur, S., Mottram, A., Glorot, X., Rae, J. W., Zielinski, M., Askham, H., Saraiva, A., Magliulo, V., Meyer, C., Ravuri, S., Protsyuk, I., Connell, A., Hughes, C. O., Karthikesalingam, A., Cornebise, J., Montgomery, H., Rees, G., Laing, C., Baker, C. R., Osborne, T. F., Reeves, R., Hassabis, D., King, D., Suleyman, M., Back, T., Nielson, C., Seneviratne, M. G., Ledsam, J. R. & Mohamed, S.
Nat. Protoc. 1–23 (2021). [readcube]
Xue Y, Du N, Mottram A, Seneviratne A, Dai AM.
NeurIPS (2020).
Xue Y, Zhou D, Du N, Dai A, Xu Z, Zhang K, Cui C.
KDD (2020).
Choi E, Xu Z, Li Y, Dusenberry MW, Flores G, Xue Y, Dai AM.
AAAI (2020).
Dusenberry MW, Tran D, Choi E, Kemp J, Nixon J, Jerfel G, Heller K, & Dai AM.
ACM CHIL (2020).
Hardt M, Rajkomar A, Flores G, Dai A, Howell M, Corrado G, Cui C, & Hardt M.
Rough, K., Dai, A. M., Zhang, K., Xue, Y., Vardoulakis, L. M., Cui, C., Butte, A. J., Howell, M. D. & Rajkomar, A.
Clin. Pharmacol. Ther. 108, 145–154 (2020).
Tomašev, N., Glorot, X., Rae, J. W., Zielinski, M., Askham, H., Saraiva, A., Mottram, A., Meyer, C., Ravuri, S., Protsyuk, I., Connell, A., Hughes, C. O., Karthikesalingam, A., Cornebise, J., Montgomery, H., Rees, G., Laing, C., Baker, C. R., Peterson, K., Reeves, R., Hassabis, D., King, D., Suleyman, M., Back, T., Nielson, C., Ledsam, J. R. & Mohamed, S.
Nature 572, 116–119 (2019). [readcube]
Connell, A., Montgomery, H., Martin, P., Nightingale, C., Sadeghi-Alavijeh, O., King, D., Karthikesalingam, A., Hughes, C., Back, T., Ayoub, K., Suleyman, M., Jones, G., Cross, J., Stanley, S., Emerson, M., Merrick, C., Rees, G., Laing, C. & Raine, R.
npj Digit Med 2, 67 (2019).
Connell A., Raine R., Martin P., Barbosa E.C., Morris S., Nightingale C., Sadeghi-Alavijeh O., King D., Karthikesalingam A., Hughes C., Back T., Ayoub K., Suleyman M., Jones G., Cross J., Stanley S., Emerson M., Merrick C., Rees G., Montgomery H., & Laing C.
J Med Internet Res 21(7):e13147 (2019).
Connell A, Black G, Montgomery H, Martin P, Nightingale C, King D, Karthikesalingam A, Hughes C, Back T, Ayoub K, Suleyman M, Jones G, Cross J, Stanley S, Emerson M, Merrick C, Rees G, Laing C, & Raine R.
J Med Internet Res 21(7):e13143 (2019).
Kemp J, Rajkomar A, & Dai AM.
arXiv [cs.LG] (2019).
Pfohl SR, Dai AM, & Heller K.
Xue Y, Zhou D, Du N, Dai AM, Xu Z, Zhang K,& Cui C.
Zhang K, Xue Y, Flores G, Rajkomar A, Cui C, & Dai AM.
Rajkomar A, Oren E, Chen K, Dai AM, Hajaj N, Hardt M, Liu PJ, Liu X, Marcus J, Sun M, Sundberg P, Yee H, Zhang K, Zhang Y, Flores G, Duggan GE, Irvine J, Le Q, Litsch K, Mossin A, Tansuwan J, Wang, Wexler J, Wilson J, Ludwig D, Volchenboum SL, Chou K, Pearson M, Madabushi S, Shah NH, Butte AJ, Howell MD, Cui C, Corrado GS, Dean J.
npj Digital Med 1, 18 (2018).
Novel Biomarkers
by Sara Ahadi & Andrew Carroll
Google AI Blog | 11-Apr-2023
by Boris Babenko & Akib Uddin
Google AI Blog | 24-Mar-2023
by Boris Babenko & Naama Hammel
by Terry Spitz & Jim Winkens
Google Keyword Blog | 18-May-2020
by Jason Yim, Reena Chopra, Jeffrey De Fauw & Joseph Ledsam
DeepMind Blog | 18-May-2020
by Akinori Mitani
Google Keyword Blog | 28-Jan-2020
Google AI Blog | 2-Feb-2018
Ahadi, S., Wilson, K. A., Jr, Babenko, B., McLean, C. Y., Bryant, D., Pritchard, O., Kumar, A., Carrera, E. M., Lamy, R., Stewart, J. M., Varadarajan, A., Berndl, M., Kapahi, P. & Bashir, A.
Elife 12, (2023).
Babenko, B., Traynis, I., Chen, C., Singh, P., Uddin, A., Cuadros, J., Daskivich, L. P., Maa, A. Y., Kim, R., Kang, E. Y.-C., Matias, Y., Corrado, G. S., Peng, L., Webster, D. R., Semturs, C., Krause, J., Varadarajan, A. V., Hammel, N. & Liu, Y.
The Lancet Digital Health (2023).
Babenko, B., Mitani, A., Traynis, I., Kitade, N., Singh, P., Maa, A. Y., Cuadros, J., Corrado, G. S., Peng, L., Webster, D. R., Varadarajan, A., Hammel, N. & Liu, Y.
Nat Biomed Eng (2022).
Liu, X., Ali, T. K., Singh, P., Shah, A., McKinney, S. M., Ruamviboonsuk, P., Turner, A. W., Keane, P. A., Chotcomwongse, P., Nganthavee, V., Chia, M., Huemer, J., Cuadros, J., Raman, R., Corrado, G. S., Peng, L., Webster, D. R., Hammel, N., Varadarajan, A. V., Liu, Y., Chopra, R. & Bavishi, P.
Ophthalmol Retina (2022).
Mitani, A., Traynis, I., Singh, P., Corrado, G. S., Webster, D. R., Peng, L. H., Varadarajan, A. V., Liu, Y. & Hammel, N.
doi:10.1101/2021.12.30.21268488
Bora, A., Balasubramanian, S., Babenko, B., Virmani, S., Venugopalan, S., Mitani, A., de Oliveira Marinho, G., Cuadros, J., Ruamviboonsuk, P., Corrado, G. S., Peng, L., Webster, D. R., Varadarajan, A. V., Hammel, N., Liu, Y. & Bavishi, P.
The Lancet Digital Health (2020). doi:10.1016/S2589-7500(20)30250-8
Moraes, G., Fu, D. J., Wilson, M., Khalid, H., Wagner, S. K., Korot, E., Ferraz, D., Faes, L., Kelly, C. J., Spitz, T., Patel, P. J., Balaskas, K., Keenan, T. D. L., Keane, P. A. & Chopra, R.
Ophthalmology (2020). doi:10.1016/j.ophtha.2020.09.025
Narayanaswamy, A., Venugopalan, S., Webster, D. R., Peng, L., Corrado, G. S., Ruamviboonsuk, P., Bavishi, P., Brenner, M., Nelson, P. C. & Varadarajan, A. V.
Medical Image Computing and Computer Assisted Intervention – MICCAI 2020 273–283 (2020). doi:10.1007/978-3-030-59710-8_27 arXiv
Yim, J., Chopra, R., Spitz, T., Winkens, J., Obika, A., Kelly, C., Askham, H., Lukic, M., Huemer, J., Fasler, K., Moraes, G., Meyer, C., Wilson, M., Dixon, J., Hughes, C., Rees, G., Khaw, P. T., Karthikesalingam, A., King, D., Hassabis, D., Suleyman, M., Back, T., Ledsam, J. R., Keane, P. A. & De Fauw, J.
Varadarajan, A. V., Bavishi, P., Ruamviboonsuk, P., Chotcomwongse, P., Venugopalan, S., Narayanaswamy, A., Cuadros, J., Kanai, K., Bresnick, G., Tadarati, M., Silpa-Archa, S., Limwattanayingyong, J., Nganthavee, V., Ledsam, J. R., Keane, P. A., Corrado, G. S., Peng, L. & Webster, D. R.
Nat. Commun. 11, 130 (2020).
Mitani, A., Huang, A., Venugopalan, S., Corrado, G. S., Peng, L., Webster, D. R., Hammel, N., Liu, Y. & Varadarajan, A. V.
Nat Biomed Eng (2019). [readcube]
Babenko, B., Balasubramanian, S., Blumer, K. E., Corrado, G. S., Peng, L., Webster, D. R., Hammel, N. & Varadarajan, A. V.
arXiv [cs.CV] (2019).
aradarajan, A.V., Poplin, R., Blumer, K., Angermueller, C., Lesdam, J., Chopra, R., Keane, P.A., Corrado, G. S., Peng, L., Webster, D. R.
Invest. Ophthalmol. Vis. Sci. 59, 2861–2868 (2018).
Poplin, R., Varadarajan, A. V., Blumer, K., Liu, Y., McConnell, M. V., Corrado, G. S., Peng, L., & Webster, D. R.
Nat. Biomed. Eng. 2, 158–164 (2018). [readcube]
Open Health Stack
by Richa Tiwari
Open Health Stack Blog | 10-Oct-2023.
Open Health Stack Blog | 2-Sep-2023.
by Abirami Sukumaran & Omar Ismail
Google Cloud Blog | 17-Aug-2023.
by Fred Hersch
Google Keyword Blog | 14-Mar-2023.
Google Open Source Blog | 17-May-2023
by Justin Krogue, Yun Liu, Po-Hsuan Cameron Chen & Ellery A
Nature Portfolio Health Community Blog | 10-May-2023
by Ellery Wulczyn and Yun Liu
Google AI Blog | 14-Mar-2023
Google Cloud Blog | 12-Dec-2022
Verily Blog | 16-Mar-2022
by Po-Hsuan Cameron Chen & Maggie Demkin
Google AI Blog | 11-Feb-2022
by Po-Hsuan Cameron Chen & Yun Liu
Google Keyword Blog | 23-Sept-2021
by People + AI Research
People + AI Research Blog | 14-May-2021
by Dave Steiner, Yun Liu, Craig Mermel, Kurt Zatloukal, Heimo Muller, Markus Plass
npj Digital Medicine Blog | 19-Apr-2021
Google Cloud Blog | 2-Sep-2020
by Kunal Nagpal & Craig Mermel
Google Keyword Blog | 23-Jul-2020
by Narayan Hedge & Carrie Cai
Google AI Blog | 19-July-2019
by Martin Stumpe & Craig Mermel
Google AI Blog | 16-Nov-2018
Google AI Blog | 12-Oct-2018
Google AI Blog | 16-Apr-2018
by Martin Stumpe & Lily Peng
Google AI Blog | 3-Mar-2017
Krogue, J. D., Azizi, S., Tan, F., Flament-Auvigne, I., Brown, T., Plass, M., Reihs, R., Müller, H., Zatloukal, K., Richeson, P., Corrado, G. S., Peng, L. H., Mermel, C. H., Liu, Y., Chen, P.-H. C., Gombar, S., Montine, T., Shen, J., Steiner, D. F. & Wulczyn, E.
Commun. Med. 3, 59 (2023).
L’Imperio, V., Wulczyn, E., Plass, M., Müller, H., Tamini, N., Gianotti, L., Zucchini, N., Reihs, R., Corrado, G. S., Webster, D. R., Peng, L. H., Chen, P.-H. C., Lavitrano, M., Liu, Y., Steiner, D. F., Zatloukal, K. & Pagni, F.
JAMA Netw Open 6, e2254891 (2023).
Jaroensri, R., Wulczyn, E., Hegde, N., Brown, T., Flament-Auvigne, I., Tan, F., Cai, Y., Nagpal, K., Rakha, E. A., Dabbs, D. J., Olson, N., Wren, J. H., Thompson, E. E., Seetao, E., Robinson, C., Miao, M., Beckers, F., Corrado, G. S., Peng, L. H., Mermel, C. H., Liu, Y., Steiner, D. F. & Chen, P.-H. C.
npj Breast Cancer 8, 1–12 (2022).
Bulten, W., Kartasalo, K., Chen, P.-H. C., Ström, P., Pinckaers, H., Nagpal, K., Cai, Y., Steiner, D. F., van Boven, H., Vink, R., Hulsbergen-van de Kaa, C., van der Laak, J., Amin, M. B., Evans, A. J., van der Kwast, T., Allan, R., Humphrey, P. A., Grönberg, H., Samaratunga, H., Delahunt, B., Tsuzuki, T., Häkkinen, T., Egevad, L., Demkin, M., Dane, S., Tan, F., Valkonen, M., Corrado, G. S., Peng, L., Mermel, C. H., Ruusuvuori, P., Litjens, G. & Eklund, M.
Nat. Med. 1–10 (2022).
Sadhwani, A., Chang, H.-W., Behrooz, A., Brown, T., Auvigne-Flament, I., Patel, H., Findlater, R., Velez, V., Tan, F., Tekiela, K., Wulczyn, E., Yi, E. S., Mermel, C. H., Hanks, D., Chen, P.-H. C., Kulig, K., Batenchuk, C., Steiner, D. F. & Cimermancic, P.
Sci. Rep. 11, 1–11 (2021).
Gamble, P., Jaroensri, R., Wang, H., Tan, F., Moran, M., Brown, T., Flament-Auvigne, I., Rakha, E. A., Toss, M., Dabbs, D. J., Regitnig, P., Olson, N., Wren, J. H., Robinson, C., Corrado, G. S., Peng, L. H., Liu, Y., Mermel, C. H., Steiner, D. F. & Chen, P.-H. C.
Communications Medicine 1, 1–12 (2021).
Wulczyn, E., Nagpal, K., Symonds, M., Moran, M., Plass, M., Reihs, R., Nader, F., Tan, F., Cai, Y., Brown, T., Flament-Auvigne, I., Amin, M. B., Stumpe, M. C., Müller, H., Regitnig, P., Holzinger, A., Corrado, G. S., Peng, L. H., Chen, P.-H. C., Steiner, D. F., Zatloukal, K., Liu, Y. & Mermel, C. H.
Communications Medicine 1, 1–8 (2021).
Cai, C.J., Steiner, D., Wilcox, L., Terry, M. and Winter, S.
Proceedings of the ACM SIGCHI Conference on Human Factors in Computing Systems, ACM (2021).
Wulczyn, E., Steiner, D. F., Moran, M., Plass, M., Reihs, R., Tan, F., Flament-Auvigne, I., Brown, T., Regitnig, P., Chen, P.-H. C., Hegde, N., Sadhwani, A., MacDonald, R., Ayalew, B., Corrado, G. S., Peng, L. H., Tse, D., Müller, H., Xu, Z., Liu, Y., Stumpe, M. C., Zatloukal, K. & Mermel, C. H.
npj Digital Medicine 4, 1–13 (2021).
Steiner, D. F., Nagpal, K., Sayres, R., Foote, D. J., Wedin, B. D., Pearce, A., Cai, C. J., Winter, S. R., Symonds, M., Yatziv, L., Kapishnikov, A., Brown, T., Flament-Auvigne, I., Tan, F., Stumpe, M. C., Jiang, P.-P., Liu, Y., Chen, P.-H. C., Corrado, G. S., Terry, M. & Mermel, C. H.
JAMA Netw Open 3, e2023267–e2023267 (2020).
Nagpal, K., Foote, D., Tan, F., Liu, Y., Chen, P.-H. C., Steiner, D. F., Manoj, N., Olson, N., Smith, J. L., Mohtashamian, A., Peterson, B., Amin, M. B., Evans, A. J., Sweet, J. W., Cheung, C., van der Kwast, T., Sangoi, A. R., Zhou, M., Allan, R., Humphrey, P. A., Hipp, J. D., Gadepalli, K., Corrado, G. S., Peng, L. H., Stumpe, M. C. & Mermel, C. H.
JAMA Oncol (2020).
Wulczyn, E., Steiner, D. F., Xu, Z., Sadhwani, A., Wang, H., Flament-Auvigne, I., Mermel, C. H., Chen, P.-H. C., Liu, Y. & Stumpe, M. C.
PLOS ONE 15, e0233678 (2020).
Kohlberger, T., Liu, Y., Moran, M., Chen, P.-H. C., Brown, T., Hipp, J. D., Mermel, C. H. & Stumpe, M. C.
J. Pathol. Inform. 10, 39 (2019).
Chen, P.C., Gadepalli, K., MacDonald, R., Liu, Y., Kadowaki, S., Nagpal, K., Kohlberger, T., Dean, J., Corrado, G.S., Hipp, J.D., Mermel, C.H., Stumpe, M. C.
Nat Med 25, 1453–1457 (2019). [readcube]
Liu, Y., Kohlberger, T., Norouzi, M., Dahl, G. E., Smith, J. L., Mohtashamian, A., Olson, N., Peng, L.H., Hipp, J.D., Stumpe, M.C. (2019).
Arch. Pathol. Lab. Med. 143, 859–868 (2019).
Hegde, N., Hipp, J. D., Liu, Y., Emmert-Buck, M., Reif, E., Smilkov, D., Terry, M., Cai, C. J., Amin, M. B., Mermel, C. H., Nelson, P. Q., Peng, L. H., Corrado, G. S. & Stumpe, M. C.
npj Digit Med 2, 56 (2019).
Cai, C.J., Winter, S., Steiner, D., Wilcox, L. and Terry, M.
Proceedings of the ACM on Human-computer Interaction, 3(CSCW), pp.1-24 (2019)
Cai, C.J., Reif, E., Hegde, N., Hipp, J., Kim, B., Smilkov, D., Wattenberg, M., Viegas, F., Corrado, G.S., Stumpe, M.C. and Terry, M.
In Proceedings of the 2019 CHI Conference on Human Factors in Computing Systems (pp. 1-14) (2019).
Nagpal, K., Foote, D., Liu, Y., Chen, P.H.C., Wulczyn, E., Tan, F., Olson, N., Smith, J.L., Mohtashamian, A., Wren, J.H., Corrado, G.S., MacDonald, R., Peng, L. H., Amin, M.B., Evans, A.J., Sanjoi, A.R., Mermel, C. H., Hipp, J. D., Stumpe, M. C.
npj Digit. Med. 2, 48 (2019).
Steiner, D. F., MacDonald, R., Liu, Y., Truszkowski, P., Hipp, J. D., Gammage, C., Thng, F., Peng, L., Stumpe, M.C.
Am. J. Surg. Pathol. 42, 1636–1646 (2018).
Liu, Y., Gadepalli, K., Norouzi, M., Dahl, G.E., Kohlberger, T., Boyko, A., Venugopalan, S., Timofeev, A., Nelson, P.Q., Corrado, G.S. and Hipp, J.D., Peng, L., Stumpe, M. C.
arXiv preprint arXiv:1703.02442 (2017).
Public & Environmental Health
Blog Posts [more above in "COVID-19 blog posts”]
by Charlotte Stanton
Google Africa Blog | 9-May-2023
by Kate Brandt
Google Keyword Blog | 29-Mar-2023
by Adam Sadilek & Xerxes Dotiwalla
Google AI Blog | 12-Nov-2019
Gligorić, K., Kamath, C., Weiss, D. J., Bavadekar, S., Liu, Y., Shekel, T., Schulman, K. & Gabrilovich, E.
Communications Medicine 3, 1–11 (2023).
Macharia, P. M., Wong, K. L. M., Olubodun, T., Beňová, L., Stanton, C., Sundararajan, N., Shah, Y., Prasad, G., Kansal, M., Vispute, S., Shekel, T., Gwacham-Anisiobi, U., Ogunyemi, O., Wang, J., Abejirinde, I.-O. O., Makanga, P. T., Afolabi, B. B. & Banke-Thomas, A.
Sci Data 10, 736 (2023).
Veneri, P., Kaufmann, T., Vispute, S., Shekel, T., Gabrilovich, E., Wellenius, G. A., Dijkstra, L. & Kansal, M.
(Organisation for Economic Co-Operation and Development (OECD), 2023).
Weintraub, R. L., Miller, K., Rader, B., Rosenberg, J., Srinath, S., Woodbury, S. R., Schultheiss, M. D., Kansal, M., Vispute, S., Serghiou, S., Flores, G., Kumok, A., Shekel, T., Gabrilovich, E., Ahmad, I., Chiang, M. E. & Brownstein, J. S.
Am. J. Public Health e1–e5 (2023).
Vaidyanathan, U., Sun, Y., Shekel, T., Chou, K., Galea, S., Gabrilovich, E. & Wellenius, G. A.
Sci. Rep. 12, 8946 (2022). [readcube]
Wahltinez, O., Cheung, A., Alcantara, R., Cheung, D., Daswani, M., Erlinger, A., Lee, M., Yawalkar, P., Lê, P., Navarro, O. P., Brenner, M. P. & Murphy, K.
Sci Data 9, 162 (2022).
Bavadekar, S., Boulanger, A., Davis, J., Desfontaines, D., Gabrilovich, E., Gadepalli, K., Ghazi, B., Griffith, T., Gupta, J., Kamath, C., Kraft, D., Kumar, R., Kumok, A., Mayer, Y., Manurangsi, P., Patankar, A., Perera, I. M., Scott, C., Shekel, T., Miller, B., Smith, K., Stanton, C., Sun, M., Young, M. & Wellenius, G.
arXiv [cs.CR] (2021).
Woskie, L. R., Hennessy, J., Espinosa, V., Tsai, T. C., Vispute, S., Jacobson, B. H., Cattuto, C., Gauvin, L., Tizzoni, M., Fabrikant, A., Gadepalli, K., Boulanger, A., Pearce, A., Kamath, C., Schlosberg, A., Stanton, C., Bavadekar, S., Abueg, M., Hogue, M., Oplinger, A., Chou, K., Corrado, G., Shekel, T., Jha, A. K., Wellenius, G. A. & Gabrilovich, E.
PLoS One 16, e0253071 (2021).
Wellenius, G. A., Vispute, S., Espinosa, V., Fabrikant, A., Tsai, T. C., Hennessy, J., Dai, A., Williams, B., Gadepalli, K., Boulanger, A., Pearce, A., Kamath, C., Schlosberg, A., Bendebury, C., Mandayam, C., Stanton, C., Bavadekar, S., Pluntke, C., Desfontaines, D., Jacobson, B. H., Armstrong, Z., Gipson, B., Wilson, R., Widdowson, A., Chou, K., Oplinger, A., Shekel, T., Jha, A. K. & Gabrilovich, E.
Nat. Commun. 12, 3118 (2021).
Venkatramanan, S., Sadilek, A., Fadikar, A., Barrett, C. L., Biggerstaff, M., Chen, J., Dotiwalla, X., Eastham, P., Gipson, B., Higdon, D., Kucuktunc, O., Lieber, A., Lewis, B. L., Reynolds, Z., Vullikanti, A. K., Wang, L. & Marathe, M.
Nat. Commun. 12, 726 (2021).
Weiss, D. J., Nelson, A., Vargas-Ruiz, C. A., Gligorić, K., Bavadekar, S., Gabrilovich, E., Bertozzi-Villa, A., Rozier, J., Gibson, H. S., Shekel, T., Kamath, C., Lieber, A., Schulman, K., Shao, Y., Qarkaxhija, V., Nandi, A. K., Keddie, S. H., Rumisha, S., Amratia, P., Arambepola, R., Chestnutt, E. G., Millar, J. J., Symons, T. L., Cameron, E., Battle, K. E., Bhatt, S. & Gething, P. W.
Nat. Med. (2020).
Abueg, M., Hinch, R., Wu, N., Liu, L., Probert, W. J. M., Wu, A., Eastham, P., Shafi, Y., Rosencrantz, M., Dikovsky, M., Cheng, Z., Nurtay, A., Abeler-Dörner, L., Bonsall, D. G., McConnell, M. V., O’Banion, S. & Fraser, C.
medRxiv (2020). doi:10.1101/2020.08.29.20184135
Bavadekar, S., Dai, A., Davis, J., Desfontaines, D., Eckstein, I., Everett, K., Fabrikant, A., Flores, G., Gabrilovich, E., Gadepalli, K., Glass, S., Huang, R., Kamath, C., Kraft, D., Kumok, A., Marfatia, H., Mayer, Y., Miller, B., Pearce, A., Perera, I. M., Ramachandran, V., Raman, K., Roessler, T., Shafran, I., Shekel, T., Stanton, C., Stimes, J., Sun, M., Wellenius, G. & Zoghi, M.
arXiv [cs.CR] (2020).
Wellenius, G. A., Vispute, S., Espinosa, V., Fabrikant, A., Tsai, T. C., Hennessy, J., Williams, B., Gadepalli, K., Boulanger, A., Pearce, A., Kamath, C., Schlosberg, A., Bendebury, C., Stanton, C., Bavadekar, S., Pluntke, C., Desfontaines, D., Jacobson, B., Armstrong, Z., Gipson, B., Wilson, R., Widdowson, A., Chou, K., Oplinger, A., Shekel, T., Jha, A. K. & Gabrilovich, E.
arXiv [q-bio.PE] (2020).
Aktay, A., Bavadekar, S., Cossoul, G., Davis, J., Desfontaines, D., Fabrikant, A., Gabrilovich, E., Gadepalli, K., Gipson, B., Guevara, M., Kamath, C., Kansal, M., Lange, A., Mandayam, C., Oplinger, A., Pluntke, C., Roessler, T., Schlosberg, A., Shekel, T., Vispute, S., Vu, M., Wellenius, G., Williams, B. & Wilson, R. J.
Ruktanonchai, N. W., Floyd, J. R., Lai, S., Ruktanonchai, C. W., Sadilek, A., Rente-Lourenco, P., Ben, X., Carioli, A., Gwinn, J., Steele, J. E., Prosper, O., Schneider, A., Oplinger, A., Eastham, P. & Tatem, A. J.
Science 369, 1465–1470 (2020).
Sadilek, A., Hswen, Y., Bavadekar, S., Shekel, T., Brownstein, J. S. & Gabrilovich, E.
npj Digital Medicine 3, 1–12 (2020).
Bassolas, A., Barbosa-Filho, H., Dickinson, B., Dotiwalla, X., Eastham, P., Gallotti, R., Ghoshal, G., Gipson, B., Hazarie, S. A., Kautz, H., Kucuktunc, O., Lieber, A., Sadilek, A., & Ramasco, J. J.
Nat. Commun. 10, 4817 (2019).
Sadilek, A., Caty, S., DiPrete, L., Mansour, R., Schenk Jr., T., Bergtholdt, M., Jha, A., Ramaswami P., & Gabrilovich E.
npj Digital Med 1, 36 (2018).
by Angelica Willis and Akib Uddin
TensorFlow Blog | 20-Jun-2023
by Perry Nelson & Aisha Walcott-Bryant
Google Africa Blog | 1-Jun-2023
Google Keyword Blog | 28-Nov-2022
by Nicole Linton
Google Keyword Blog | 21-Oct-2022
by Akib Uddin & Andrew Sellergren
Google AI Blog | 19-Jul-2022
Google Japan Blog | 25-Nov-2021
by Zaid Nabulsi & Po-Hsuan Cameron Chen
Google AI Blog | 1-Sep-2021
by Rory Pilgrim & Shruthi Prabhakara
by Sunny Jansen & Krish Eswaran
Google Keyword Blog | 25-Feb-2021
by Cian Hughes
Google Keyword Blog | 29-Oct-2020
by Shravya Shetty & Daniel Tse
Google Keyword Blog | 1-Jan-2020
by Dave Steiner & Shravya Shetty
Google AI Blog | 3-Dec-2019
by Shravya Shetty
Google Keyword Blog | 20-May-2019
Lucido, J. J., DeWees, T. A., Leavitt, T. R., Anand, A., Beltran, C. J., Brooke, M. D., Buroker, J. R., Foote, R. L., Foss, O. R., Gleason, A. M., Hodge, T. L., Hughes, C. O., Hunzeker, A. E., Laack, N. N., Lenz, T. K., Livne, M., Morigami, M., Moseley, D. J., Undahl, L. M., Patel, Y., Tryggestad, E. J., Walker, M. Z., Zverovitch, A. & Patel, S. H.
Front. Oncol. 13, (2023).
Lee, C., Willis, A., Chen, C., Sieniek, M., Watters, A., Stetson, B., Uddin, A., Wong, J., Pilgrim, R., Chou, K., Tse, D., Shetty, S. & Gomes, R. G.
JAMA Netw Open 6, e2248685 (2023).
Gomes, R. G., Vwalika, B., Lee, C., Willis, A., Sieniek, M., Price, J. T., Chen, C., Kasaro, M. P., Taylor, J. A., Stringer, E. M., McKinney, S. M., Sindano, N., Dahl, G. E., Goodnight, W., Gilmer, J., Chi, B. H., Lau, C., Spitz, T., Saensuksopa, T., Liu, K., Tiyasirichokchai, T., Wong, J., Pilgrim, R., Uddin, A., Corrado, G., Peng, L., Chou, K., Tse, D., Stringer, J. S. A. & Shetty, S.
Communications Medicine 2, 1–9 (2022).
Kazemzadeh, S., Yu, J., Jamshy, S., Pilgrim, R., Nabulsi, Z., Chen, C., Beladia, N., Lau, C., McKinney, S. M., Hughes, T., Kiraly, A. P., Kalidindi, S. R., Muyoyeta, M., Malemela, J., Shih, T., Corrado, G. S., Peng, L., Chou, K., Chen, P.-H. C., Liu, Y., Eswaran, K., Tse, D., Shetty, S. & Prabhakara, S.
Radiology 212213 (2022).
Sellergren, A. B., Chen, C., Nabulsi, Z., Li, Y., Maschinot, A., Sarna, A., Huang, J., Lau, C., Kalidindi, S. R., Etemadi, M., Garcia-Vicente, F., Melnick, D., Liu, Y., Eswaran, K., Tse, D., Beladia, N., Krishnan, D. & Shetty, S.
Radiology 212482 (2022).
Anand, A., Beltran, C. J., Brooke, M. D., Buroker, J. R., DeWees, T. A., Foote, R. L., Foss, O. R., Hughes, C. O., Hunzeker, A. E., John Lucido, J., Morigami, M., Moseley, D. J., Pafundi, D. H., Patel, S. H., Patel, Y., Ridgway, A. K., Tryggestad, E. J., Wilson, M. Z., Xi, L. & Zverovitch, A.
medRxiv 2021.12.07.21266421 (2021).
Nabulsi, Z., Sellergren, A., Jamshy, S., Lau, C., Santos, E., Kiraly, A. P., Ye, W., Yang, J., Pilgrim, R., Kazemzadeh, S., Yu, J., Kalidindi, S. R., Etemadi, M., Garcia-Vicente, F., Melnick, D., Corrado, G. S., Peng, L., Eswaran, K., Tse, D., Beladia, N., Liu, Y., Chen, P.-H. C. & Shetty, S.
Sci. Rep. 11, 1–15 (2021).
Nikolov, S., Blackwell, S., Zverovitch, A., Mendes, R., Livne, M., De Fauw, J., Patel, Y., Meyer, C., Askham, H., Romera-Paredes, B., Kelly, C., Karthikesalingam, A., Chu, C., Carnell, D., Boon, C., D’Souza, D., Moinuddin, S. A., Garie, B., McQuinlan, Y., Ireland, S., Hampton, K., Fuller, K., Montgomery, H., Rees, G., Suleyman, M., Back, T., Hughes, C. O., Ledsam, J. R. & Ronneberger, O.
J. Med. Internet Res. 23, e26151 (2021).
Duggan, G. E., Reicher, J. J., Liu, Y., Tse, D. & Shetty, S.
Br J Radiol. 94, 20210435 (2021).
McKinney, S. M., Sieniek, M., Godbole, V., Godwin, J., Antropova, N., Ashrafian, H., Back, T., Chesus, M., Corrado, G. S., Darzi, A., Etemadi, M., Garcia-Vicente, F., Gilbert, F. J., Halling-Brown, M., Hassabis, D., Jansen, S., Karthikesalingam, A., Kelly, C. J., King, D., Ledsam, J. R., Melnick, D., Mostofi, H., Peng, L., Reicher, J. J., Romera-Paredes, B., Sidebottom, R., Suleyman, M., Tse, D., Young, K. C., De Fauw, J. & Shetty, S.
Nature 577, 89–94 (2020). [readcube]
Majkowska, A., Mittal, S., Steiner, D. F., Reicher, J. J., McKinney, S. M., Duggan, G. E., Eswaran, K., Cameron Chen, P.-H., Liu, Y., Kalidindi, S. R., Ding, A., Corrado, G. S., Tse, D. & Shetty, S.
Radiology 191293 (2019).
Ardila, D., Kiraly, A. P., Bharadwaj, S., Choi, B., Reciher, J. J., Peng, L., Tse, D., Etemadi, M., Ye, W., Corrado, G., Naidich, D. P., Shetty, S.
Nat. Med. 25, 954–961 (2019). [readcube]
Blog Posts [more at Youtube Official Blog ]
Youtube Official Blog
15-Aug-2023
12-Jun-2023
15-May-2023
by Garth Graham
Youtube Official Blog | 18-Apr-2023
by Jessica DiVento Dzuban
Youtube Official Blog | 7-Oct-2022
Youtube Official Blog | 1-Mar-2023
Youtube Official Blog | 27-Oct-2022
Youtube Official Blog | 28–Sep-2022
Youtube Official Blog | 12–Sep-2022
Youtube Official Blog | 15-Jun-2022
Youtube Official Blog | 24-Mar-2022
Youtube Official Blog | 26-Jan-2022
Youtube Official Blog | 13-Oct-2021
Youtube Official Blog | 19-Jul-2021
Thank you for visiting nature.com. You are using a browser version with limited support for CSS. To obtain the best experience, we recommend you use a more up to date browser (or turn off compatibility mode in Internet Explorer). In the meantime, to ensure continued support, we are displaying the site without styles and JavaScript.
- View all journals
- Explore content
- About the journal
- Publish with us
- Sign up for alerts
- Review Article
- Published: 20 January 2022
AI in health and medicine
- Pranav Rajpurkar ORCID: orcid.org/0000-0002-8030-3727 1 na1 ,
- Emma Chen 2 na1 ,
- Oishi Banerjee 2 na1 &
- Eric J. Topol ORCID: orcid.org/0000-0002-1478-4729 3
Nature Medicine volume 28 , pages 31–38 ( 2022 ) Cite this article
131k Accesses
563 Citations
619 Altmetric
Metrics details
- Computational biology and bioinformatics
- Medical research
Artificial intelligence (AI) is poised to broadly reshape medicine, potentially improving the experiences of both clinicians and patients. We discuss key findings from a 2-year weekly effort to track and share key developments in medical AI. We cover prospective studies and advances in medical image analysis, which have reduced the gap between research and deployment. We also address several promising avenues for novel medical AI research, including non-image data sources, unconventional problem formulations and human–AI collaboration. Finally, we consider serious technical and ethical challenges in issues spanning from data scarcity to racial bias. As these challenges are addressed, AI’s potential may be realized, making healthcare more accurate, efficient and accessible for patients worldwide.
This is a preview of subscription content, access via your institution
Access options
Access Nature and 54 other Nature Portfolio journals
Get Nature+, our best-value online-access subscription
24,99 € / 30 days
cancel any time
Subscribe to this journal
Receive 12 print issues and online access
195,33 € per year
only 16,28 € per issue
Rent or buy this article
Prices vary by article type
Prices may be subject to local taxes which are calculated during checkout
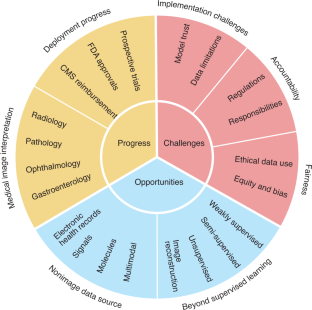
Similar content being viewed by others
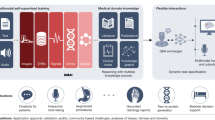
Foundation models for generalist medical artificial intelligence
Michael Moor, Oishi Banerjee, … Pranav Rajpurkar
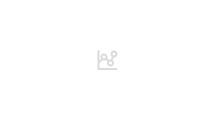
Guiding principles for the responsible development of artificial intelligence tools for healthcare
Kimberly Badal, Carmen M. Lee & Laura J. Esserman
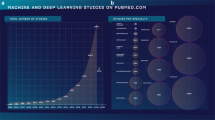
A short guide for medical professionals in the era of artificial intelligence
Bertalan Meskó & Marton Görög
Gulshan, V. et al. Development and validation of a deep learning algorithm for detection of diabetic retinopathy in retinal fundus photographs. J. Am. Med. Assoc. 316 , 2402–2410 (2016).
Article Google Scholar
Esteva, A. et al. Dermatologist-level classification of skin cancer with deep neural networks. Nature 542 , 115–118 (2017).
Article CAS PubMed PubMed Central Google Scholar
Rajpurkar, P. et al. Deep learning for chest radiograph diagnosis: a retrospective comparison of the CheXNeXt algorithm to practicing radiologists. PLoS Med. 15 , e1002686 (2018).
Article PubMed PubMed Central Google Scholar
Hannun, A. Y. et al. Cardiologist-level arrhythmia detection and classification in ambulatory electrocardiograms using a deep neural network. Nat. Med. 25 , 65–69 (2019).
Wiens, J. et al. Do no harm: a roadmap for responsible machine learning for health care. Nat. Med. 25 , 1337–1340 (2019).
Article CAS PubMed Google Scholar
Kanagasingam, Y. et al. Evaluation of artificial intelligence-based grading of diabetic retinopathy in primary care. JAMA Netw. Open 1 , e182665 (2018).
Beede, E. et al. A human-centered evaluation of a deep learning system deployed in clinics for the detection of diabetic retinopathy. in Proceedings of the 2020 CHI Conference on Human Factors in Computing Systems 1–12 (Association for Computing Machinery, 2020); https://dl.acm.org/doi/abs/10.1145/3313831.3376718
Kiani, A. et al. Impact of a deep learning assistant on the histopathologic classification of liver cancer. NPJ Digit. Med. 3 , 23 (2020).
Lin, H. et al. Diagnostic efficacy and therapeutic decision-making capacity of an artificial intelligence platform for childhood cataracts in eye clinics: a multicentre randomized controlled trial. EClinicalMedicine 9 , 52–59 (2019).
Gong, D. et al. Detection of colorectal adenomas with a real-time computer-aided system (ENDOANGEL): a randomised controlled study. Lancet Gastroenterol. Hepatol. 5 , 352–361 (2020).
Article PubMed Google Scholar
Wang, P. et al. Effect of a deep-learning computer-aided detection system on adenoma detection during colonoscopy (CADe-DB trial): a double-blind randomised study. Lancet Gastroenterol. Hepatol. 5 , 343–351 (2020).
Hollon, T. C. et al. Near real-time intraoperative brain tumor diagnosis using stimulated Raman histology and deep neural networks. Nat. Med. 26 , 52–58 (2020).
Phillips, M. et al. Assessment of accuracy of an artificial intelligence algorithm to detect melanoma in images of skin lesions. JAMA Netw. Open 2 , e1913436 (2019).
Nimri, R. et al. Insulin dose optimization using an automated artificial intelligence-based decision support system in youths with type 1 diabetes. Nat. Med. 26 , 1380–1384 (2020).
Wijnberge, M. et al. Effect of a machine learning-derived early warning system for intraoperative hypotension vs. standard care on depth and duration of intraoperative hypotension during elective noncardiac surgery. J. Am. Med. Assoc. 323 , 1052–1060 (2020).
Wismüller, A. & Stockmaster, L. A prospective randomized clinical trial for measuring radiology study reporting time on Artificial Intelligence-based detection of intracranial hemorrhage in emergent care head CT. in Medical Imaging 2020: Biomedical Applications in Molecular, Structural, and Functional Imaging vol. 11317, 113170M (International Society for Optics and Photonics, 2020).
Liu, X. et al. Reporting guidelines for clinical trial reports for interventions involving artificial intelligence: the CONSORT-AI extension. Br. Med. J. 370 , m3164 (2020).
Rivera, S. C. et al. Guidelines for clinical trial protocols for interventions involving artificial intelligence: the SPIRIT-AI extension. Nat. Med. 26 , 1351–1363 (2020).
Centers for Medicare & Medicaid Services. Medicare Program; Hospital Inpatient Prospective Payment Systems for Acute Care Hospitals and the Long-Term Care Hospital Prospective Payment System and Final Policy Changes and Fiscal Year 2021 Rates; Quality Reporting and Medicare and Medicaid Promoting Interoperability Programs Requirements for Eligible Hospitals and Critical Access Hospitals. Fed. Regist. 85 , 58432–59107 (2020).
Benjamens, S., Dhunnoo, P. & Meskó, B. The state of artificial intelligence-based FDA-approved medical devices and algorithms: an online database. NPJ Digit. Med. 3 , 118 (2020).
Wu, N. et al. Deep neural networks improve radiologists’ performance in breast cancer screening. IEEE Trans. Med. Imaging 39 , 1184–1194 (2020).
McKinney, S. M. et al. International evaluation of an AI system for breast cancer screening. Nature 577 , 89–94 (2020).
Ghorbani, A. et al. Deep learning interpretation of echocardiograms. NPJ Digit. Med. 3 , 10 (2020).
Ouyang, D. et al. Video-based AI for beat-to-beat assessment of cardiac function. Nature 580 , 252–256 (2020).
Ardila, D. et al. End-to-end lung cancer screening with three-dimensional deep learning on low-dose chest computed tomography. Nat. Med. 25 , 954–961 (2019).
Huynh, E. et al. Artificial intelligence in radiation oncology. Nat. Rev. Clin. Oncol. 17 , 771–781 (2020).
Huang, P. et al. Prediction of lung cancer risk at follow-up screening with low-dose CT: a training and validation study of a deep learning method. Lancet Digit. Health 1 , e353–e362 (2019).
Kather, J. N. et al. Deep learning can predict microsatellite instability directly from histology in gastrointestinal cancer. Nat. Med. 25 , 1054–1056 (2019).
Jackson, H. W. et al. The single-cell pathology landscape of breast cancer. Nature 578 , 615–620 (2020).
Campanella, G. et al. Clinical-grade computational pathology using weakly supervised deep learning on whole slide images. Nat. Med. 25 , 1301–1309 (2019).
Fu, Y. et al. Pan-cancer computational histopathology reveals mutations, tumor composition and prognosis. Nat. Cancer 1 , 800–810 (2020).
Courtiol, P. et al. Deep learning-based classification of mesothelioma improves prediction of patient outcome. Nat. Med. 25 , 1519–1525 (2019).
Bera, K., Schalper, K. A., Rimm, D. L., Velcheti, V. & Madabhushi, A. Artificial intelligence in digital pathology: new tools for diagnosis and precision oncology. Nat. Rev. Clin. Oncol. 16 , 703–715 (2019).
Zhou, D. et al. Diagnostic evaluation of a deep learning model for optical diagnosis of colorectal cancer. Nat. Commun. 11 , 2961 (2020).
Zhao, S. et al. Magnitude, risk factors, and factors associated with adenoma miss rate of tandem colonoscopy: a systematic review and meta-analysis. Gastroenterology 156 , 1661–1674 (2019).
Freedman, D. et al. Detecting deficient coverage in colonoscopies. IEEE Trans. Med. Imaging 39 , 3451–3462 (2020).
Liu, H. et al. Development and validation of a deep learning system to detect glaucomatous optic neuropathy using fundus photographs. JAMA Ophthalmol. 137 , 1353–1360 (2019).
Milea, D. et al. Artificial intelligence to detect papilledema from ocular fundus photographs. N. Engl. J. Med. 382 , 1687–1695 (2020).
Wolf, R. M., Channa, R., Abramoff, M. D. & Lehmann, H. P. Cost-effectiveness of autonomous point-of-care diabetic retinopathy screening for pediatric patients with diabetes. JAMA Ophthalmol. 138 , 1063–1069 (2020).
Xie, Y. et al. Artificial intelligence for teleophthalmology-based diabetic retinopathy screening in a national programme: an economic analysis modelling study. Lancet Digit. Health 2 , e240–e249 (2020).
Arcadu, F. et al. Deep learning algorithm predicts diabetic retinopathy progression in individual patients. NPJ Digit. Med. 2 , 92 (2019).
Senior, A. W. et al. Improved protein structure prediction using potentials from deep learning. Nature 577 , 706–710 (2020).
Alley, E. C., Khimulya, G., Biswas, S., AlQuraishi, M. & Church, G. M. Unified rational protein engineering with sequence-based deep representation learning. Nat. Methods 16 , 1315–1322 (2019).
Gainza, P. et al. Deciphering interaction fingerprints from protein molecular surfaces using geometric deep learning. Nat. Methods 17 , 184–192 (2020).
Greener, J.G. et al. Deep learning extends de novo protein modelling coverage of genomes using iteratively predicted structural constraints. Nat. Commun. 10 , 3977 (2019).
Chabon, J. J. et al. Integrating genomic features for non-invasive early lung cancer detection. Nature 580 , 245–251 (2020).
Luo, H. et al. Circulating tumor DNA methylation profiles enable early diagnosis, prognosis prediction, and screening for colorectal cancer. Sci. Transl. Med. 12 , eaax7533 (2020).
Cristiano, S. et al. Genome-wide cell-free DNA fragmentation in patients with cancer. Nature 570 , 385–389 (2019).
Gussow, A. B. et al. Machine-learning approach expands the repertoire of anti-CRISPR protein families. Nat. Commun. 11 , 3784 (2020).
Wang, D. et al. Optimized CRISPR guide RNA design for two high-fidelity Cas9 variants by deep learning. Nat. Commun. 10 , 4284 (2019).
Bhattacharyya, R. P. et al. Simultaneous detection of genotype and phenotype enables rapid and accurate antibiotic susceptibility determination. Nat. Med. 25 , 1858–1864 (2019).
Stokes, J. M. et al. A deep learning approach to antibiotic discovery. Cell 181 , 475–483 (2020).
Zhavoronkov, A. et al. Deep learning enables rapid identification of potent DDR1 kinase inhibitors. Nat. Biotechnol. 37 , 1038–1040 (2019).
Lee, J. et al. BioBERT: a pre-trained biomedical language representation model for biomedical text mining. Bioinformatics 36 , 1234–1240 (2020).
CAS PubMed Google Scholar
Zhu, Y., Li, L., Lu, H., Zhou, A. & Qin, X. Extracting drug-drug interactions from texts with BioBERT and multiple entity-aware attentions. J. Biomed. Inform. 106 , 103451 (2020).
Smit, A. et al. CheXbert: Combining automatic labelers and expert annotations for accurate radiology report labeling using BERT. in Proceedings of the 2020 Conference on Empirical Methods in Natural Language Processing 1500–1519 (2020).
Sarker, A., Gonzalez-Hernandez, G., Ruan, Y. & Perrone, J. Machine learning and natural language processing for geolocation-centric monitoring and characterization of opioid-related social media chatter. JAMA Netw. Open 2 , e1914672 (2019).
Claassen, J. et al. Detection of brain activation in unresponsive patients with acute brain injury. N. Engl. J. Med. 380 , 2497–2505 (2019).
Porumb, M., Stranges, S., Pescapè, A. & Pecchia, L. Precision medicine and artificial intelligence: a pilot study on deep learning for hypoglycemic events detection based on ECG. Sci. Rep. 10 , 170 (2020).
Attia, Z. I. et al. An artificial intelligence-enabled ECG algorithm for the identification of patients with atrial fibrillation during sinus rhythm: a retrospective analysis of outcome prediction. Lancet 394 , 861–867 (2019).
Chan, J., Raju, S., Nandakumar, R., Bly, R. & Gollakota, S. Detecting middle ear fluid using smartphones. Sci. Transl. Med. 11 , eaav1102 (2019).
Willett, F. R., Avansino, D. T., Hochberg, L. R., Henderson, J. M. & Shenoy, K. V. High-performance brain-to-text communication via handwriting. Nature 593 , 249–254 (2021).
Green, E. M. et al. Machine learning detection of obstructive hypertrophic cardiomyopathy using a wearable biosensor. NPJ Digit. Med. 2 , 57 (2019).
Thorsen-Meyer, H.-C. et al. Dynamic and explainable machine learning prediction of mortality in patients in the intensive care unit: a retrospective study of high-frequency data in electronic patient records. Lancet Digit. Health 2 , e179–e191 (2020).
Porter, P. et al. A prospective multicentre study testing the diagnostic accuracy of an automated cough sound centred analytic system for the identification of common respiratory disorders in children. Respir. Res. 20 , 81 (2019).
Tomašev, N. et al. A clinically applicable approach to continuous prediction of future acute kidney injury. Nature 572 , 116–119 (2019).
Kehl, K. L. et al. Assessment of deep natural language processing in ascertaining oncologic outcomes from radiology reports. JAMA Oncol. 5 , 1421–1429 (2019).
Huang, S.-C., Pareek, A., Seyyedi, S., Banerjee, I. & Lungren, M. P. Fusion of medical imaging and electronic health records using deep learning: a systematic review and implementation guidelines. NPJ Digit. Med. 3 , 136 (2020).
Wang, C. et al. Quantitating the epigenetic transformation contributing to cholesterol homeostasis using Gaussian process. Nat. Commun. 10 , 5052 (2019).
Li, Y. et al. Inferring multimodal latent topics from electronic health records. Nat. Commun. 11 , 2536 (2020).
Tshitoyan, V. et al. Unsupervised word embeddings capture latent knowledge from materials science literature. Nature 571 , 95–98 (2019).
Li, X. et al. Deep learning enables accurate clustering with batch effect removal in single-cell RNA-seq analysis. Nat. Commun. 11 , 2338 (2020).
Amodio, M. et al. Exploring single-cell data with deep multitasking neural networks. Nat. Methods 16 , 1139–1145 (2019).
Urteaga, I., McKillop, M. & Elhadad, N. Learning endometriosis phenotypes from patient-generated data. NPJ Digit. Med. 3 , 88 (2020).
Brbić, M. et al. MARS: discovering novel cell types across heterogeneous single-cell experiments. Nat. Methods 17 , 1200–1206 (2020).
Seymour, C. W. et al. Derivation, validation, and potential treatment implications of novel clinical phenotypes for sepsis. J. Am. Med. Assoc. 321 , 2003–2017 (2019).
Article CAS Google Scholar
Fries, J. A. et al. Weakly supervised classification of aortic valve malformations using unlabeled cardiac MRI sequences. Nat. Commun. 10 , 3111 (2019).
Jin, L. et al. Deep learning enables structured illumination microscopy with low light levels and enhanced speed. Nat. Commun. 11 , 1934 (2020).
Vishnevskiy, V. et al. Deep variational network for rapid 4D flow MRI reconstruction. Nat. Mach. Intell. 2 , 228–235 (2020).
Masutani, E. M., Bahrami, N. & Hsiao, A. Deep learning single-frame and multiframe super-resolution for cardiac MRI. Radiology 295 , 552–561 (2020).
Rana, A. et al. Use of deep learning to develop and analyze computational hematoxylin and eosin staining of prostate core biopsy images for tumor diagnosis. JAMA Netw. Open 3 , e205111 (2020).
Liu, X. et al. A comparison of deep learning performance against health-care professionals in detecting diseases from medical imaging: a systematic review and meta-analysis. Lancet Digit. Health 1 , e271–e297 (2019).
Chen, P.-H. C. et al. An augmented reality microscope with real-time artificial intelligence integration for cancer diagnosis. Nat. Med. 25 , 1453–1457 (2019).
Patel, B. N. et al. Human–machine partnership with artificial intelligence for chest radiograph diagnosis. NPJ Digit. Med. 2 , 111 (2019).
Sim, Y. et al. Deep convolutional neural network–based software improves radiologist detection of malignant lung nodules on chest radiographs. Radiology 294 , 199–209 (2020).
Park, A. et al. Deep learning–assisted diagnosis of cerebral aneurysms using the HeadXNet model. JAMA Netw. Open 2 , e195600 (2019).
Steiner, D. F. et al. Impact of deep learning assistance on the histopathologic review of lymph nodes for metastatic breast cancer. Am. J. Surg. Pathol. 42 , 1636–1646 (2018).
Jain, A. et al. Development and assessment of an artificial intelligence-based tool for skin condition diagnosis by primary care physicians and nurse practitioners in teledermatology practices. JAMA Netw. Open 4 , e217249 (2021).
Seah, J. C. Y. et al. Effect of a comprehensive deep-learning model on the accuracy of chest x-ray interpretation by radiologists: a retrospective, multireader multicase study. Lancet Digit. Health 3 , e496–e506 (2021).
Rajpurkar, P. et al. CheXaid: deep learning assistance for physician diagnosis of tuberculosis using chest x-rays in patients with HIV. NPJ Digit. Med. 3 , 115 (2020).
Kim, H.-E. et al. Changes in cancer detection and false-positive recall in mammography using artificial intelligence: a retrospective, multireader study. Lancet Digit. Health 2 , e138–e148 (2020).
Tschandl, P. et al. Human–computer collaboration for skin cancer recognition. Nat. Med. 26 , 1229–1234 (2020).
van der Laak, J., Litjens, G. & Ciompi, F. Deep learning in histopathology: the path to the clinic. Nat. Med. 27 , 775–784 (2021).
Willemink, M. J. et al. Preparing medical imaging data for machine learning. Radiology 295 , 4–15 (2020).
Irvin, J. et al. CheXpert: a large chest radiograph dataset with uncertainty labels and expert comparison. in Proceedings of the AAAI Conference on Artificial Intelligence vol. 33, 590–597 (2019).
Kelly, C. J., Karthikesalingam, A., Suleyman, M., Corrado, G. & King, D. Key challenges for delivering clinical impact with artificial intelligence. BMC Med. 17 , 195 (2019).
DeGrave, A. J., Janizek, J. D. & Lee, S.-I. AI for radiographic COVID-19 detection selects shortcuts over signal. Nat. Mach. Intell. 3 , 610–619 (2021).
Cutillo, C. M. et al. Machine intelligence in healthcare: perspectives on trustworthiness, explainability, usability, and transparency. NPJ Digit. Med. 3 , 47 (2020).
Sendak, M. P., Gao, M., Brajer, N. & Balu, S. Presenting machine learning model information to clinical end users with model facts labels. NPJ Digit. Med. 3 , 41 (2020).
Saporta, A. et al. Deep learning saliency maps do not accurately highlight diagnostically relevant regions for medical image interpretation. Preprint at medRxiv https://doi.org/10.1101/2021.02.28.21252634 (2021).
Ehsan, U. et al . The who in explainable AI: how AI background shapes perceptions of AI explanations. Preprint at https://arxiv.org/abs/2107.13509 (2021).
Reyes, M. et al. On the interpretability of artificial intelligence in radiology: Challenges and opportunities. Radio. Artif. Intell. 2 , e190043 (2020).
Liu, C. et al . On the replicability and reproducibility of deep learning in software engineering. Preprint at https://arxiv.org/abs/2006.14244 (2020).
Beam, A. L., Manrai, A. K. & Ghassemi, M. Challenges to the reproducibility of machine learning models in health care. J. Am. Med. Assoc. 323 , 305–306 (2020).
Gerke, S., Babic, B., Evgeniou, T. & Cohen, I. G. The need for a system view to regulate artificial intelligence/machine learning-based software as medical device. NPJ Digit. Med. 3 , 53 (2020).
Lee, C. S. & Lee, A. Y. Clinical applications of continual learning machine learning. Lancet Digit. Health 2 , e279–e281 (2020).
Food and Drug Administration. Proposed Regulatory Framework for Modifications to Artificial Intelligence/Machine Learning (AI/ML)-Based Software as a Medical Device (SaMD): Discussion Paper and Request for Feedback (FDA, 2019).
Morley, J. et al. The debate on the ethics of AI in health care: a reconstruction and critical review. SSRN http://dx.doi.org/10.2139/ssrn.3486518 (2019.
Price, W. N., Gerke, S. & Cohen, I. G. Potential liability for physicians using artificial intelligence. J. Am. Med. Assoc. 322 , 1765–1766 (2019).
Larson, D. B., Magnus, D. C., Lungren, M. P., Shah, N. H. & Langlotz, C. P. Ethics of using and sharing clinical imaging data for artificial intelligence: a proposed framework. Radiology 295 , 675–682 (2020).
Kaissis, G. A., Makowski, M. R., Rückert, D. & Braren, R. F. Secure, privacy-preserving and federated machine learning in medical imaging. Nat. Mach. Intell. 2 , 305–311 (2020).
Larrazabal, A. J., Nieto, N., Peterson, V., Milone, D. H. & Ferrante, E. Gender imbalance in medical imaging datasets produces biased classifiers for computer-aided diagnosis. Proc. Natl Acad. Sci. USA 117 , 12592–12594 (2020).
Vyas, D. A., Eisenstein, L. G. & Jones, D. S. Hidden in plain sight: reconsidering the use of race correction in clinical algorithms. N. Engl. J. Med. 383 , 874–882 (2020).
Obermeyer, Z., Powers, B., Vogeli, C. & Mullainathan, S. Dissecting racial bias in an algorithm used to manage the health of populations. Science 366 , 447–453 (2019).
Cirillo, D. et al. Sex and gender differences and biases in artificial intelligence for biomedicine and healthcare. NPJ Digit. Med. 3 , 81 (2020).
Download references
Acknowledgements
We thank A. Tamkin and N. Phillips for their feedback. E.J.T. receives funding support from US National Institutes of Health grant UL1TR002550.
Author information
These authors contributed equally: Pranav Rajpurkar, Emma Chen, Oishi Banerjee.
Authors and Affiliations
Department of Biomedical Informatics, Harvard University, Cambridge, MA, USA
Pranav Rajpurkar
Department of Computer Science, Stanford University, Stanford, CA, USA
Emma Chen & Oishi Banerjee
Scripps Translational Science Institute, San Diego, CA, USA
Eric J. Topol
You can also search for this author in PubMed Google Scholar
Contributions
P.R. and E.J.T. conceptualized this Review. E.C., O.B. and P.R. were responsible for the design and synthesis of this Review. All authors contributed to writing and editing the manuscript.
Corresponding author
Correspondence to Eric J. Topol .
Ethics declarations
Competing interests.
The authors declare no competing interests.
Peer review
Peer review information.
Nature Medicine thanks Despina Kontos and the other, anonymous, reviewer(s) for their contribution to the peer review of this work. Karen O’Leary was the primary editor on this article and managed its editorial process and peer review in collaboration with the rest of the editorial team.
Additional information
Publisher’s note Springer Nature remains neutral with regard to jurisdictional claims in published maps and institutional affiliations.
Rights and permissions
Reprints and permissions
About this article
Cite this article.
Rajpurkar, P., Chen, E., Banerjee, O. et al. AI in health and medicine. Nat Med 28 , 31–38 (2022). https://doi.org/10.1038/s41591-021-01614-0
Download citation
Received : 23 July 2021
Accepted : 05 November 2021
Published : 20 January 2022
Issue Date : January 2022
DOI : https://doi.org/10.1038/s41591-021-01614-0
Share this article
Anyone you share the following link with will be able to read this content:
Sorry, a shareable link is not currently available for this article.
Provided by the Springer Nature SharedIt content-sharing initiative
This article is cited by
Development and validation of a machine learning model to predict time to renal replacement therapy in patients with chronic kidney disease.
- Takeshi Nakata
- Hirotaka Shibata
BMC Nephrology (2024)
Individualized estimation of arterial carbon dioxide partial pressure using machine learning in children receiving mechanical ventilation
- Bongjin Lee
- June Dong Park
BMC Pediatrics (2024)
“That’s just Future Medicine” - a qualitative study on users’ experiences of symptom checker apps
- Regina Müller
- Malte Klemmt
- Robert Ranisch
BMC Medical Ethics (2024)
Machine learning in physical activity, sedentary, and sleep behavior research
- Vahid Farrahi
- Mehrdad Rostami
Journal of Activity, Sedentary and Sleep Behaviors (2024)
Physician–machine partnerships boost diagnostic accuracy, but bias persists
Nature Medicine (2024)
Quick links
- Explore articles by subject
- Guide to authors
- Editorial policies
Sign up for the Nature Briefing newsletter — what matters in science, free to your inbox daily.

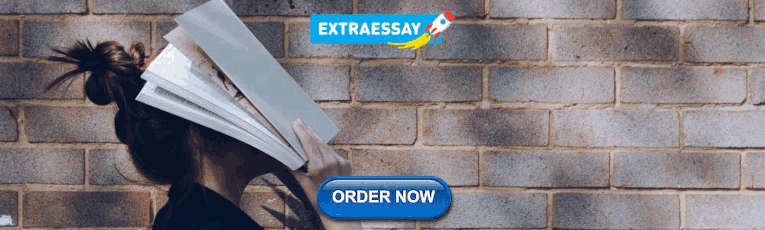
IMAGES
COMMENTS
Mar 20, 2024. Case Records of the Massachusetts General Hospital. Mar 15, 2024. The New England Journal of Medicine (NEJM) is a weekly general medical journal that publishes new medical research ...
PubMed is a comprehensive database of biomedical literature from various sources, including MEDLINE, life science journals, and online books. You can search for citations, access full text content, and explore topics related to health, medicine, and biology. PubMed also provides advanced search options and tools for researchers and clinicians.
Original Article Mar 13, 2024 Brief Report: Intraventricular CARv3-TEAM-E T Cells in Recurrent Glioblastoma. B.D. Choi and Others DOI: 10.1056/NEJMoa2314390. Perspective Mar 6, 2024 Back to the ...
To examine the knowledge, attitudes, and practices (KAP) of caregivers of children with Kawasaki disease toward Kawasaki disease. Miaomiao Zhao, Jiaxin Ye, Luping Chen, Yitong Yang, Meng Zhao, Mingzhu Yang and Zhaoling Shi. BMC Public Health 2024 24 :899. Research Published on: 26 March 2024. Full Text.
Co-occurrence analysis assumes that the authors’ keywords of a paper may be considered a synthetic descriptor of the content but also a reference for detecting linkages among issues analysed. 51 Therefore, the co-occurrence around the same word or pair of words may point out a research subject or trend in a specific field. 14 The tool allows ...
Artificial intelligence (AI) is a powerful and disruptive area of computer science, with the potential to fundamentally transform the practice of medicine and the delivery of healthcare. In this review article, we outline recent breakthroughs in the application of AI in healthcare, describe a roadmap to building effective, reliable and safe AI ...
Explore this issue of The New England Journal of Medicine (Vol. 0 No. 0).
Original research studies that can improve decision making in clinical medicine, public health, health care policy, medical education, or biomedical research. Intended for healthcare professionals Our Company Subscribe My Account Login
The Lancet Digital Health (2022). Publications. Redesigning Clinical Pathways for Immediate Diabetic Retinopathy Screening Results. Pedersen Elin Rønby, Cuadros Jorge, Khan Mahbuba, Fleischmann Sybille, Wolff Gregory, Hammel Naama, Liu Yun & Leung Geoffrey.
A comparison of deep learning performance against health-care professionals in detecting diseases from medical imaging: a systematic review and meta-analysis. Lancet Digit. Health 1, e271–e297 ...